.jpg)
Effective data cleaning is necessary for the data analytics process. How do you go about accomplishing it, what exactly is it, and why is it important? Read on to find out.
Data cleaning is essential for business. To start with, it's a good idea to track your data and make sure it's correct and updated. Yet, data cleansing is an essential step in the data analytics process. If your data contains errors or inconsistencies, you may be certain that your conclusions will be erroneous. And it won't take a lot to see what could go wrong if you base business decisions on those insights!
The significance of quality data for companies wanting to succeed cannot be emphasized. Decisions you make based on data are as good as the quality of the data that it is based on.
Do you want to improve the quality of data for your organization? Capella can help, book a call today to find out how you can improve the quality of your data.
In this article, we will talk about the importance of data cleaning and the steps involved in the process. We will also discuss the challenges of data cleaning, the best practices to follow, and the tools and software that can help reduce data cleaning time.
Finally, we will answer some of the frequently asked questions about data cleaning. By the end of this article, you will have a better understanding of data cleaning and how to simplify the process.
What Is Data Cleaning?
Data cleaning is the process of preparing and organizing data for analysis. It includes identifying and correcting erroneous or missing data, eliminating unnecessary data, and formatting data in a usable way.
Data cleaning guarantees that the data is accurate and trustworthy, making it a crucial stage in the data analysis process.
To make sure they are working with the best possible data, data scientists and analysts frequently perform data cleansing. The procedure entails locating and fixing data mistakes, inconsistencies, and missing numbers. Moreover, data cleaning entails converting the data into a format that is better suited for analysis.

Why Is Data Cleaning Important?
Any data analysis or data mining method must include data cleaning. It is the process of making sure the data is correct, comprehensive, consistent, and pertinent to the analysis's goals. Data cleaning is necessary because it ensures that the data is ready for use and that any potential errors or inconsistencies are identified and addressed.
Data cleaning is beneficial since it lowers the possibility of mistakes during the analysis process. Without data cleaning, it is challenging to believe in the analysis's findings. Data cleaning makes sure that the data is correct and consistent, both of which are necessary for trustworthy outcomes.
Improves Quality Of Data
Data cleaning is important because it helps to improve the quality of the data. Poor quality data can lead to inaccurate results and can affect the accuracy of the analysis. Data cleaning helps to identify and correct any issues with the data, which can improve the accuracy and reliability of the analysis.
Reduces Time Needed To Analyze Data
Data cleaning is essential as it helps to reduce the amount of time and effort needed to analyze the data. Data cleaning helps to identify any issues with the data, which can help to lessen the time needed to analyze the data. This can help to reduce the cost and time associated with the analysis process.
Overall, data cleaning is an essential part of any data analysis process. It helps to ensure that the data is accurate, complete, consistent, and relevant for the analysis. Data cleaning is useful because it helps to reduce the risk of errors in the analysis process, improve the quality of the data, and minimizes the amount of time and effort needed to analyze the data.
Benefits Of Data Cleaning
Data cleaning is a necessary process that helps ensure the accuracy and quality of data. Data cleaning has many benefits, including improved data accuracy, better decision-making, and increased efficiency.
Data Accuracy
Data accuracy is one of the most useful benefits of data cleaning. Data cleaning involves removing any redundant data, such as typos and incorrect values. This ensures that any data used is accurate and reliable. This is especially vital when making decisions based on data, as decisions should be based on accurate data.
Improves Decision-Making
Data cleaning helps improve decision-making. By removing any inaccurate data, data cleaning helps ensure that any decisions made are based on reliable data. This helps ensure that any decisions made are the best decisions for the company or organization.
Increases Efficiency
Data cleaning also helps increase efficiency. By removing any unreliable data, data cleaning helps reduce the amount of time needed to process data. This helps decrease the amount of time needed to make decisions, saving time and money in the long run.
Challenges Of Data Cleaning
Data cleaning is an essential part of data analysis and can be a time-consuming process. There are a number of challenges that can arise during the data cleaning process that can make it difficult to complete the task efficiently and accurately.
Dealing With Missing Values
One of the biggest challenges of data cleaning is dealing with missing values. Many datasets contain missing values, which can make it difficult to interpret the data accurately. Missing values can be caused by a variety of factors, including data entry errors, data corruption, or incomplete data collection. It is beneficial to identify and address these missing values during the data cleaning process to ensure that the data is sufficient and complete.
Dealing With Outliers
Another challenge of data cleaning is dealing with outliers. Outliers are data points that are significantly different from the rest of the data. Outliers can be caused by errors in data collection or data entry, or they can be legitimate data points that are simply different from the rest of the data. It is essential to recognize and treat outliers during the data-cleaning process to make sure that the data is accurate and meaningful.
Requires Time And Effort
Data cleaning also requires much time and effort. Data cleaning is a labor-intensive process that requires a great deal of time and effort to complete. To guarantee that the data is cleaned correctly, it is important to dedicate enough time and resources to the task.
Data Is Complex
Finally, data cleaning can be a difficult task due to the complexity of the data. Many datasets contain complex data that is difficult to interpret and clean. It is vital to have the necessary skills and knowledge to effectively clean complex datasets to ensure that the data is accurate and meaningful.
Data cleaning is a necessary process for data analysis, but it can also be challenging. It is important to identify and handle various challenges of data cleaning to guarantee that the data is cleaned correctly and efficiently.
Steps Of Data Cleaning
Data cleaning is an essential part of data analysis, and it can be a time-consuming process. To make sure that your data cleaning process is efficient, it is vital to understand the different steps involved. Here are the steps of data cleaning that you should follow to establish the accuracy and quality of your data:
Identify The Data
The first and most crucial phase in the data cleaning process is data identification. Before attempting to clean the data, it is essential to understand it. Understanding the data kinds, data structures, connections between various data points, and any other information that might be pertinent is all part of this process.
It's useful to examine the data for any errors or inconsistencies while identifying it. This includes typos, wrong data types, erroneous values, and any other potential problems. Searching for any missing data or other potentially incomplete data is also crucial.
Understand The Data
Understanding the data is a crucial step in the data-cleaning process. This involves taking a closer look at the data to determine its structure, format, and content. It is critical to understand the data before attempting to clean it.
To understand the data, it is helpful to ask questions such as:
- What is the purpose of the data?
- What are the data types?
- What is the structure of the data?
- Are there any missing values?
- Are there any outliers?
- Are there any duplicates?
- Are there any inconsistencies?
- Are there any errors?
By asking these questions, you can better understand the data and identify any potential issues. This will help you to determine the best approach for cleaning the data.
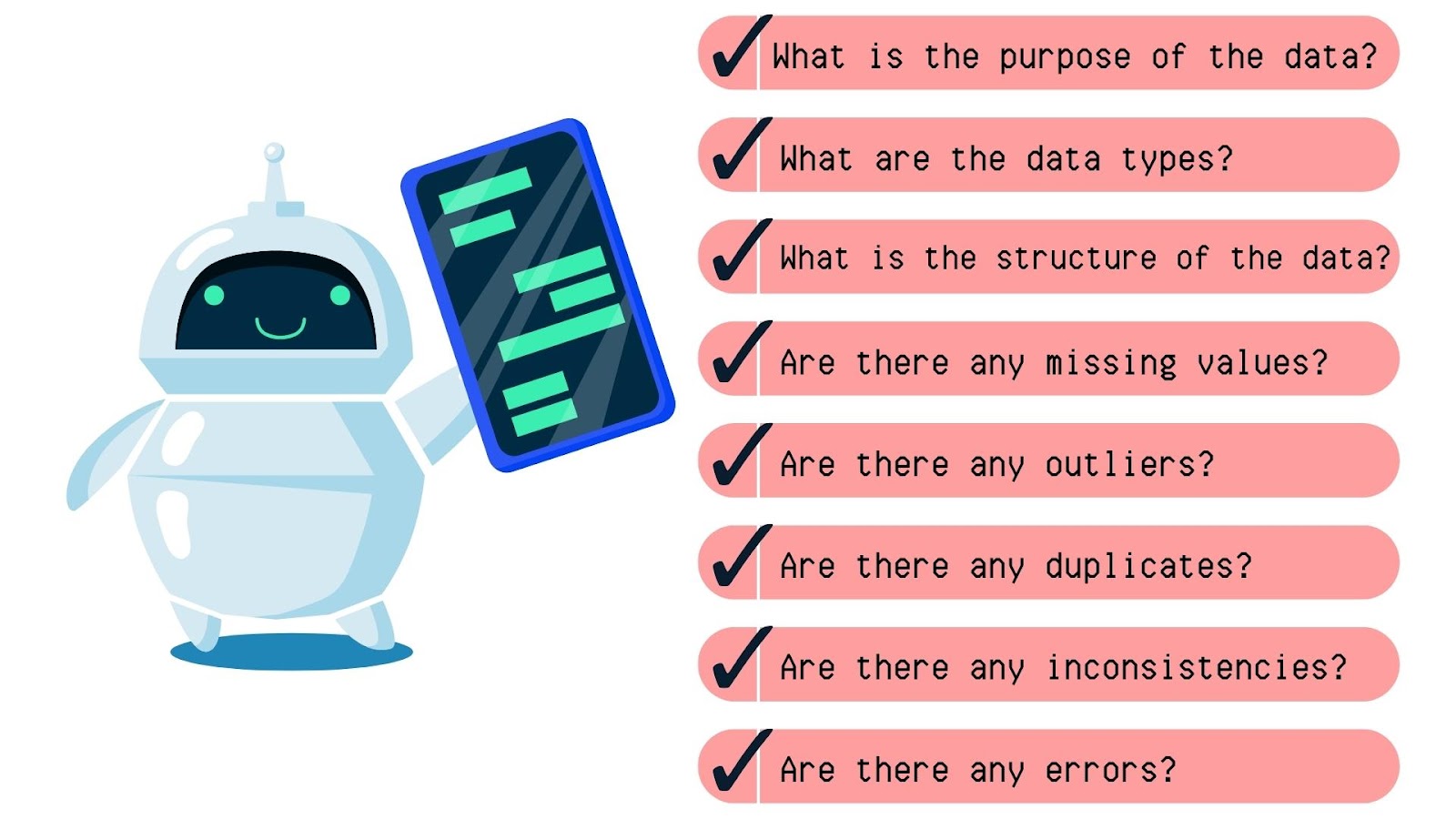
Check For Missing Values
A crucial phase in the data cleansing process is to look for missing values. Finding missing or incomplete data can help prevent erroneous results or conclusions. Each field in the dataset is examined to see if any values are missing or incomplete as part of the process of looking for missing values.
There are different methods for checking missing values. Visual inspection of the data is the approach that is used the most frequently. To do this, examine the data and manually spot any numbers that are missing. Another approach to find any missing values is to employ a statistical test like the chi-square test or the t-test.
It is helpful to note that not all missing values are necessarily bad. For example, some data sets may have intentional missing values, such as when a survey respondent does not answer a particular question. In these cases, it is beneficial to understand why the data is missing to make informed decisions about how to handle the missing values.
Remove Duplicates
In the data cleaning process, it is relevant to remove duplicate data. Duplicate data might interfere with data analysis and produce inaccurate or deceptive results. To ensure the data's accuracy and dependability, duplicate data must be found and eliminated.
The detection and removal of duplicate data can be done in several ways. Making use of a unique identifier to spot duplicate records is one of the most used techniques.
A combination of columns from the data set, like a customer ID or a product SKU, can serve as this unique identifier. Once the unique identifier has been found, it can be used to sort the data set to find duplicate records.
Check For Accuracy
One of the most significant aspects of data cleaning is data accuracy. It entails reviewing the data to look for any mistakes or discrepancies that might have happened during data entry or processing. Data reliability and accuracy ensure that the data may be used for insightful analysis and decision-making.
Reviewing the data and ensuring it is complete with no missing numbers is the first step in ensuring correctness. If there are any missing values, they need to be located and fixed.
Furthermore, any differences or inconsistencies across the data sets should be found and fixed. This can be carried out either manually or automatically using technologies like data validation software.
Standardize Data
Data standardization is a critical phase of the data cleaning process. Data must be transformed into a common format that is simpler to use and understand to be standardized. This step is especially vital when working with data from multiple sources, as it ensures that all data is in the same format and follows the same rules.
Depending on the type of data and the desired result, there are various methods for standardizing data. The format of the data, for instance, can be standardized to dd/mm/yyyy or mm/dd/yyyy if it contains dates. If the data includes text, the case can be standardized to either all lowercase letters or all capital letters.
Standardization of numerical data, such as currency values, is also essential. This can be done by converting all values to the same currency or by converting all values to a standard unit of measurement. For example, if the data contains US and Euro values, they can be converted to US dollars.
Handle Outliers
Outliers can skew results and lead to inaccurate conclusions, so it is better to identify and handle them properly.
Data cleaning techniques for handling outliers include:
1. Identifying And Removing Outliers
This involves manually examining the data to identify any present outliers. Once these outliers are identified, they can be removed from the dataset.
2. Using Statistical Methods
Statistical methods such as boxplots or standard deviation can be used to identify outliers in a dataset. Once identified, these outliers can be removed or replaced with a more appropriate value.
3. Imputing Values
Outliers can also be replaced with a more appropriate value. This can be done by calculating the data’s mean, median, or mode and replacing the outlier with the calculated value.
4. Clipping Values
Clipping is a data cleaning technique where extreme values are replaced with a more appropriate value. This is often done by setting a maximum and minimum value for the data, and any values outside of this range are replaced with the maximum or minimum value.
Verify Data Integrity
Data cleaning must begin with the verification of data integrity. This entails making sure all the data is current and verifying for mistakes and inconsistencies.
You should initially look for potential problems and inconsistencies before checking data integrity. Checking for duplicate entries, missing data, wrong data types, and inaccurate values could be part of this. You can then take action to address these concerns once you've discovered them.
You should also check that your data is consistent across different sources if you’re working with multiple data sources. This could involve checking for differences in formatting, data types, or values.
Document Cleaning Procedures
The data cleaning process must include the step of documenting your data cleaning practices. You can monitor your development and be certain that your data is accurate. If you need to clean the same data set again, being able to repeat your data cleaning techniques is another benefit of doing so.
It's useful to list every step you performed to clean your data when documenting data-cleaning operations. This includes any data processing you performed, such as removing columns or rows, combining datasets, or altering the data type. Provide any presumptions you had regarding the data, such as what specific values meant or how to handle missing values.
It's also a good idea to include notes about any errors or issues you encountered during the process. This can help you to identify and troubleshoot any potential problems in the future.
Test And Validity
Two important steps in the data cleansing process are testing and validity. Validity refers to the process of making sure the data is complete and adheres to predetermined norms, whereas testing refers to the process of ensuring the data is accurate and up to date. Both procedures are required to guarantee the data’s accuracy and ensure it is appropriate for the application for which it is intended.
To evaluate data for accuracy, completeness, and consistency, a number of tests are routinely run on the data. Depending on the complexity of the data and the available resources, these tests might be either human or automated. The tests should be created to find any errors or discrepancies in the data in either scenario.
Tools And Softwares For Reducing Data Cleaning Time
Data cleaning can be a time-consuming and labor-intensive process, but with the right tools and software, you can reduce the time and effort required to clean your data. Various tools and software are available to help you simplify the data-cleaning process. Each tool offers different features and capabilities, from data-wrangling tools to data-cleansing software.
Data Wrangling Tools
Data wrangling tools are done to help you easily manipulate and transform raw data into a usable format. These tools provide a graphical user interface (GUI) that enables you to quickly and easily identify and remove any unwanted data points and convert the data into the desired format. Popular data-wrangling tools include Trifacta, Alteryx, and Tableau Prep.
Data Cleansing Software
Data cleansing software help you identify and correct errors in your data. These tools provide powerful algorithms that can detect and correct errors in the data, such as typos, incorrect values, and duplicates. Popular data cleansing software includes DataMatch Enterprise, Data Ladder, and DQS.
Data Visualization Tools
Data visualization tools are intended to assist you to easily visualize your data. These tools include an intuitive interface that enables you to quickly and easily create charts, graphs, and other visualizations that can be used to identify patterns and trends in the data. Popular data visualization tools include Tableau, QlikView, and Microsoft Power BI.
Data Quality Tools
Data quality tools are built to help you assess the quality of your data and deliver powerful algorithms that can detect errors and inconsistencies in the data, as well as to identify any potential data issues. Popular data quality tools include Talend Data Quality, Informatica Data Quality, and SAS Data Quality.
Data Mining Tools
Data mining tools extract meaningful insights from your data and provide powerful algorithms that can identify patterns and trends in the data, as well as to detect any anomalies or outliers. Popular data mining tools include RapidMiner, KNIME, and Weka.
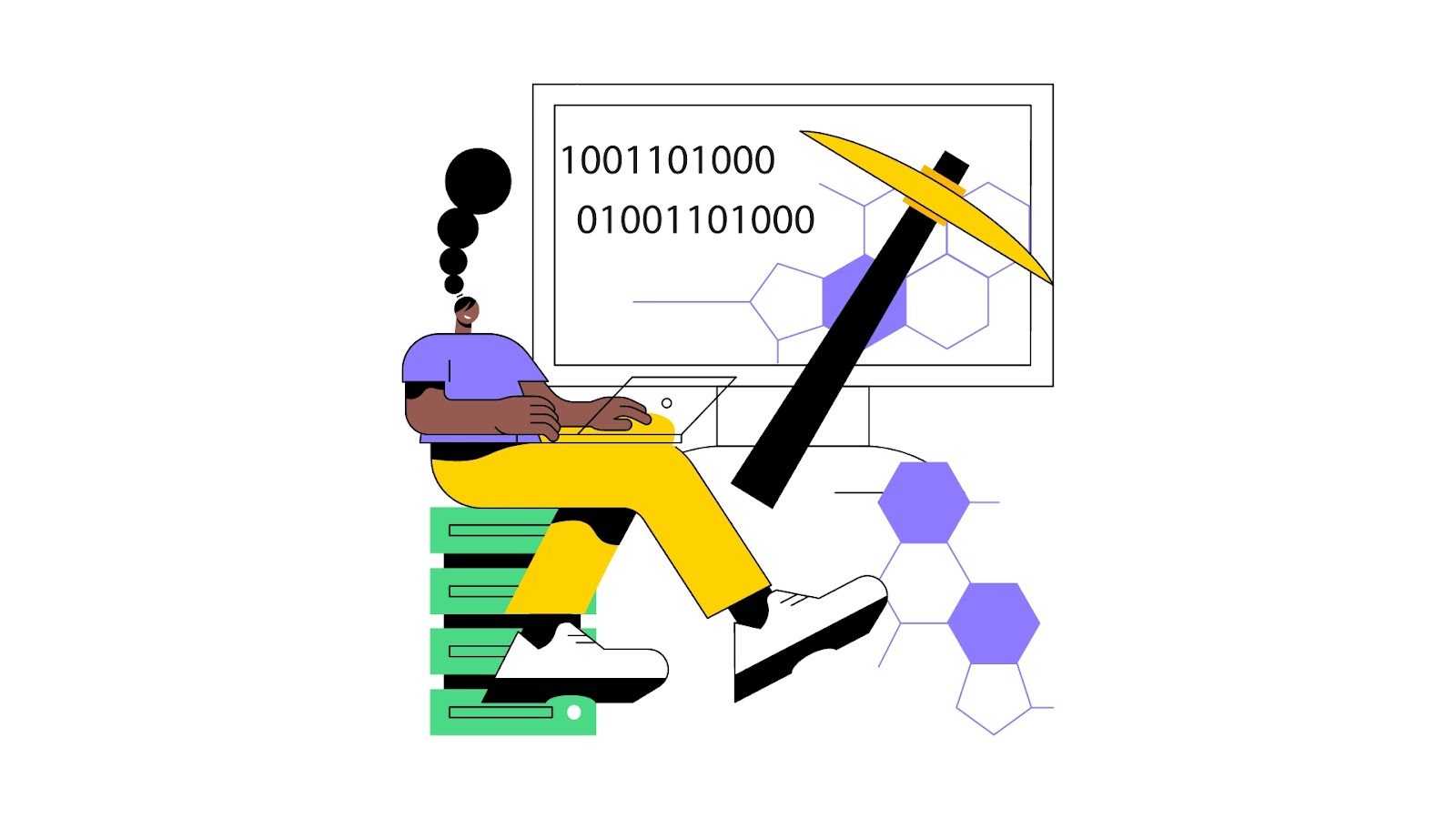
Final Thoughts
The key to unlocking the insights that can be drawn from data is data cleaning, which is a fundamental component of data analysis. You may streamline your data-cleaning procedure and save time by using the advice provided in this article.
The steps in the data cleaning process include identifying the data, comprehending the data, looking for missing values, eliminating duplicates, examining accuracy, standardizing data, handling outliers, ensuring data integrity, documenting cleaning procedures, testing and validity, and adhering to best practices.
Capella is one unique platform that offers you a combination of data hub and data service.
At Capella, We start by discussing your future strategy and analyzing your data sources. From there we would design a data-driven model for different parts of your business so you can make decisions on more than intuition.
Book a call today to data to analyze your data situation and develop a custom solution that works for your business.
FAQs
What are the different data cleaning strategies?
Data cleaning strategies can vary depending on the nature of the data and the desired outcome. Common strategies include identifying missing or inaccurate values, removing duplicates, standardizing data, handling outliers, verifying data integrity, and documenting cleaning procedures. Additionally, some strategies may involve data transformation and data integration.
How can data inconsistency be reduced?
Data inconsistency can be reduced by validating data, checking for accuracy, and standardizing data. Additionally, it is important to check for missing values and remove any duplicate records. Data cleansing techniques such as data deduplication, data scrubbing, and data transformation can also help to reduce data inconsistency.
Why does data cleaning take so long?
Data cleaning can take a long time due to the complexity of the data and the number of steps involved. Therefore, data cleaning can be time-consuming due to the need to identify and understand the data, check for accuracy, standardize data, handle outliers, verify data integrity, document cleaning procedures, and test for validity.
What is the difference between data cleaning and data cleansing?
Data cleaning and data cleansing are often used interchangeably, but they are two distinct processes. Data cleaning is the process of preparing data for analysis by identifying and correcting errors, while data cleansing is the process of transforming data into a more consistent and usable format. Data cleansing typically involves data transformation, data integration, and data deduplication.
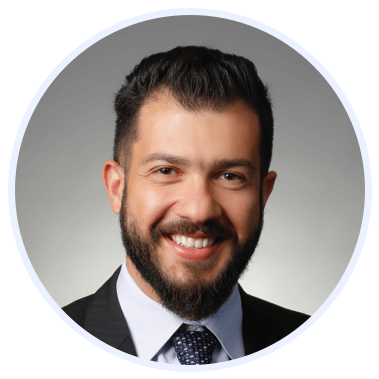
Rasheed Rabata
Is a solution and ROI-driven CTO, consultant, and system integrator with experience in deploying data integrations, Data Hubs, Master Data Management, Data Quality, and Data Warehousing solutions. He has a passion for solving complex data problems. His career experience showcases his drive to deliver software and timely solutions for business needs.