
I recently visited a manufacturing facility where a critical CNC machine had just failed unexpectedly. Production ground to a halt. Workers stood idle. Customer deadlines loomed. The maintenance team scrambled to diagnose the issue while operations managers calculated the mounting costs of downtime. The frustration was palpable.
It didn't have to be this way. After spending over a decade helping companies optimize their operations, I've seen firsthand how predictive modeling and AI can transform asset management from reactive firefighting into proactive lifecycle optimization. The right predictive maintenance strategy doesn't just prevent catastrophic failures – it extends equipment life, reduces operating costs, and keeps production running smoothly.
What surprises many operations leaders is that implementing predictive maintenance isn't as complex or disruptive as they fear. The key is starting small, focusing on critical assets, and gradually expanding based on proven results. In this post, I'll share practical insights from my experience helping mid-sized manufacturers modernize their asset management approach.
The True Cost of Reactive Maintenance
Most manufacturing operations still rely heavily on reactive maintenance – fixing equipment after it breaks down. While this might seem cost-effective in the short term, the true costs are staggering. Beyond the immediate expense of emergency repairs and replacement parts, there are the harder-to-quantify costs: lost production time, missed delivery deadlines, rushed shipping fees, and damaged customer relationships.
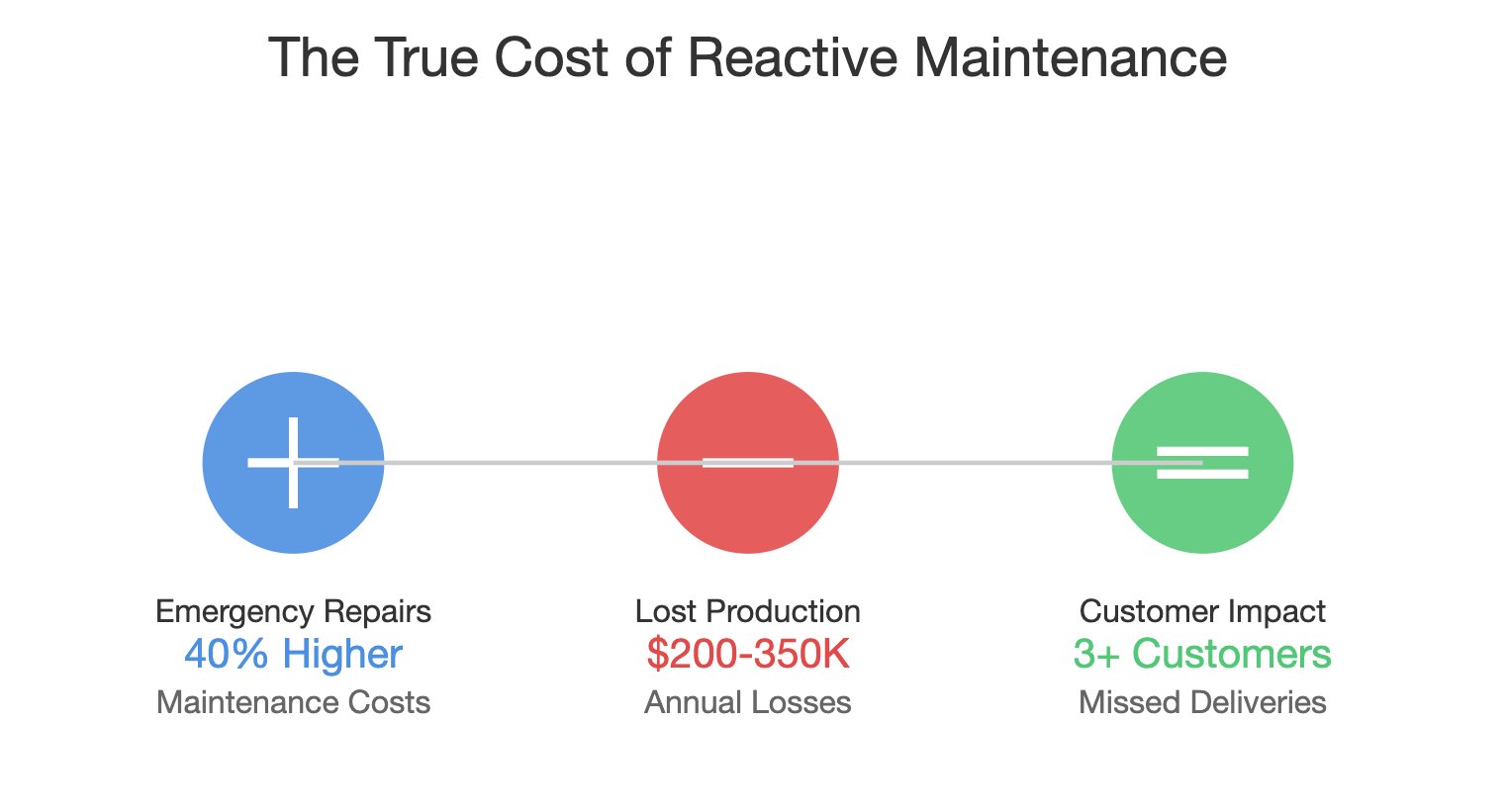
I recently worked with a metal finishing company that was losing $200,000 to $350,000 annually just from misplaced or poorly maintained equipment. Their maintenance team was constantly in "firefighting mode," dealing with emergency repairs rather than focusing on preventive measures. This reactive approach meant they were spending 40% more on maintenance than necessary, while still suffering frequent unexpected downtime.
The breaking point came when a critical plating line went down during their busiest season, causing them to miss deliveries to three major customers. That's when they realized their approach to asset management needed a complete overhaul.
The Predictive Advantage
Modern predictive maintenance combines sensor data, machine learning algorithms, and domain expertise to forecast when equipment is likely to fail. But it's not just about preventing failures – it's about optimizing the entire lifecycle of your assets.
Here's what makes predictive maintenance transformative:

- Early Warning System: By analyzing patterns in equipment behavior, predictive models can identify potential issues weeks or months before they cause problems. This gives maintenance teams time to plan repairs during scheduled downtime.
- Optimized Maintenance Intervals: Instead of following rigid maintenance schedules, you can maintain equipment based on its actual condition and usage patterns. This prevents both over-maintenance and under-maintenance.
- Extended Asset Life: By catching and addressing minor issues before they become major problems, you can significantly extend the useful life of your equipment.
- Better Resource Allocation: Maintenance teams can work more efficiently by focusing on the equipment that truly needs attention, rather than performing unnecessary routine checks.
Starting Your Predictive Journey
The path to predictive maintenance doesn't require a massive upfront investment or wholesale replacement of existing systems. Here's the approach I recommend to my clients:

Step 1: Asset Prioritization
Begin by identifying your most critical assets – those where failure would cause the most significant disruption to your operations. For most manufacturers, this represents about 20% of their equipment but accounts for 80% of potential downtime costs.
Create a simple prioritization matrix:

Step 2: Data Collection and Analysis
Start collecting relevant data from your priority assets. This might include:
- Operating temperatures
- Vibration readings
- Power consumption
- Production rates
- Quality metrics
- Maintenance history

Modern sensors are surprisingly affordable and can often be retrofitted to existing equipment. The key is collecting the right data, not necessarily the most data.
Step 3: Model Development and Implementation
With good data in hand, you can begin developing predictive models. These models learn from historical patterns to identify potential issues before they cause failures. I've seen companies achieve 85-95% accuracy in predicting equipment failures up to two weeks in advance.
The most effective approach combines machine learning algorithms with human expertise. Your maintenance team's knowledge is invaluable in interpreting model outputs and fine-tuning predictions.

Real-World Results
Let me share a recent success story. A precision parts manufacturer I worked with implemented predictive maintenance on their five most critical CNC machines. Within the first year, they achieved:
- 32% reduction in unplanned downtime
- 21% decrease in maintenance costs
- 18% improvement in machine lifecycle
- ROI of 3.2x on their predictive maintenance investment

But the benefits went beyond the numbers. Maintenance technicians reported feeling more in control and less stressed. Production managers could plan with greater confidence. And customers noticed the improvement in delivery reliability.
Common Challenges and Solutions
While the benefits of predictive maintenance are clear, implementation isn't without challenges. Here are the most common obstacles I've encountered and how to overcome them:

Data Quality Issues
Many companies struggle with inconsistent or incomplete data. The solution is to start small and focus on quality over quantity. Begin with a single critical asset and ensure you're collecting clean, reliable data before expanding.
Resistance to Change
Maintenance teams sometimes resist new technologies, fearing they'll be replaced. I always emphasize that predictive maintenance enhances their capabilities rather than replacing their expertise. Involving maintenance staff early in the implementation process is crucial for success.
Integration Challenges
Legacy equipment often lacks built-in sensors or digital interfaces. However, modern retrofit solutions can work with almost any machine. I've helped companies instrument 40-year-old equipment with excellent results.
Looking Ahead
The future of asset management is even more exciting. Advanced AI models are becoming increasingly accurate at predicting failures and optimizing maintenance schedules. Digital twins allow us to simulate different maintenance strategies and their long-term impacts. And augmented reality tools are making it easier for maintenance teams to access real-time data and guidance.
But the core principle remains the same: proactive asset management is far more cost-effective than reactive maintenance. Every dollar invested in predictive maintenance typically returns $3-5 in reduced maintenance costs and avoided downtime.

Taking Action
If you're considering implementing predictive maintenance, here are my recommended first steps:
- Audit your current maintenance practices and costs
- Identify your most critical assets and their failure modes
- Start collecting basic condition monitoring data
- Partner with experts who can help you develop and implement a predictive strategy
Remember, the goal isn't to transform everything overnight. Start small, prove the concept, and scale based on results. The journey to predictive maintenance is a marathon, not a sprint.
After years of helping companies optimize their operations, I'm more convinced than ever that predictive asset management is a competitive necessity, not a luxury. The technology is mature, the costs are reasonable, and the benefits are clear.
The manufacturing leaders who embrace predictive maintenance now will have a significant advantage over those who wait. They'll operate more efficiently, deliver more reliably, and ultimately build stronger, more profitable businesses.
If you're ready to explore how predictive maintenance could benefit your operations, let's connect. Together, we can develop a strategy that fits your needs and budget while delivering real, measurable results.
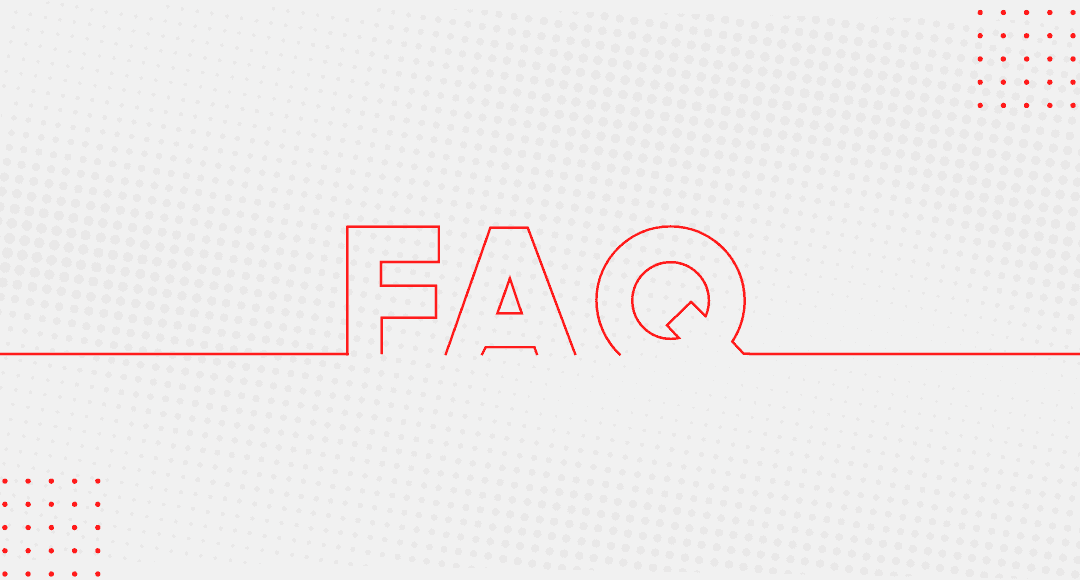
Q1: We're a small manufacturer with limited resources. How can we start implementing predictive maintenance without breaking the bank?
Start with a focused approach on your most critical equipment. You don't need to implement sensors and monitoring systems across your entire facility at once. Begin by identifying one piece of equipment where failures are particularly costly or disruptive. Many modern sensors are surprisingly affordable, often costing less than a single emergency repair. You can start with basic monitoring of temperature, vibration, or power consumption – whichever parameter best indicates your equipment's health. As you demonstrate ROI from this initial implementation, you can gradually expand to other assets.
Q2: Our maintenance team is skeptical about AI and predictive models. How can we get their buy-in?
This is a common and valid concern. The key is to position predictive maintenance as a tool that enhances, rather than replaces, your maintenance team's expertise. Start by involving your maintenance staff in the implementation process from day one. Let them help identify which parameters to monitor and what thresholds indicate potential problems. Their hands-on experience is invaluable in training and fine-tuning the predictive models. Show them how the technology can eliminate tedious tasks and help them focus on more skilled work. When they see how predictive insights make their jobs easier and more effective, skepticism typically turns into enthusiasm.
Q3: How accurate are predictive maintenance models? Can we really trust them to prevent failures?
Modern predictive maintenance models can achieve accuracy rates of 85-95% in identifying potential failures weeks in advance. However, it's important to understand that these models don't operate in isolation – they're most effective when combined with human expertise. The models flag potential issues, but your maintenance team's judgment remains crucial in interpreting these warnings and deciding on appropriate actions. Initially, run your predictive maintenance program alongside existing maintenance schedules until you've validated its reliability for your specific equipment and conditions.
Q4: We have older equipment without built-in sensors or digital interfaces. Can we still implement predictive maintenance?
Absolutely. Many of our most successful implementations have been with older equipment. External sensors can be retrofitted to almost any machine to monitor key parameters like vibration, temperature, or power consumption. In fact, older equipment often benefits more from predictive maintenance because it tends to have more predictable wear patterns and failure modes. We've successfully implemented monitoring systems on machinery from the 1980s and earlier. The key is choosing the right parameters to monitor and the appropriate sensor technology for your specific situation.
Q5: What kind of ROI can we expect, and how quickly will we see results?
Based on our experience with mid-sized manufacturers, a well-implemented predictive maintenance program typically delivers ROI of 3-5x within the first year. You'll likely see initial results within 3-6 months, starting with reduced emergency repairs and overtime costs. A typical implementation timeline shows 10-15% maintenance cost reduction in the first quarter, growing to 25-30% by the end of the first year. However, the full benefits, including extended equipment life and optimized maintenance scheduling, usually become apparent in the second year of implementation.
Q6: Our data quality isn't great. Should we wait to improve our data collection before starting with predictive maintenance?
While good data is important, waiting for perfect data often means missing out on significant benefits in the meantime. Start with whatever data you have, even if it's just maintenance logs and basic equipment readings. You can improve data quality incrementally as your program develops. Many organizations actually find that implementing predictive maintenance naturally leads to better data collection practices across their operations. The key is to begin with clear documentation of your current state and gradually build up your data infrastructure.
Q7: How do we handle the cybersecurity risks associated with connecting our equipment to monitoring systems?
Cybersecurity is a crucial consideration in any industrial IoT implementation. A properly designed predictive maintenance system can be implemented with robust security measures. This typically includes network segmentation, encrypted communications, secure authentication, and regular security updates. Many systems can operate on an isolated network, separate from your primary business systems. We recommend working with IT security professionals during implementation to ensure all security requirements are met while maintaining system effectiveness.
Q8: What happens if the predictive model misses a potential failure or gives a false alarm?
No predictive system is perfect, which is why we recommend a graduated implementation approach. Initially, run predictive maintenance alongside your existing maintenance procedures. This allows you to validate the model's accuracy while maintaining your safety net. False positives (false alarms) are typically more common than false negatives (missed failures) and can be tuned over time as the system learns from your specific operating conditions. The cost of investigating a false alarm is usually minimal compared to the cost of an unexpected failure.
Q9: We operate multiple shifts with different maintenance crews. How do we ensure consistent implementation across shifts?
Consistency across shifts requires a combination of standardized procedures and good communication tools. Start by creating clear, documented procedures for responding to predictive maintenance alerts. Implement a digital system for shift handovers that includes the status of all monitored equipment and any pending alerts. Regular cross-shift meetings can help ensure everyone understands the system and interprets alerts consistently. Many predictive maintenance platforms include built-in communication tools that help maintain consistency across shifts.
Q10: How do we measure success beyond just reduced downtime and maintenance costs?
While downtime reduction and cost savings are important metrics, a comprehensive evaluation should consider multiple factors. Track mean time between failures (MTBF), mean time to repair (MTTR), and overall equipment effectiveness (OEE). Look at quality metrics, as better-maintained equipment typically produces better output. Consider employee satisfaction – maintenance teams often report higher job satisfaction when they can prevent problems rather than constantly react to them. Monitor energy consumption, as well-maintained equipment typically operates more efficiently. Finally, track customer satisfaction metrics, as more reliable equipment usually leads to better on-time delivery performance and product quality.
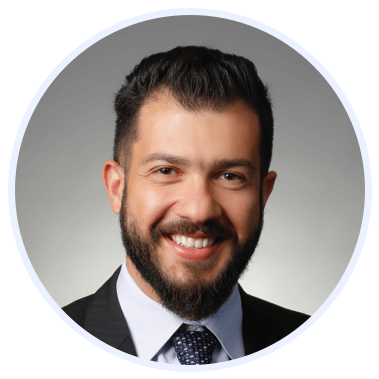
Rasheed Rabata
Is a solution and ROI-driven CTO, consultant, and system integrator with experience in deploying data integrations, Data Hubs, Master Data Management, Data Quality, and Data Warehousing solutions. He has a passion for solving complex data problems. His career experience showcases his drive to deliver software and timely solutions for business needs.