
Advancements in materials science have always shaped our world. From the industrial revolution to the silicon age, new materials have driven innovation. But the pace of discovery has often been slow. The testing, tweaking, and refining of materials can take years. Today, artificial intelligence (AI) is changing that.
AI accelerates the discovery process, reduces costs, and opens doors to previously unimaginable possibilities. For R&D teams and process innovators, the integration of AI into materials research isn’t just a trend; it’s a transformation.

Why Materials Innovation Matters
Materials are the backbone of progress. The steel in bridges, the silicon in chips, the polymers in your phone’s casing — all came from meticulous research. When a new material is discovered, industries shift.
Take lightweight composites in aerospace. They reduced fuel consumption and made air travel cheaper. Or lithium-ion batteries in consumer electronics. They transformed how we store and use energy. Each breakthrough reshapes markets, creating competitive advantages for those ahead of the curve.
Yet, developing these materials has traditionally been a slow, resource-intensive process. The trial-and-error approach of the past cannot keep up with modern demands. Industries now require faster solutions, and AI offers the perfect answer.

The AI Advantage in R&D
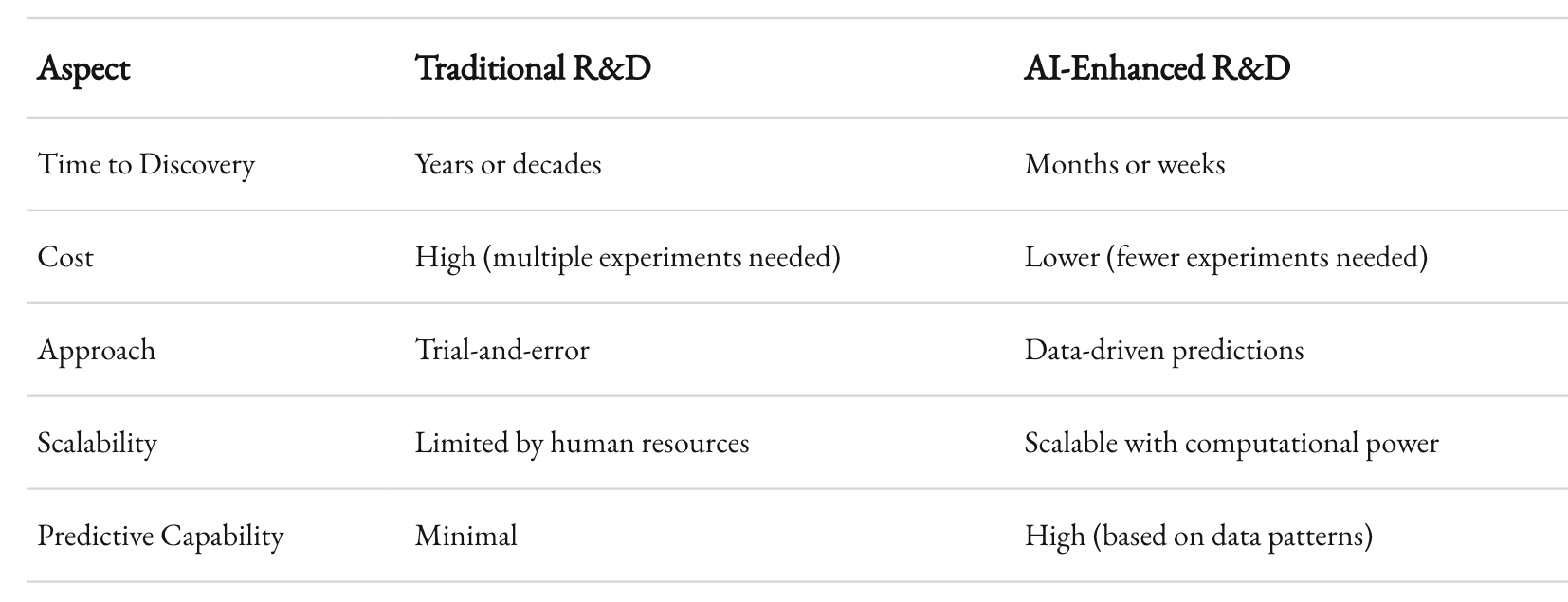
AI doesn’t just speed things up. It redefines how we think about discovery. Here’s how:
- Prediction Over Experimentation
Traditional research relies on physical experiments. AI, with machine learning (ML) models, predicts outcomes without needing a lab. It analyzes vast datasets to uncover relationships between variables, guiding researchers toward the most promising solutions. - High-Throughput Analysis
AI processes thousands of data points in seconds. For instance, quantum mechanical simulations that once took weeks can now be completed in hours. This allows researchers to test multiple hypotheses simultaneously. - Uncovering Hidden Patterns
Some material properties are influenced by intricate factors. AI excels in identifying hidden correlations that might be missed by human analysis. These insights can direct innovation toward areas ripe for breakthroughs.

Real-World Applications

Faster Material Discovery
AI helps predict the properties of new compounds before they are synthesized. Companies like Citrine Informatics and researchers at leading universities use AI-driven tools to screen millions of potential materials for specific characteristics, such as conductivity or tensile strength.
For example, in battery research, AI models have identified novel electrode materials that enhance energy density. These discoveries cut years from the R&D timeline.
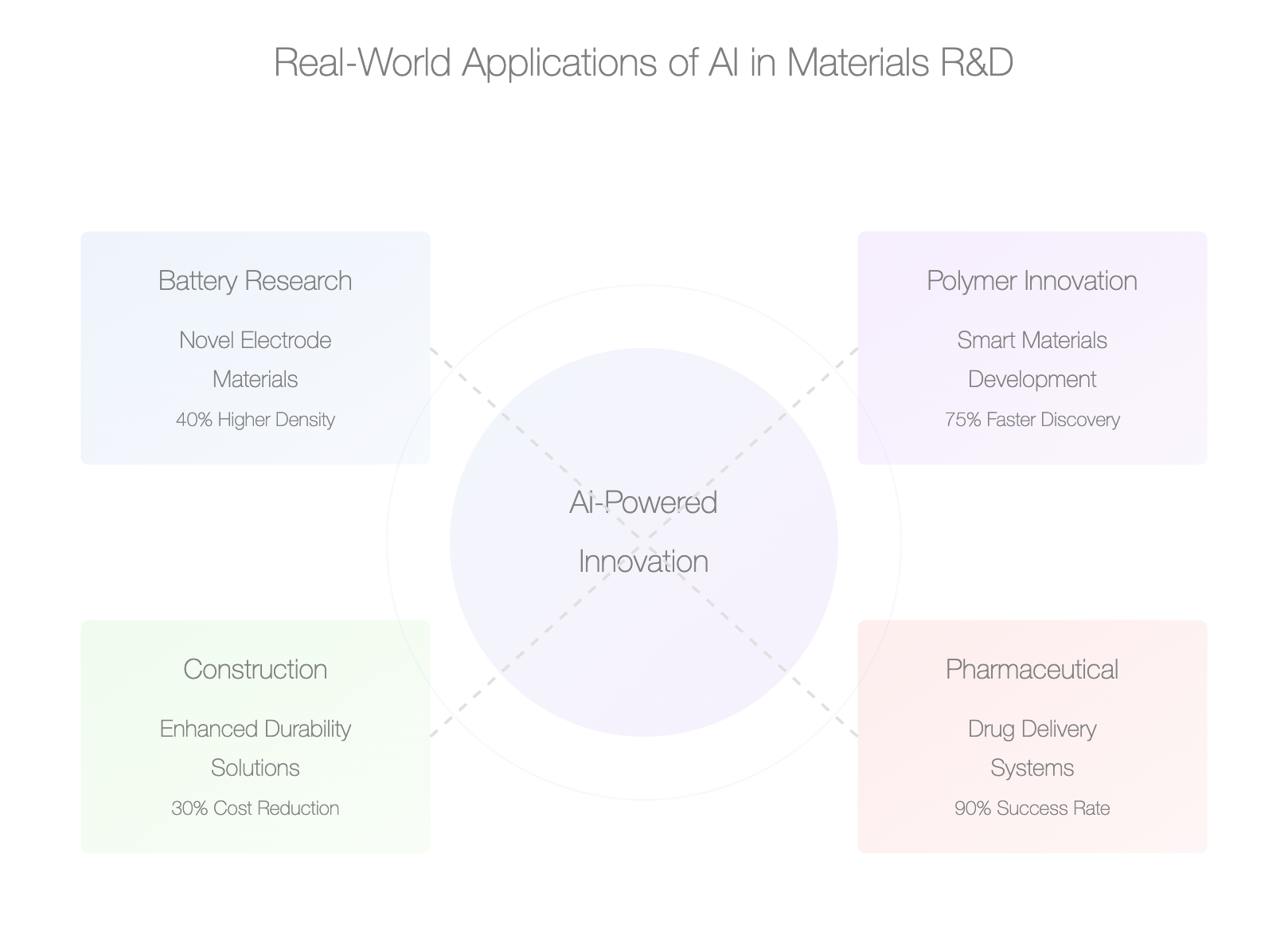
Enhanced Testing Protocols
Testing remains a critical part of materials development. AI optimizes this phase too. Through predictive modeling, researchers can focus on the most promising candidates, reducing wasted resources.
Consider polymer research. Instead of synthesizing hundreds of variations, AI narrows the field to a handful of top contenders. This saves time, reduces costs, and accelerates product development cycles.
Optimizing Existing Materials
AI isn’t just about new discoveries. It’s about refining what we already have. For example, advanced algorithms have helped improve the durability of construction materials, making them more sustainable without increasing costs.
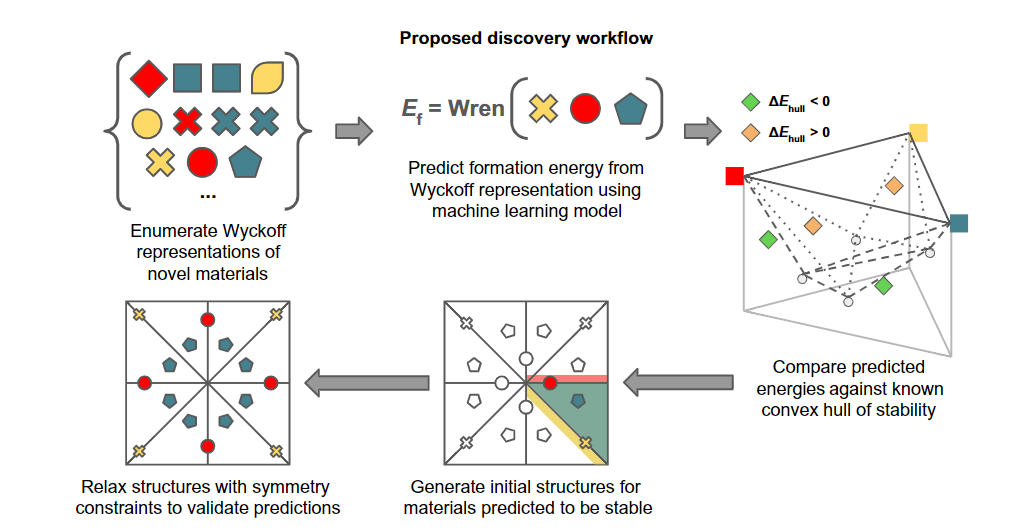
The Workflow: AI in Action
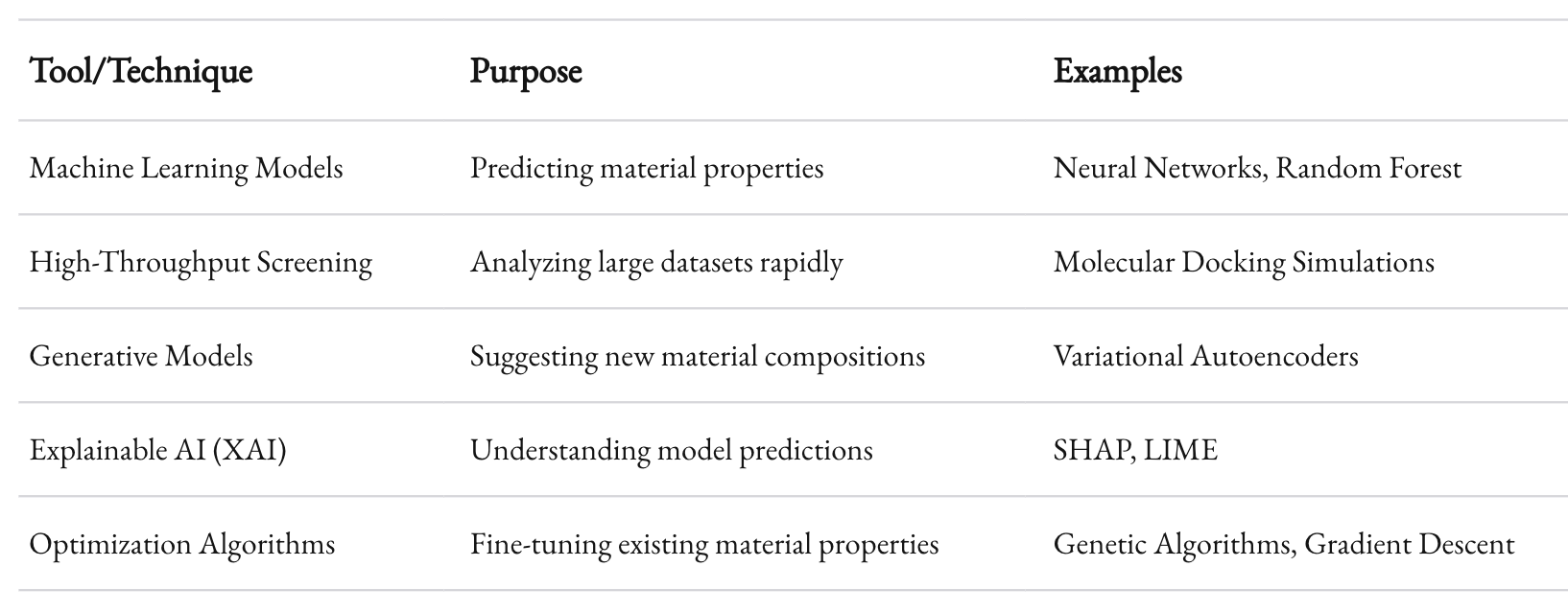
Data Collection and Curation
Every AI model thrives on data. For materials science, this includes:
- Experimental results from past research.
- Computational simulations.
- Published literature.
- Industry-specific datasets.
Curating clean, structured data is the first step. Without it, even the best AI algorithms falter.
Model Development
Next, machine learning models are trained to predict material properties. Techniques like neural networks, random forests, and support vector machines dominate this phase. Researchers tweak these models to achieve maximum accuracy.
Validation and Testing
AI predictions are validated against real-world results. This iterative process ensures reliability and builds confidence in the AI’s recommendations.
Deployment in Research
Finally, these tools are integrated into everyday workflows. From screening compounds to simulating performance, AI becomes a seamless part of the R&D toolkit.

Challenges and Solutions
Challenge: Data Scarcity
Not all materials have extensive datasets. When data is sparse, AI models may struggle to produce accurate predictions.
Solution: Transfer learning and generative models can help. By leveraging knowledge from similar materials, these techniques fill gaps and extend AI’s capabilities.
Challenge: Interpretability
AI models often operate as “black boxes,” making it difficult for researchers to understand why certain predictions are made.
Solution: Explainable AI (XAI) tools are evolving to address this. They provide insights into the decision-making process, fostering trust and usability.
Challenge: Integration
Incorporating AI into traditional workflows can face resistance from teams unfamiliar with the technology.
Solution: Training and collaboration are key. Cross-functional teams combining data scientists and materials experts ensure smoother adoption.

Case Studies
Breakthroughs in Pharmaceuticals
Pharmaceutical R&D relies heavily on material properties. AI has streamlined drug delivery systems by predicting how compounds interact with carriers, leading to more effective treatments.
Revolutionizing Construction
The construction industry has seen significant improvements in concrete formulations. AI-enhanced models predict the optimal mix of ingredients to improve strength and sustainability.
Greener Energy Solutions
In renewable energy, materials like perovskite for solar cells have benefited from AI’s predictive capabilities. This has accelerated their adoption and reduced costs.
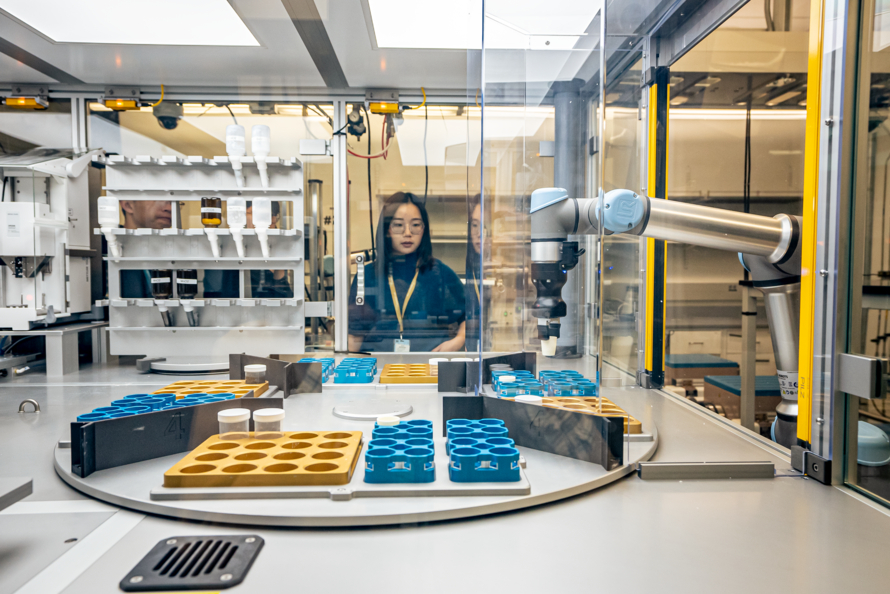
The Future of AI in Materials Science
The impact of AI in materials R&D is only beginning. Here’s what lies ahead:
- Autonomous Labs: Fully automated research facilities driven by AI. They’ll conduct experiments, analyze results, and refine hypotheses without human intervention.
- Collaboration at Scale: AI platforms connecting researchers globally to share insights and accelerate progress.
- Sustainability Focus: Materials designed with AI to minimize environmental impact, from recyclable composites to carbon-neutral alloys.
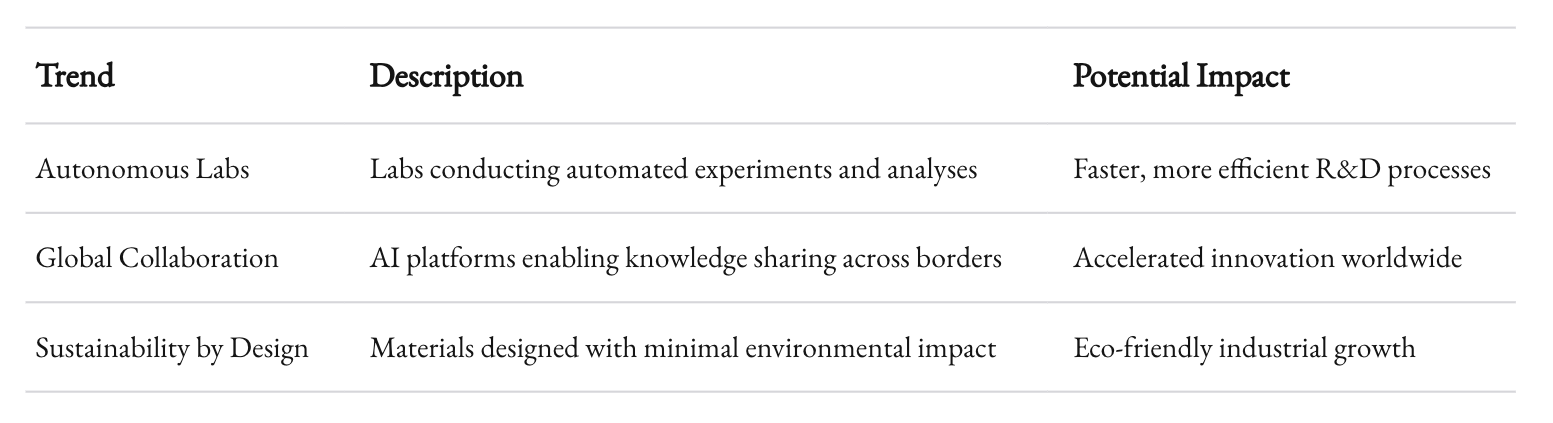
Recap
AI has transformed materials R&D from a slow, iterative process to an agile, insight-driven endeavor. For R&D teams and process innovators, embracing this technology isn’t optional; it’s essential.
Whether it’s discovering the next breakthrough material, optimizing existing solutions, or driving sustainability, AI is the catalyst for change. As industries evolve, those who integrate AI into their research processes will lead the charge. The future is here. And it’s intelligent.
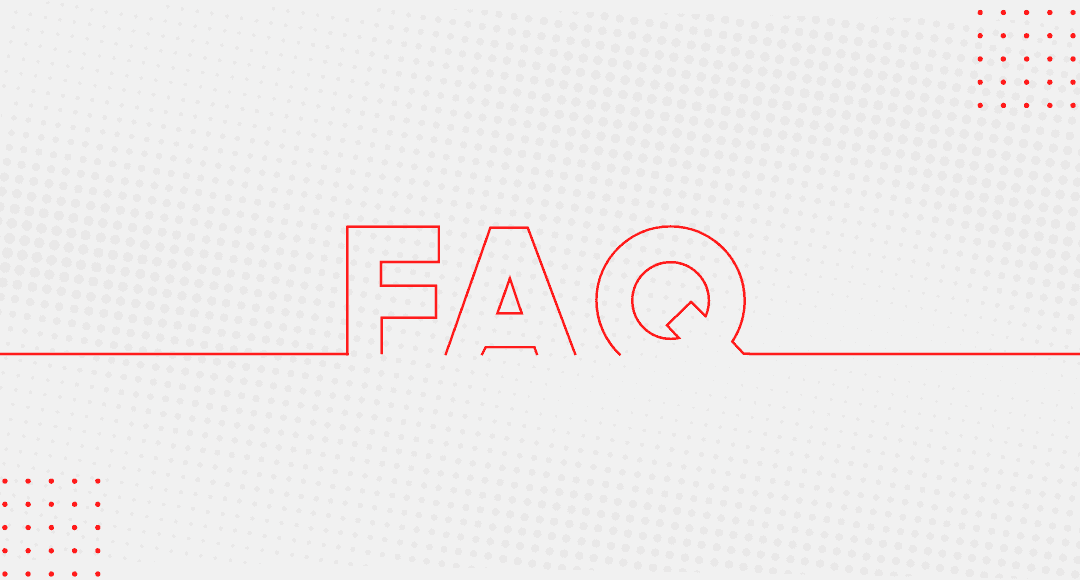
1. What is AI-enhanced materials R&D?
AI-enhanced materials R&D refers to the application of artificial intelligence technologies to accelerate the discovery, testing, and optimization of new materials. By analyzing vast datasets and simulating potential outcomes, AI allows researchers to predict material properties, identify optimal compositions, and reduce the need for time-consuming experiments.
2. How does AI make materials discovery faster?
AI can predict the properties of new materials using machine learning models trained on existing datasets. This prediction reduces the need for extensive trial-and-error experiments in the lab. Moreover, high-throughput analysis powered by AI allows researchers to test thousands of hypotheses simultaneously, saving months or even years in the discovery timeline.
3. What kinds of materials benefit from AI-driven research?
AI has been successfully applied to a wide range of materials, including but not limited to:
- Advanced composites for aerospace and automotive industries.
- Electrode materials for batteries and energy storage.
- Polymer blends for packaging and consumer goods.
- Alloys for construction and manufacturing.
- Materials for renewable energy, such as perovskites in solar cells.
4. Is AI replacing human researchers in materials science?
No, AI is not replacing human researchers. Instead, it is a tool that enhances their capabilities. AI can handle repetitive data analysis tasks, identify patterns, and provide insights, allowing scientists to focus on creativity, hypothesis generation, and complex decision-making. The collaboration between human expertise and AI creates the best outcomes.
5. What role does data play in AI-enhanced R&D?
Data is the foundation of AI-enhanced R&D. Machine learning models rely on clean, structured datasets to make accurate predictions. This data can come from past experiments, computational simulations, or even literature reviews. The more comprehensive and high-quality the dataset, the better the AI's performance.
6. Are there challenges in adopting AI for materials research?
Yes, there are challenges. These include:
- Limited datasets for certain niche materials.
- Difficulty in interpreting complex AI models (the “black-box” problem).
- High upfront costs for infrastructure and tools.
- Resistance to change from teams accustomed to traditional workflows. However, solutions such as transfer learning, explainable AI, and cross-functional training programs are addressing these hurdles.
7. Can small and medium-sized R&D teams afford AI tools?
Yes, AI tools have become increasingly accessible. Cloud-based platforms and open-source frameworks lower the cost barrier for small and medium-sized teams. Additionally, partnerships with academic institutions or leveraging grants can help smaller teams adopt these technologies without significant capital investment.
8. How does AI improve sustainability in materials R&D?
AI helps design materials that are more efficient and environmentally friendly. For example, it can identify formulations that reduce waste, require less energy to produce, or are easier to recycle. AI can also optimize the use of renewable resources, supporting the development of sustainable alternatives to traditional materials.
9. What industries benefit the most from AI-enhanced materials research?
Industries such as aerospace, automotive, energy, pharmaceuticals, and construction see significant benefits. For example, AI-driven advancements in lightweight composites have reduced fuel consumption in aerospace, while predictive modeling in pharmaceuticals has streamlined drug delivery systems. Renewable energy and green construction also benefit from AI-designed sustainable materials.
10. What does the future hold for AI in materials science?
The future is promising. Autonomous labs powered by AI will enable faster, fully automated research processes. Collaborative platforms will allow researchers worldwide to share data and insights in real time. Sustainability-focused designs will become the norm, driven by AI's ability to optimize material properties for minimal environmental impact. The integration of AI into materials science will redefine how industries innovate.
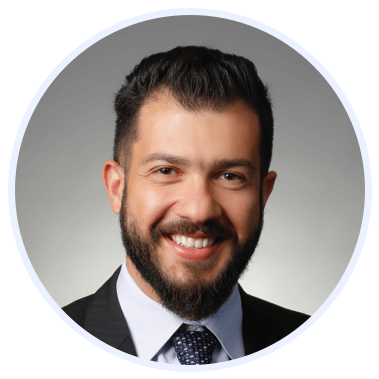
Rasheed Rabata
Is a solution and ROI-driven CTO, consultant, and system integrator with experience in deploying data integrations, Data Hubs, Master Data Management, Data Quality, and Data Warehousing solutions. He has a passion for solving complex data problems. His career experience showcases his drive to deliver software and timely solutions for business needs.