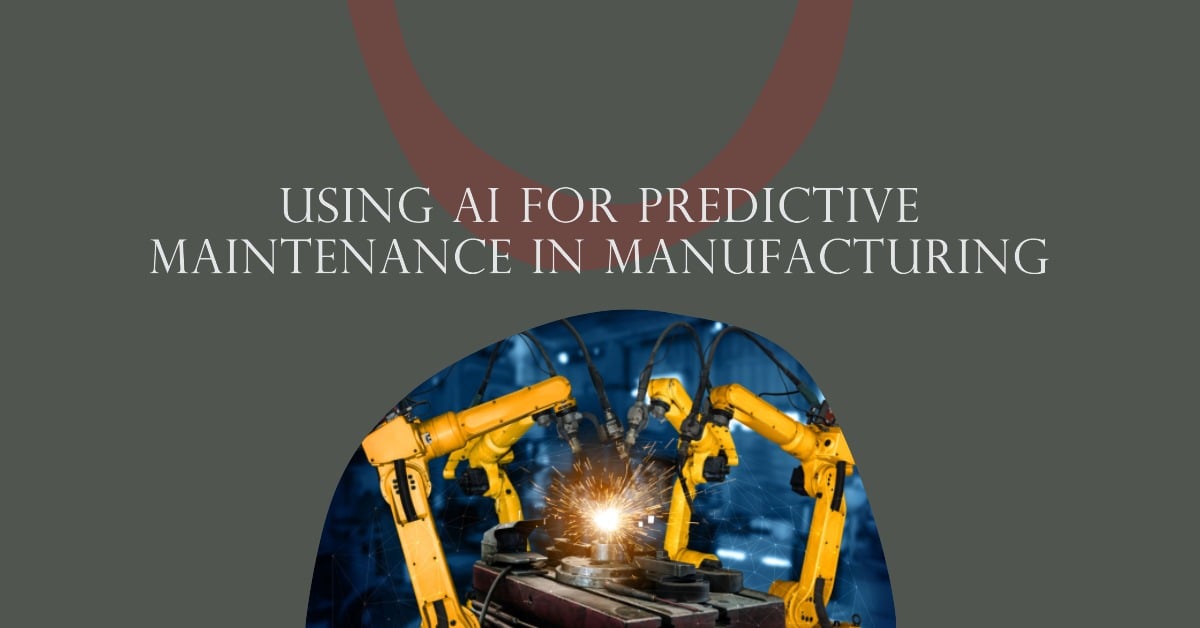

The role of artificial intelligence (AI) is rapidly expanding in every industry, and manufacturing is no exception. Predictive maintenance, in particular, is where AI has made a significant impact, empowering enterprises to foresee potential equipment failures and proactively tackle them. This blog post dives deep into the topic, discussing how AI-driven predictive maintenance is reshaping the manufacturing sector, bringing use cases and practical examples into the spotlight.
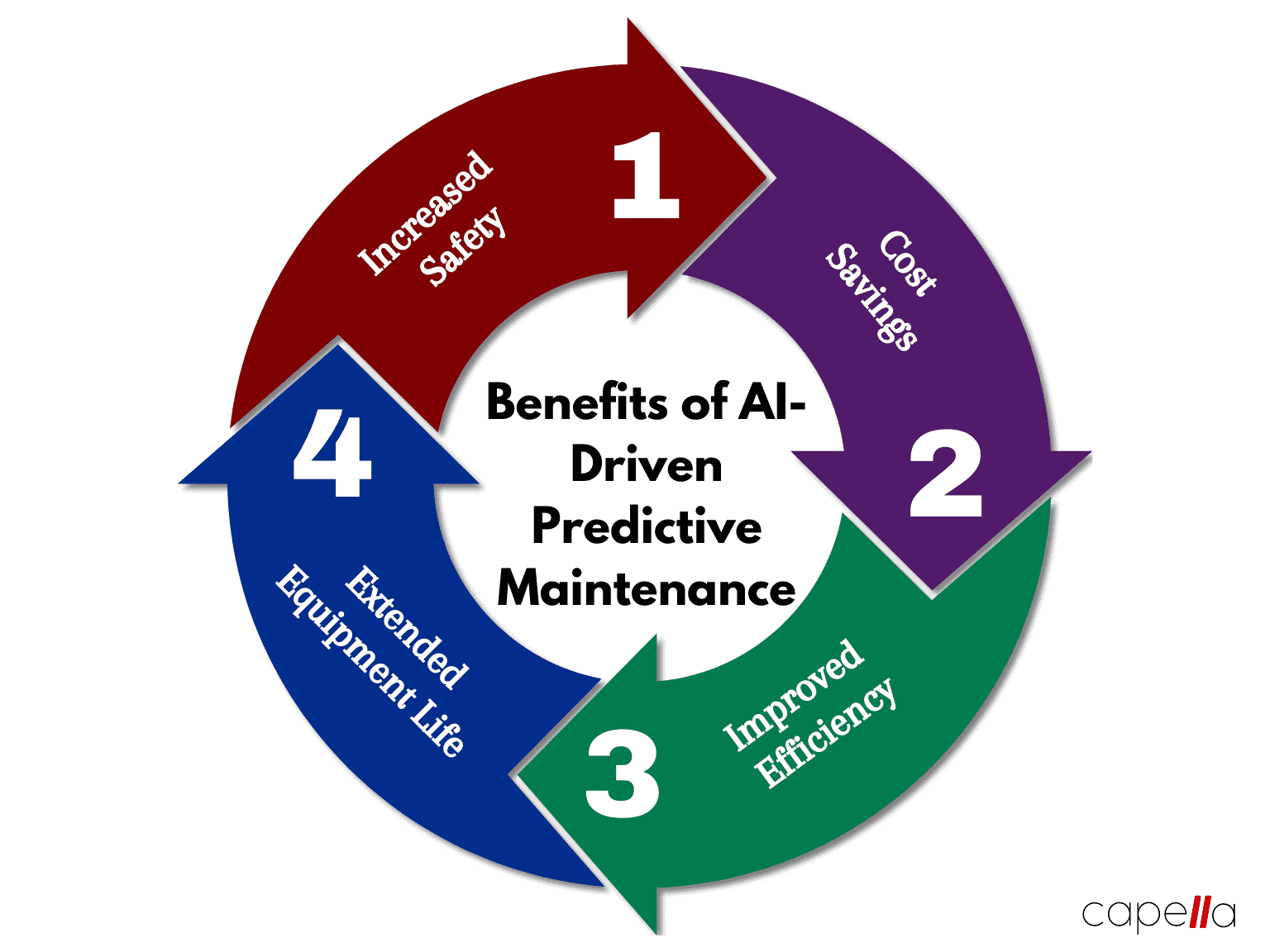
The Genesis of Predictive Maintenance
Before diving into how AI is transforming predictive maintenance, let's take a stroll down memory lane to appreciate the evolution of maintenance practices.
In the early days of industrial production, the dominant practice was "run-to-failure" – that is, let the machine operate until it broke down. Then came preventative maintenance, which prescribed routine check-ups and repairs based on manufacturers' recommendations, independent of the machine's actual condition.
Finally, the advent of technology and data analytics gave rise to predictive maintenance, which is based on the principle of detecting anomalies before they cause any significant issues. This is the place where AI is coming to play, enhancing predictive maintenance through intelligent data analysis and automation.

Understanding the Role of AI in Predictive Maintenance
Artificial Intelligence has been making waves in the tech world for years. Now, it's marching into manufacturing, transforming traditional practices into data-driven approaches. Here's how.
AI for Data Analysis
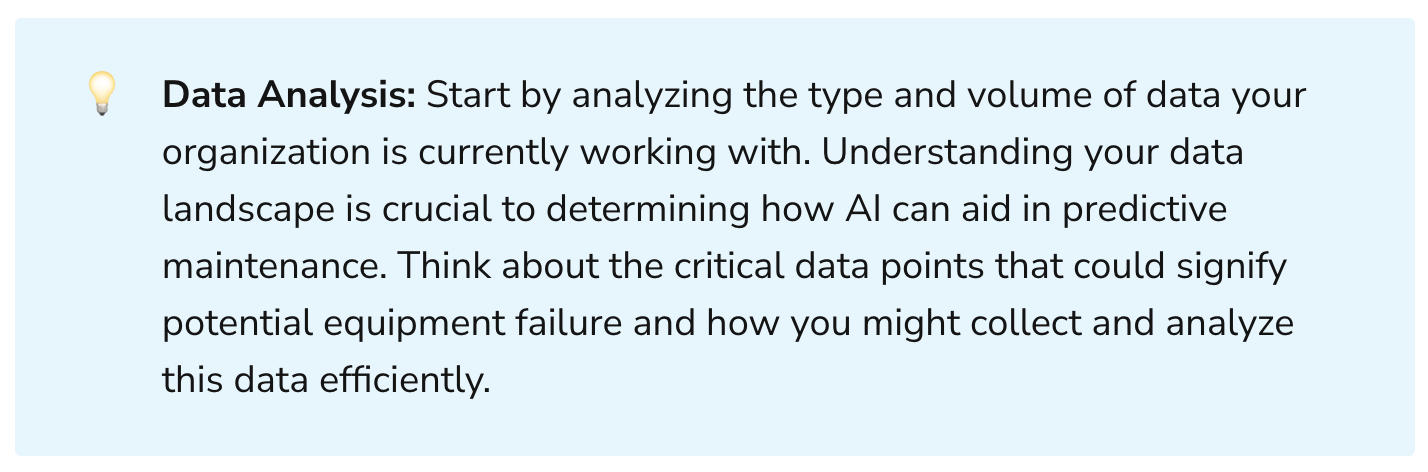
Traditional predictive maintenance is based on simple data analysis. It involves identifying patterns in historical data to predict future equipment failures. But what happens when your machine data is complex, scattered, and vast? That's where AI enters the scene.
AI tools are designed to handle vast amounts of complex data. They can learn from historical data, identify patterns that the human eye can't see, and predict potential equipment failures with high accuracy.
The National Institute of Standards and Technology (NIST) reports that the implementation of AI in manufacturing data analysis can reduce prediction errors by up to 50%. That's a statistic that any enterprise, large or small, can't afford to ignore.
AI for Automating Predictive Maintenance

Predictive maintenance is more than just predicting equipment failures. It also involves making decisions - what to do, when to do it, and how to do it. And this is another area where AI shines.
AI algorithms can automate decision-making processes. For example, they can analyze the impact of various maintenance actions and recommend the most cost-effective and efficient strategies.

Use Cases: AI-Powered Predictive Maintenance in Action
Now that we understand how AI is reshaping predictive maintenance let's examine some practical examples.
Predictive Maintenance in the Auto Industry

The auto industry has been one of the early adopters of AI-driven predictive maintenance. For instance, consider Tesla's use of AI for predictive maintenance in its production line.
Tesla has been using AI algorithms to analyze the vast amounts of data generated by its production equipment. These algorithms can identify patterns that predict potential equipment failures, allowing Tesla to address issues proactively, thus reducing downtime and increasing productivity.
Predictive Maintenance in the Aerospace Industry
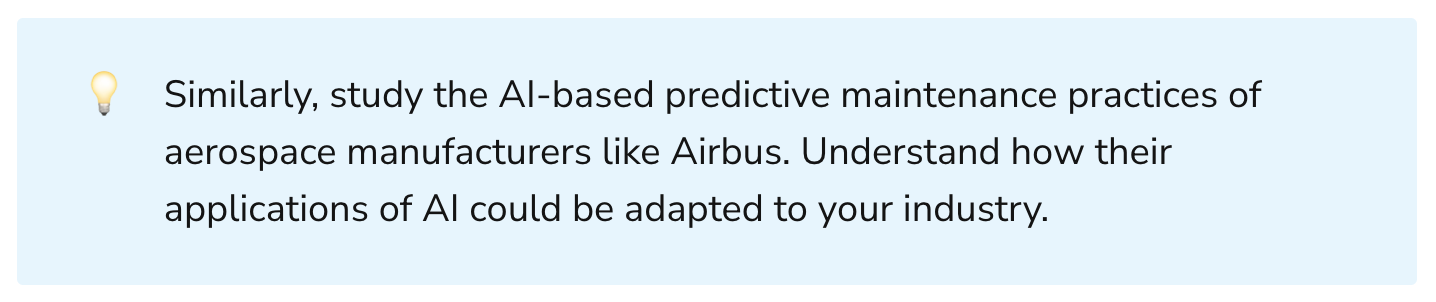
In the aerospace industry, aircraft manufacturers like Boeing and Airbus have been using AI for predictive maintenance of their production equipment and even their aircraft.
For instance, Airbus's Skywise platform, launched in collaboration with Palantir Technologies, uses AI algorithms to predict potential issues with aircraft components. This AI-driven approach allows airlines to conduct maintenance proactively, minimizing aircraft downtime and improving flight safety.
Why Should Enterprises Embrace AI-Driven Predictive Maintenance?
The examples above illustrate the potential of AI in predictive maintenance. But what are the specific benefits that should convince decision-makers to embrace this technology? Here are a few.
Cost Savings: Predictive maintenance, empowered by AI, can reduce maintenance costs by 25-30%, according to a study by Deloitte. This is achieved by optimizing maintenance activities, reducing equipment downtime, and preventing catastrophic failures.

Improved Efficiency: By reducing equipment downtime, AI-driven predictive maintenance can significantly increase production efficiency. The same Deloitte study mentioned above reports a 70-75% decrease in breakdowns with predictive maintenance.

Extended Equipment Life: Predictive maintenance can extend machinery life by 20-40%. It achieves this by identifying potential issues early and addressing them before they can cause significant damage.
Increased Safety: Lastly, by preventing catastrophic equipment failures, AI-driven predictive maintenance can improve workplace safety. This is particularly important in industries such as aerospace, where equipment failures can have severe consequences.

Implementing AI-Powered Predictive Maintenance: A Step-by-Step Guide
Embracing AI-driven predictive maintenance is not a one-step process. It requires careful planning and execution. Here's a step-by-step guide to get you started.
Data Collection: Start by collecting data from your machines. This could be done using IoT sensors or by tapping into your machine's built-in sensors.

Data Analysis: Once you have collected data, use AI algorithms to analyze it. Look for patterns that can predict equipment failures.

Model Building: Build a predictive model based on your data analysis. This model should be able to predict potential equipment failures and recommend maintenance actions.

Model Deployment: Deploy your model and start using it to guide your maintenance decisions. Make sure to continually monitor and improve your model based on its performance.

Review and Improve: Finally, continuously review your predictive maintenance practices and look for opportunities to improve. This could involve refining your AI algorithms, incorporating additional data sources, or optimizing your maintenance actions.

Wrapping Up
In conclusion, AI has immense potential in the realm of predictive maintenance. Its ability to analyze complex data and automate decision-making processes allows enterprises to anticipate equipment failures, optimize maintenance activities, and ultimately save costs and improve productivity. As decision-makers, it's imperative to understand this potential and start exploring opportunities to leverage AI in your predictive maintenance practices.
If you're just starting on this journey, remember it's a process, not a one-time event. So start small, learn from your experiences, and keep iterating and improving. The future of manufacturing is here, and it's powered by AI. Are you ready to embrace it?
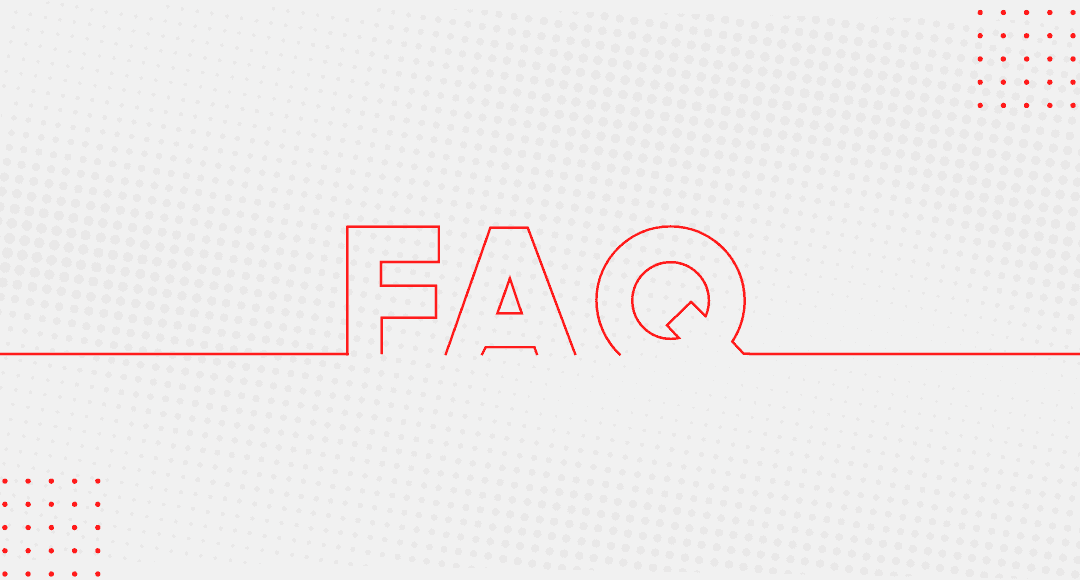
What is predictive maintenance?
Predictive maintenance refers to the use of data-driven, proactive maintenance strategies that aim to predict equipment failures before they occur. This approach allows for timely intervention, which can prevent costly downtime and extend the lifespan of machinery.
How does AI enhance predictive maintenance?
AI enhances predictive maintenance by providing advanced data analysis capabilities. Traditional predictive maintenance techniques rely on simple statistical analysis and human experience. In contrast, AI can handle vast amounts of complex data, identify subtle patterns, and make accurate predictions about equipment failures. Moreover, AI can automate decision-making processes, recommending the most effective maintenance strategies based on learned patterns.
What types of data are used in AI-driven predictive maintenance?
AI-driven predictive maintenance uses a variety of data, including machine operating parameters (such as temperature, pressure, and vibration levels), production data, environmental data, and historical maintenance records. This data can be collected through sensors embedded in the machines or provided by external IoT devices.
What industries can benefit from AI-driven predictive maintenance?
Virtually any industry that relies on machinery can benefit from AI-driven predictive maintenance. This includes manufacturing, transportation, energy, utilities, aerospace, automotive, and many others. By reducing downtime and maintenance costs, AI-driven predictive maintenance can enhance productivity and efficiency across a wide range of industrial contexts.
What are the main benefits of AI-driven predictive maintenance?
The main benefits of AI-driven predictive maintenance include cost savings, improved efficiency, extended equipment life, and increased safety. By predicting and preventing equipment failures, AI-driven predictive maintenance can reduce maintenance costs, minimize downtime, extend machinery lifespan, and prevent safety incidents.
How is AI-driven predictive maintenance implemented?
Implementing AI-driven predictive maintenance involves a series of steps. First, data is collected from the machines. Then, AI algorithms are used to analyze the data and build a predictive model. This model is then deployed and used to guide maintenance decisions. Finally, the predictive maintenance practices are continually reviewed and improved based on the model's performance.
How is the effectiveness of AI-driven predictive maintenance measured?
The effectiveness of AI-driven predictive maintenance can be measured using various key performance indicators (KPIs), such as downtime reduction, maintenance cost savings, equipment lifespan extension, and safety incidents reduction. These KPIs should be monitored regularly to assess the performance and impact of AI-driven predictive maintenance.
What are the challenges of implementing AI-driven predictive maintenance?
Implementing AI-driven predictive maintenance can present several challenges. These include data quality issues (since predictive models rely on high-quality, accurate data), the need for advanced technical skills (to develop and maintain AI models), and the requirement for substantial upfront investment (in data collection devices and AI software). However, these challenges can be overcome with careful planning and execution, and the potential benefits of AI-driven predictive maintenance often far outweigh the initial hurdles.
What role does IoT play in AI-driven predictive maintenance?
The Internet of Things (IoT) plays a crucial role in AI-driven predictive maintenance by providing the data needed for AI algorithms. IoT devices, such as sensors and smart meters, can collect a vast array of data from machinery in real time. This data can then be analyzed by AI algorithms to predict potential equipment failures and guide maintenance decisions.
How is AI-driven predictive maintenance likely to evolve in the future?
The future of AI-driven predictive maintenance is likely to be shaped by advancements in AI algorithms, increased adoption of IoT devices, and the growth of digital twin technology. AI algorithms are becoming increasingly sophisticated, allowing for more accurate and nuanced predictions. IoT devices are becoming more affordable and widespread, enabling more comprehensive data collection. And digital twin technology, which creates a digital replica of a physical system, offers exciting new opportunities for predictive maintenance by allowing for virtual testing and simulation.
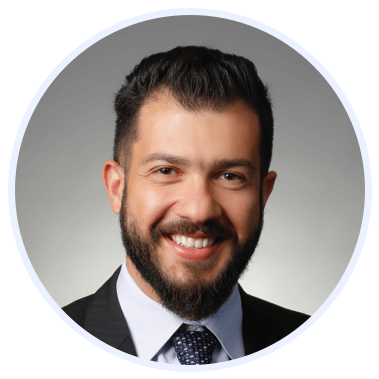
Rasheed Rabata
Is a solution and ROI-driven CTO, consultant, and system integrator with experience in deploying data integrations, Data Hubs, Master Data Management, Data Quality, and Data Warehousing solutions. He has a passion for solving complex data problems. His career experience showcases his drive to deliver software and timely solutions for business needs.