
For decades, quality control meant sampling—testing 5% here, 10% there, and using those results to make general assumptions about a lot. To say it was a blunt tool would be an understatement. But AI is changing that. It’s not about sampling anymore; it’s about real-time quality assurance at a level of detail and scale we’ve never seen before. We’re talking 100% inspection, every single time. The same promise the industrial revolutions offered: better, faster, more reliable—only now, it’s at a microscopic level.

So, why is AI-powered quality control the next frontier in manufacturing? It isn’t about technology for technology's sake. It’s about how AI aligns with the very soul of manufacturing—an uncompromising pursuit of excellence.
Quality Control Yesterday vs. Today: An Evolution of Tools
Manufacturing is no stranger to innovation. We've gone from manual craftsmanship to assembly lines, to full-scale automation. But quality control has always had a catch-up problem.
In the past, the quality control process hinged on statistical sampling and human judgment. Picture a quality inspector meticulously checking products on the line, marking defects, deciding which items get tossed aside. The system was workable. It got us here. But it was also deeply flawed. Subjective. Prone to error. And simply too slow for today’s hyper-efficient factories. Human inspectors can only be so consistent. Their eyes can only catch so much, and fatigue is inevitable. That’s where AI steps in—not to replace human inspectors but to give them superhuman support.
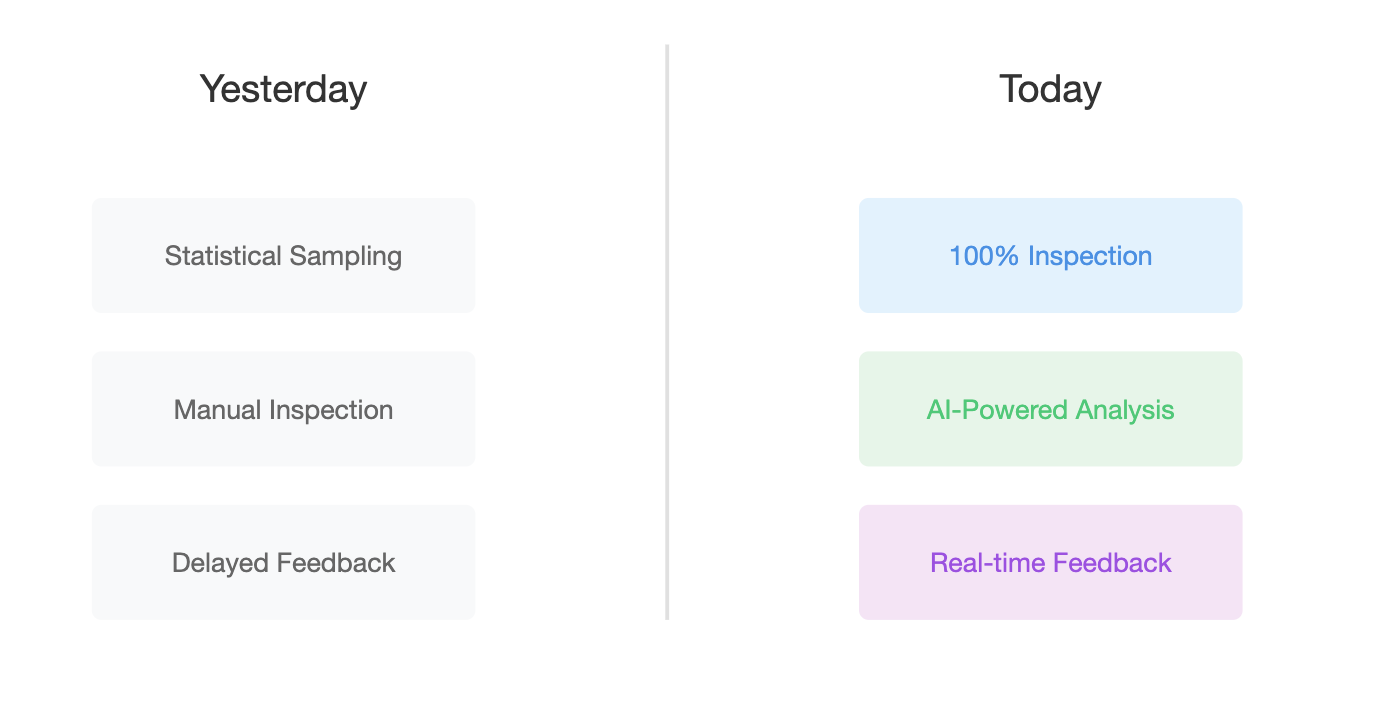
Now, imagine an AI-powered vision system installed along a production line. Cameras capture every angle, every detail of the product in real-time. AI algorithms, trained on massive datasets, compare every item to the ideal model. It’s not just looking for defects, it’s learning—adapting to new production conditions and updating its parameters autonomously. And it’s doing this thousands of times per second.
The outcome? Defects detected and corrected almost instantly. Downtime minimized. Scrap rates reduced. And most importantly, the quality of the final product reaching customers is virtually assured, not assumed.
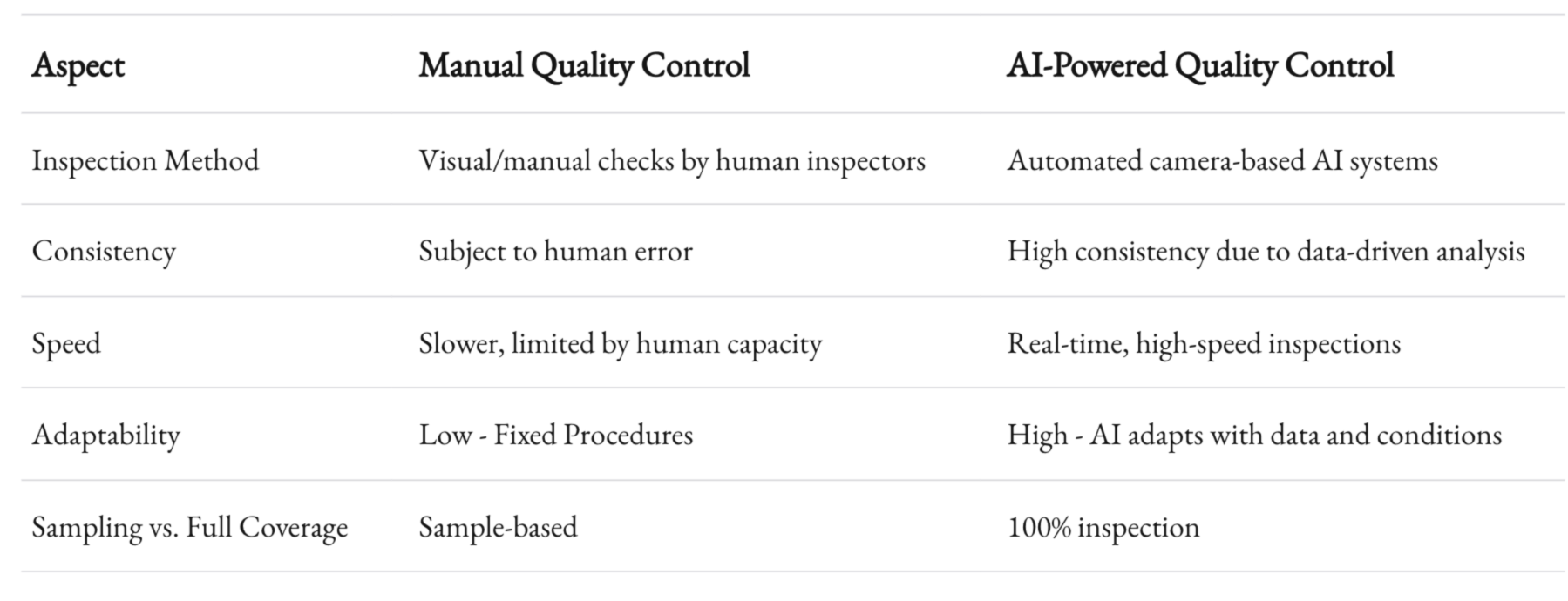
The True Potential of AI in Manufacturing Quality
A factory’s value proposition is not only about volume but also about precision. AI delivers exactly this, but what truly stands out is the nuanced capability to spot patterns no human ever could.

Take, for example, an automotive manufacturer that relies heavily on welding. In the old days, quality meant inspecting the welds visually or by using X-rays—sampling a handful of welds out of thousands made every day. Now, AI can “hear” and “see” the welding process in real time. Acoustic sensors analyze the sound of the welding arc, and high-speed cameras capture every flash and fizzle. AI models learn the acoustic signature of a perfect weld and flag anomalies on the spot. Operators are alerted, adjustments made—in a matter of seconds. That’s proactive quality control, reducing rework and waste.

A similar leap forward can be seen in pharmaceutical manufacturing. Production must be highly regulated, precise, and adhere to rigorous quality standards. AI-powered cameras, tuned to detect micro-level anomalies in packaging or pill formation, catch deviations that could otherwise lead to contamination or underdosing—factors which could have disastrous consequences.
It's not just defect detection. AI-driven quality control systems can also predict equipment failure. By combining computer vision data with sensor readings like vibration or temperature, AI can tell when a motor is straining before it breaks down. This foresight turns reactive maintenance into planned actions, slashing the risk of unexpected downtime.
Some Real-world Success Stories
We can theorize all day, but what does it look like in practice? The story of Siemens’ Amberg Electronics Plant in Germany is a good place to start. The facility is often cited as a model of what a “smart factory” can achieve. Their adoption of AI-driven quality control has led to near-zero defects. Machines learn from human workers and vice versa, in a process Siemens calls “human-in-the-loop.” It’s a symbiotic relationship where AI picks up what humans might miss and vice versa—resulting in production that’s 99.99885% defect-free. It’s astonishing.
Another example is Fanuc, the Japanese robotics manufacturer. Their AI-enabled robots have been equipped with sophisticated vision systems and learning capabilities. These robots can “fine-tune” their quality inspection algorithms in real time. Imagine a robot that can improve its own accuracy with each inspection cycle, getting better with every product it reviews. That’s what’s happening at Fanuc, where AI is cutting defect rates while increasing throughput.
Then there's Tesla, which uses AI-powered cameras to inspect virtually every component that goes into its vehicles. AI analyzes everything from paint to gaps between panels to ensure each Model 3 that comes off the line looks and functions the way Elon Musk envisioned. The more Teslas they produce, the better their AI gets at predicting potential quality issues—and all of this happens without adding significant human intervention.

AI Algorithms in Quality Control: Not All Black Boxes
There’s a fear that AI in quality control can become too much of a black box—a mystical entity no one truly understands. But that’s not entirely true. The reality is that manufacturers are increasingly using explainable AI (XAI) to demystify quality control.

For instance, manufacturers are adopting machine learning algorithms that provide a feedback loop, identifying the specific reasons why a defect is flagged. A machine learning model that not only tells you a metal part is faulty but explains that the surface finish is out of tolerance by 15% is more valuable than one that simply says “fail.” Explainability improves the relationship between humans and AI, as workers understand precisely why the AI reaches its conclusions, and it helps improve the AI models over time.
Moreover, AI in quality control isn't just about deep learning black-box models. Rule-based systems, unsupervised learning, and hybrid approaches are being used where suitable—depending on the complexity and requirements of each product line. Manufacturers must pick the right AI tool for the job, not the flashiest or the most computationally expensive.
Data - The Backbone of AI Quality Control
At its heart, AI-powered quality control is a data game. It’s no surprise that a strong data management foundation is critical. Without high-quality data, AI cannot function effectively.

Data from IoT sensors, machine vision systems, and production logs is gathered, cleansed, and labeled—creating a foundation for training AI models. The power of AI comes from its ability to sift through colossal datasets and reveal hidden insights—things like the fact that a certain defect only happens when ambient temperatures reach a specific point, or a subtle pattern indicating a batch of raw materials isn't quite right.

Take Procter & Gamble, for example. In their diaper manufacturing facilities, quality data points are collected at multiple stages, from raw materials to the final product. AI analyzes these data points in near real-time to identify weak links in the process. This attention to data is not just about identifying defective products; it’s about anticipating potential failures before they affect the product.
Data is also used to create digital twins—virtual models of physical production systems. By simulating processes in a digital environment, manufacturers can test quality scenarios, understand how production changes impact the end product, and ensure their real-world production line is continuously optimized.
The Challenges and Opportunities
Of course, this isn’t all smooth sailing. Adopting AI for quality control presents unique challenges.
One of the main issues is cost—both in terms of equipment and training. AI systems require an upfront investment in high-speed cameras, sensors, and computational power. Additionally, the workforce needs retraining to understand how to interact with these AI systems. The fear of job loss—though largely unfounded as AI is a collaborative tool rather than a replacement—adds another layer of complexity to adoption.

But here lies an opportunity. The organizations that lean in and embrace AI find that the ROI can be substantial. Reduced scrap, fewer recalls, improved customer satisfaction, and enhanced brand loyalty. In sectors where reputation means everything—like aerospace or healthcare manufacturing—the cost of not implementing these tools could be much higher in the long run.
Moreover, regulatory hurdles are another significant concern. In highly regulated industries like pharma or aerospace, there is some skepticism regarding the validation and compliance of AI systems. Authorities are yet to adapt to the speed at which AI is changing, but that’s evolving as AI itself becomes more transparent and reliable.
Where Do We Go From Here?
The future is not about eliminating human workers but about redefining their roles. The symbiotic relationship between machines and humans will continue to evolve. Quality inspectors will shift from mundane, repetitive tasks to more strategic oversight roles, working alongside AI to make judgment calls where intuition and experience are still required. AI will support inspectors to do their job better, faster, and with greater precision—a genuine partnership.
Imagine a world where quality inspectors use augmented reality (AR) glasses, connected to AI systems, that highlight defects or deviations in real-time. This blending of human expertise with machine precision would take quality control to new heights. AI won’t just be analyzing; it will be empowering.
In this next frontier of manufacturing, excellence won’t be a one-time achievement—it’ll be a continuously moving target. AI will be the compass, constantly pointing us in the right direction.
Wrapping It All Up
AI-powered quality control is reshaping the boundaries of what's possible in manufacturing. It’s taking us beyond detection and allowing us to understand and predict problems before they arise. It’s transforming the role of quality from an assurance to a proactive, data-driven guarantee.
We’re still in the early days, but the seeds have been planted. Factories are becoming smarter, quality is becoming more intrinsic, and AI is the bridge taking us there. The next frontier isn’t some distant dream—it’s here. It’s about having the courage to make the leap and embracing the potential that lies beyond.
Manufacturing has always been about excellence. And now, with AI as a partner, that pursuit has never been more attainable.
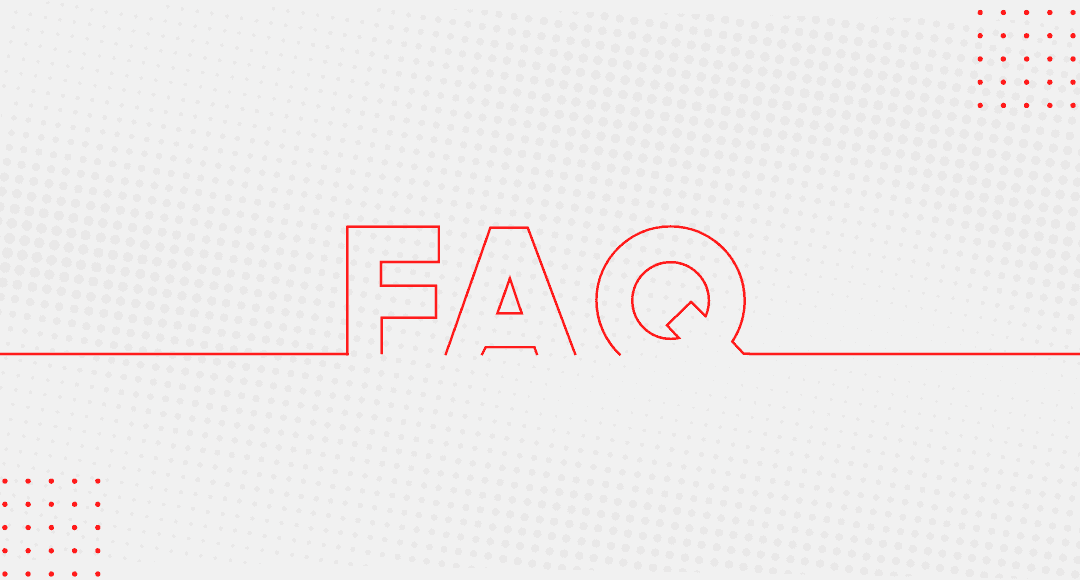
1. How does AI differ from traditional quality control methods in manufacturing?
AI moves beyond sampling by offering real-time, full-product inspections. Traditional methods rely on statistical sampling and manual inspection, which can miss defects and are prone to inconsistencies. AI ensures a 100% inspection rate, providing highly accurate quality analysis across the entire production line.
2. What industries are seeing the most benefit from AI-powered quality control?
Industries like automotive, pharmaceuticals, and consumer electronics benefit significantly. In automotive manufacturing, AI helps in real-time inspection of welding quality, while in pharmaceuticals, AI monitors packaging precision. Consumer electronics also use AI to ensure detailed inspection at the component level.
3. What types of AI models are used for quality control, and why?
Manufacturers use a mix of deep learning, rule-based models, and explainable AI. Deep learning is employed for complex visual inspections, while rule-based systems work well for simple, repetitive quality checks. Explainable AI (XAI) is valuable in regulated industries to provide transparency in defect identification.
4. Is AI-powered quality control only suitable for large manufacturing companies?
Not necessarily. AI solutions are scalable and can be adapted for small and medium-sized enterprises (SMEs) as well. The key is to evaluate the potential return on investment (ROI) and operational gains. SMEs can benefit from the improved quality, reduced waste, and predictive maintenance that AI offers.
5. Can AI-powered quality control systems replace human quality inspectors?
AI doesn’t eliminate the need for human inspectors. Instead, it augments their capabilities by handling repetitive and highly detailed inspections. Humans focus on strategic decision-making and quality assurance processes that require experience, judgment, and adaptability.
6. How do manufacturers handle the data requirements of AI quality control?
AI-powered quality control relies on large, high-quality datasets. Manufacturers gather data from sensors, production logs, and vision systems, which are cleaned and labeled for training AI models. Effective data management practices, including using cloud platforms and digital twins, are critical to support AI.
7. What are the primary challenges of implementing AI in quality control?
The main challenges are high initial costs, training requirements for the workforce, and regulatory compliance. Implementing high-speed cameras and sensors, along with computational resources, represents a significant investment. Employee retraining is also crucial for adapting to AI-driven workflows.
8. Are AI-driven quality control systems compatible with existing production lines?
Yes, AI-driven quality systems can be integrated with existing production lines, often with minimal disruption. Retrofitting involves adding cameras, sensors, and computing capabilities. Additionally, modern AI systems are designed to work with IoT infrastructure, enhancing compatibility.
9. How can AI help in predictive maintenance as part of quality control?
AI systems analyze sensor data such as vibration, sound, and temperature from production equipment to predict potential failures. This proactive approach allows manufacturers to address issues before they lead to significant downtimes, effectively linking quality control with equipment health.
10. Is AI quality control suitable for regulated industries like pharmaceuticals?
Yes, but careful consideration of regulatory compliance is needed. Explainable AI models are increasingly being used in these sectors to offer transparency in decision-making, which is critical for audit trails. In pharmaceuticals, AI can improve the accuracy of packaging inspections and ensure that all regulatory standards are met.
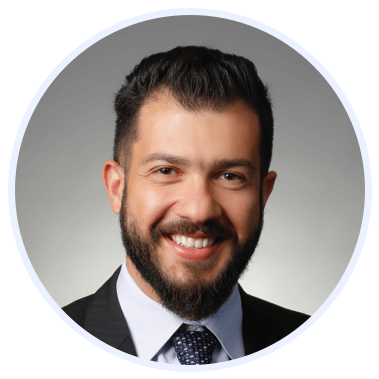
Rasheed Rabata
Is a solution and ROI-driven CTO, consultant, and system integrator with experience in deploying data integrations, Data Hubs, Master Data Management, Data Quality, and Data Warehousing solutions. He has a passion for solving complex data problems. His career experience showcases his drive to deliver software and timely solutions for business needs.