
AI is no longer just a buzzword. It's a powerful force driving real change across industries.
From cutting costs to improving efficiency, AI is transforming the way businesses operate. It’s about making smarter decisions faster and staying ahead in a competitive world.
Curious how it all works? Let’s see AI in action across various fields.
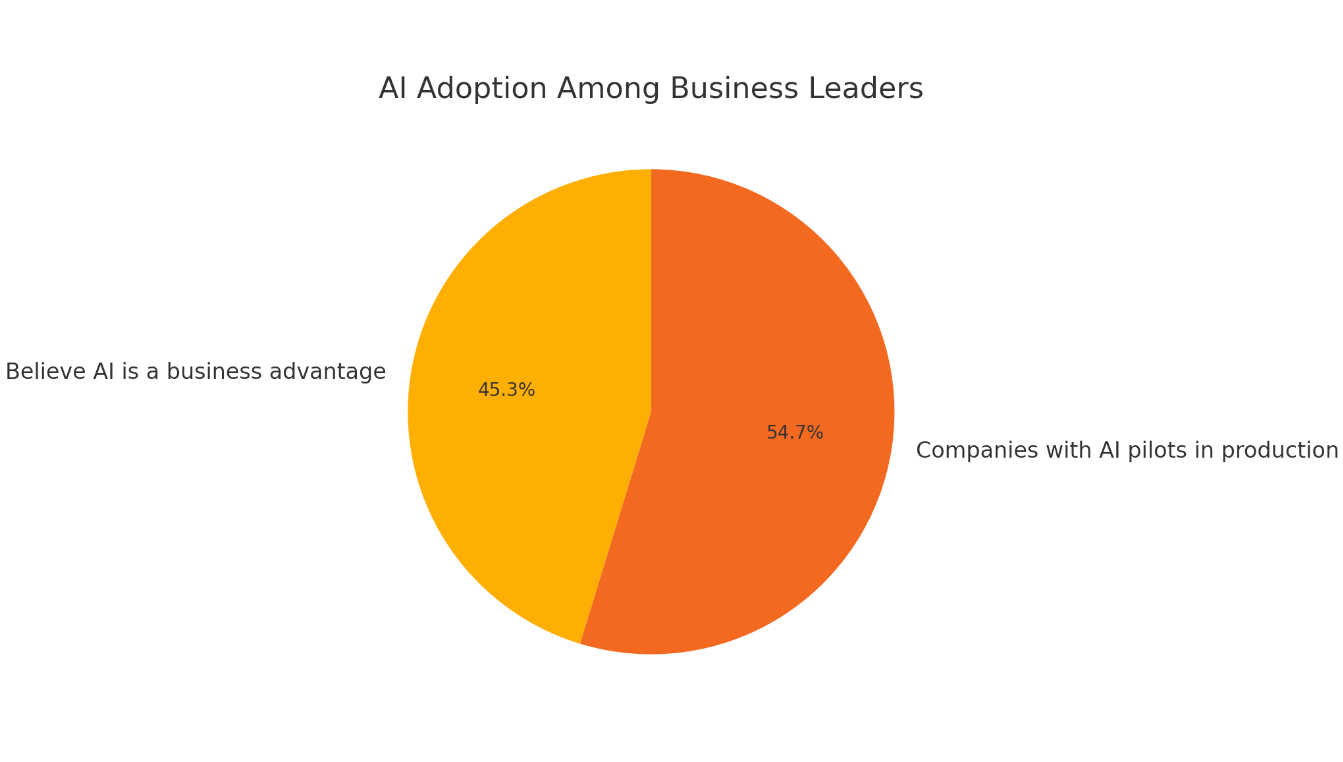
Healthcare: Revolutionizing Diagnosis and Treatment
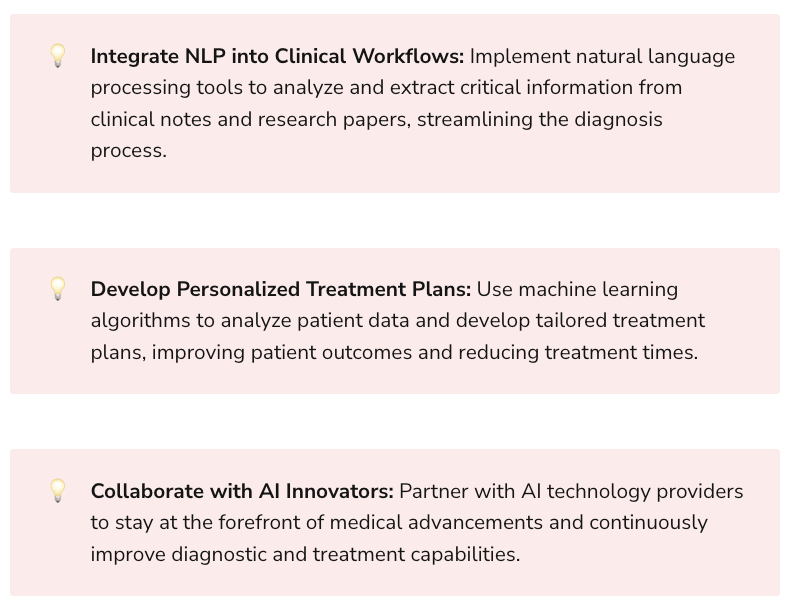
Case Study: IBM Watson Health
IBM Watson Health has been a pioneer in deploying AI to improve healthcare outcomes. One of the most notable implementations is its collaboration with Memorial Sloan Kettering Cancer Center (MSK). Watson's ability to process vast amounts of medical literature and patient data has been instrumental in aiding oncologists to diagnose and recommend treatment options.
Tactics:
- Natural Language Processing (NLP): Watson uses NLP to interpret clinical notes and research papers, extracting relevant information to support decision-making.
- Machine Learning Algorithms: These algorithms analyze patient data to identify patterns and suggest treatment protocols.
Impact:
- Reduced diagnosis time from weeks to hours.
- Increased accuracy in identifying cancer types and recommending personalized treatments.


Finance: Enhancing Risk Management and Fraud Detection
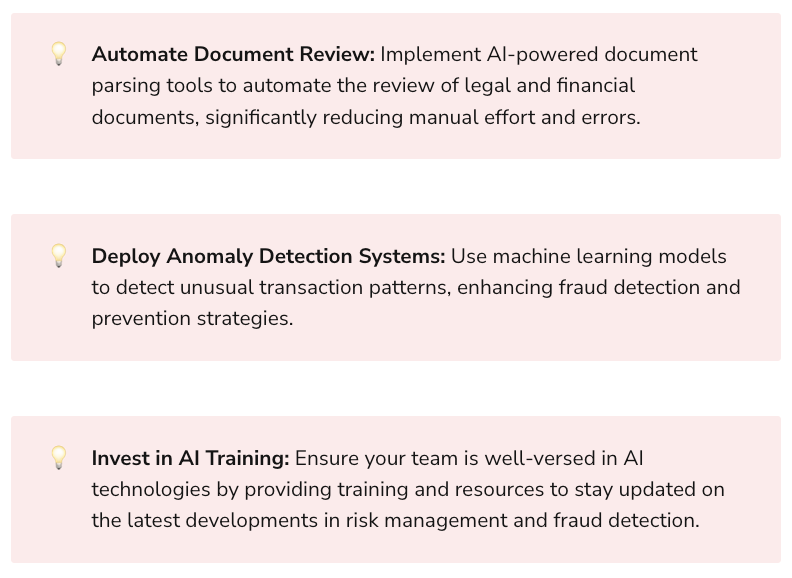
Case Study: JPMorgan Chase
JPMorgan Chase has implemented AI to enhance risk management and fraud detection processes. The bank's Contract Intelligence (COiN) platform uses machine learning to review legal documents and extract essential data points.
Tactics:
- Document Parsing: COiN parses through thousands of legal documents, identifying and classifying critical information.
- Anomaly Detection: Machine learning models detect unusual transaction patterns indicative of fraudulent activity.
Impact:
- Reduced time to review documents from 360,000 hours annually to seconds.
- Enhanced accuracy in identifying fraud, leading to a significant reduction in financial losses.

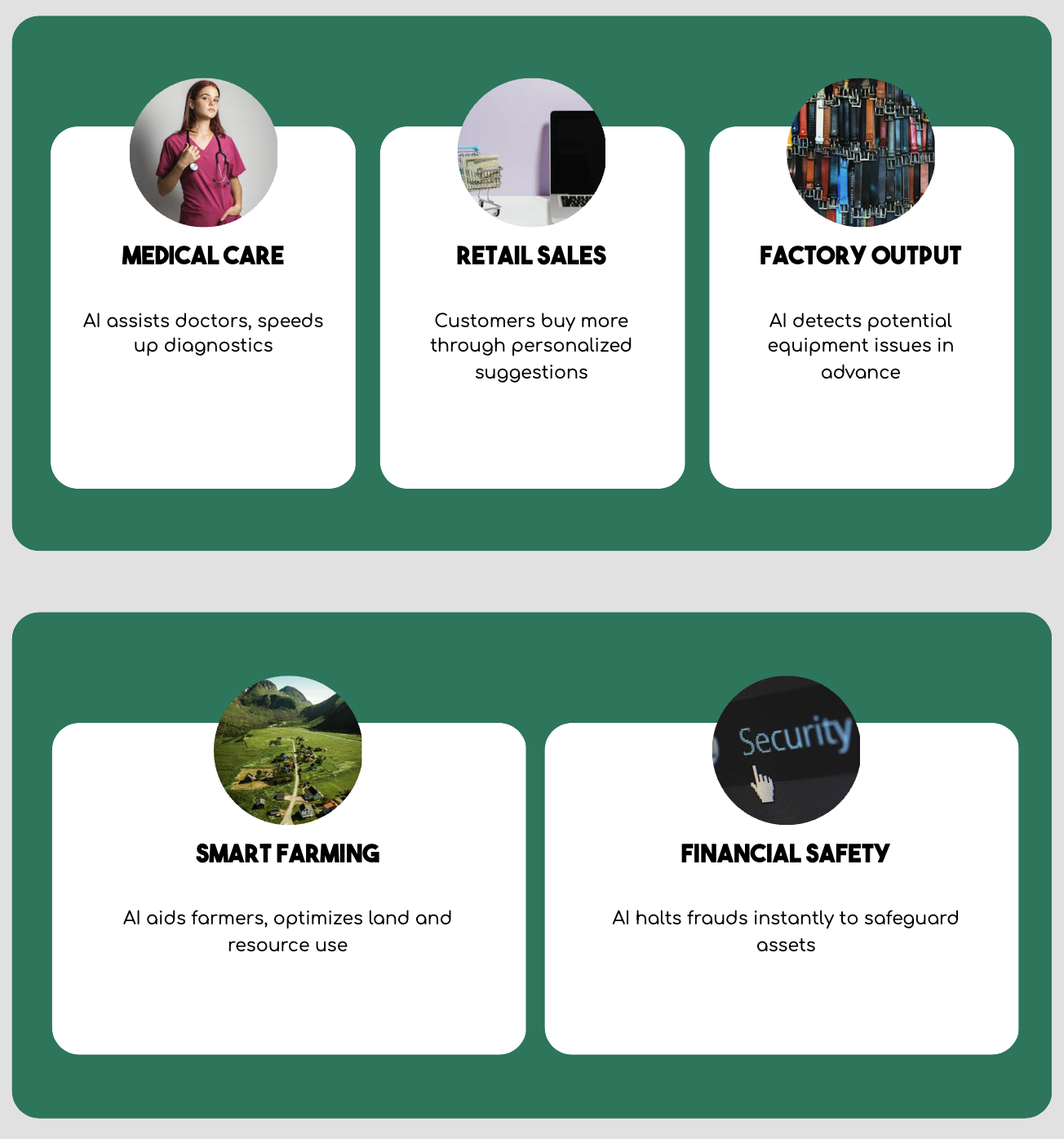
Manufacturing: Optimizing Production and Predictive Maintenance

Case Study: Siemens
Siemens has benefited from AI to optimize its manufacturing processes and predictive maintenance. By integrating AI with its production lines, Siemens has achieved significant improvements in efficiency and cost reduction.
Tactics:
- Predictive Maintenance: AI models predict equipment failures before they occur, allowing for proactive maintenance.
- Process Optimization: Machine learning algorithms analyze production data to identify inefficiencies and recommend process improvements.
Impact:
- Reduced unplanned downtime by up to 50%.
- Increased production efficiency by 20%.
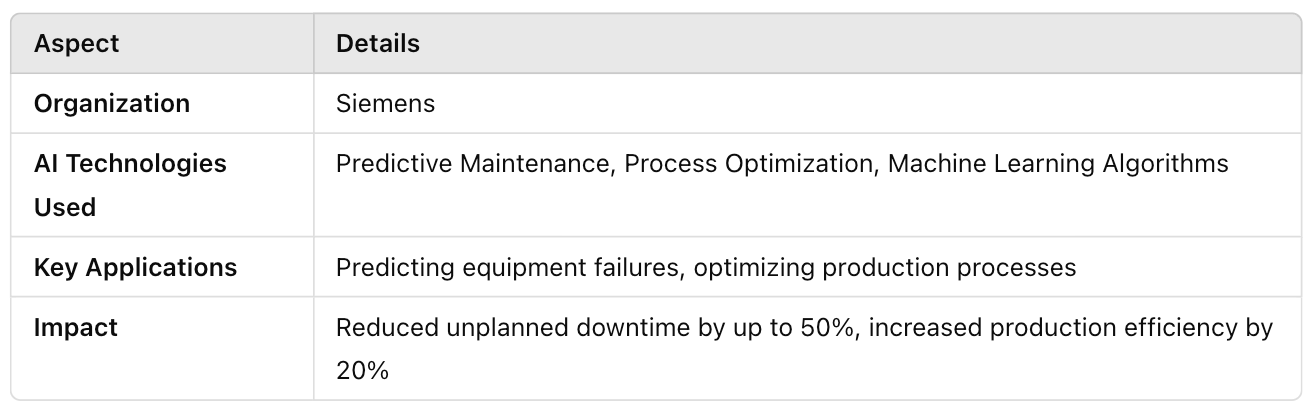
Retail: Personalizing Customer Experience and Inventory Management

Case Study: Amazon
Amazon's use of AI is a benchmark in the retail industry, particularly in personalizing customer experiences and optimizing inventory management. The company's recommendation engine and supply chain optimization algorithms are exemplary.
Tactics:
- Recommendation Systems: Collaborative filtering and deep learning techniques recommend products to users based on their browsing and purchase history.
- Inventory Optimization: AI models predict product demand, optimizing stock levels and reducing overstock and stockouts.
Impact:
- Increased sales through personalized recommendations, contributing to 35% of Amazon's revenue.
- Improved inventory turnover, reducing holding costs by 15%.

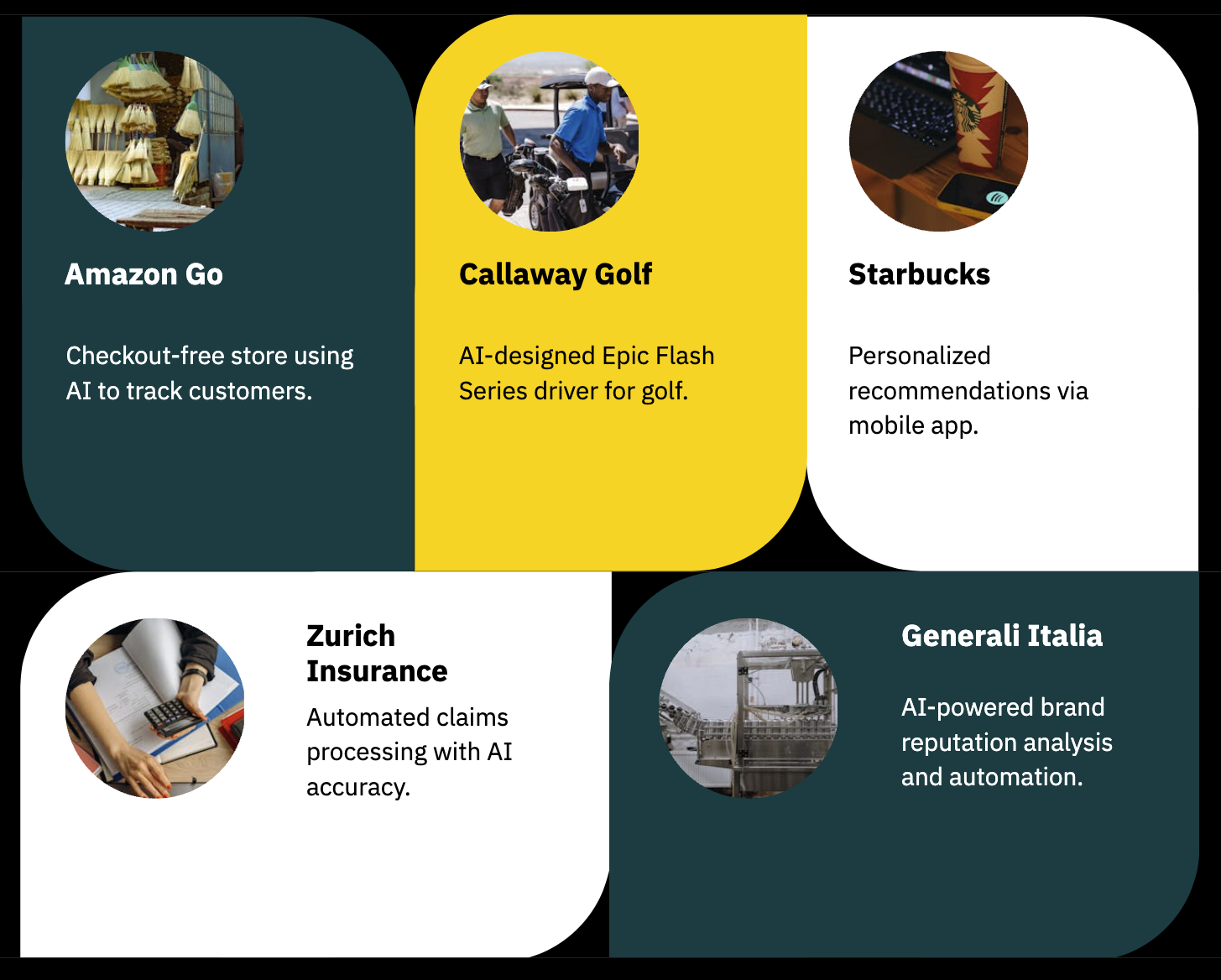
Transportation: Autonomous Vehicles and Fleet Management

Case Study: Tesla
Tesla's advancements in autonomous vehicle technology highlight the transformative potential of AI in the transportation industry. The company's AI-powered Autopilot and Full Self-Driving (FSD) systems are at the forefront of innovation.
Tactics:
- Computer Vision: AI models process camera inputs to understand the vehicle's surroundings and make real-time driving decisions.
- Reinforcement Learning: Self-driving algorithms are trained using reinforcement learning to improve driving performance over time.
Impact:
- Enhanced safety features, reducing the likelihood of accidents.
- Improved efficiency in fleet management, leading to cost savings and operational optimization.

Agriculture: Precision Farming and Crop Management
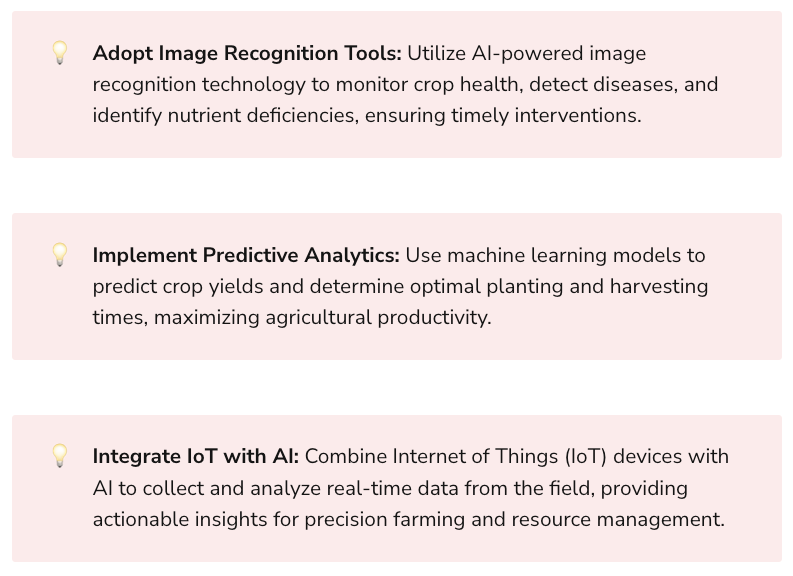
Case Study: John Deere
John Deere has integrated AI into its agricultural machinery to promote precision farming and improve crop management. The company's AI-powered equipment provides farmers with data-driven insights to optimize their operations.
Tactics:
- Image Recognition: AI models analyze images of crops to detect diseases, pests, and nutrient deficiencies.
- Predictive Analytics: Machine learning algorithms predict crop yields and suggest optimal planting and harvesting times.
Impact:
- Increased crop yields by 15% through precise monitoring and management.
- Reduced use of fertilizers and pesticides, leading to cost savings and environmental benefits.
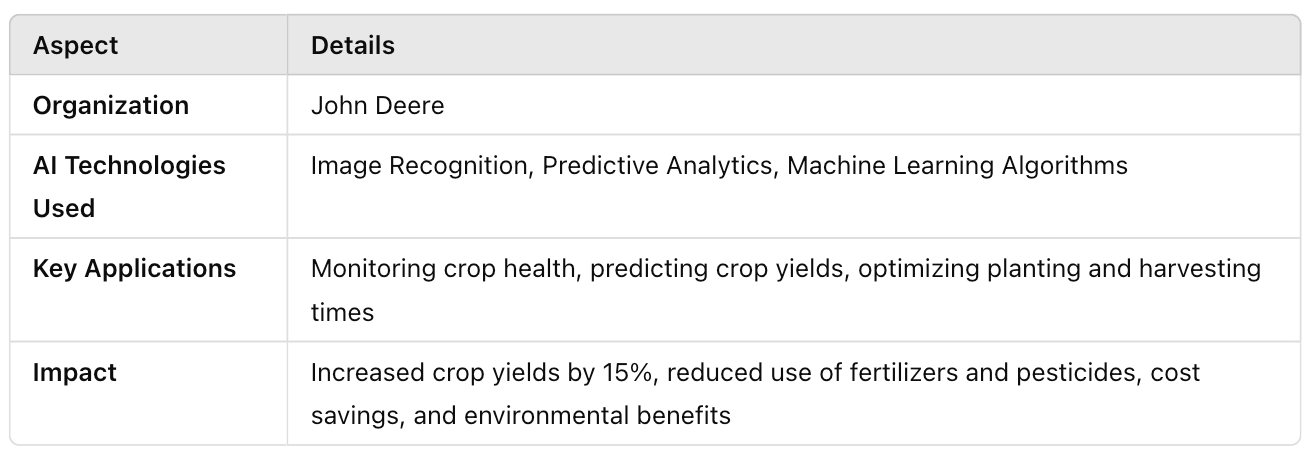

Conclusion
The successful implementation of AI across various industries underscores its transformative potential. These case studies highlight how AI can enhance operational efficiency, drive innovation, and provide a competitive edge. By engaging in tactics such as NLP, machine learning, and predictive analytics, organizations can harness the power of AI to solve complex problems and achieve remarkable outcomes. The future of AI promises even greater advancements, making it an essential tool for senior executives and decision-makers looking to stay ahead in an increasingly competitive landscape.
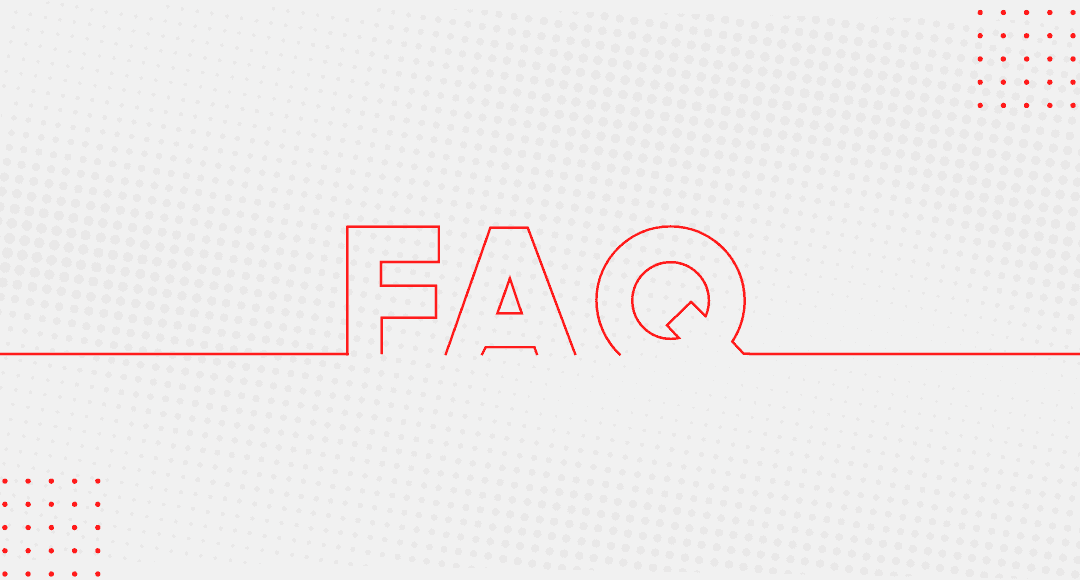
1. What are some real-world examples of AI in healthcare?
AI in healthcare includes IBM Watson Health's use in cancer diagnosis and treatment, where it processes vast amounts of medical literature and patient data to assist oncologists.
2. How is AI enhancing fraud detection in finance?
AI enhances fraud detection by using machine learning algorithms to analyze transaction patterns, detect anomalies, and identify potentially fraudulent activities more accurately than traditional methods.
3. What role does AI play in manufacturing?
In manufacturing, AI is used for predictive maintenance and process optimization. For example, Siemens employs AI to predict equipment failures and improve production efficiency, reducing downtime and costs.
4. How does AI improve the customer experience in retail?
AI improves the retail customer experience through personalized recommendations and optimized inventory management. Amazon's recommendation engine, which uses collaborative filtering and deep learning, significantly boosts sales and customer satisfaction.
5. What advancements has AI brought to transportation?
AI advancements in transportation include autonomous driving technologies and fleet management. Tesla's AI-powered Autopilot system uses computer vision and reinforcement learning to enhance driving safety and efficiency.
6. How is AI used in agriculture?
AI in agriculture is used for precision farming and crop management. John Deere integrates AI for image recognition to monitor crop health and predictive analytics to optimize planting and harvesting schedules.
7. What are the benefits of using AI for predictive maintenance?
Predictive maintenance with AI helps in predicting equipment failures before they occur, reducing unplanned downtime and maintenance costs, thereby increasing operational efficiency.
8. How does AI contribute to risk management in finance?
AI contributes to risk management by automating document reviews and identifying irregular transaction patterns, which enhances the accuracy and efficiency of compliance and fraud detection processes.
9. Can AI help with inventory management in retail?
Yes, AI helps with inventory management by predicting product demand, optimizing stock levels, and reducing holding costs. This ensures that products are available when needed without overstocking.
10. What is the impact of AI on production efficiency in manufacturing?
AI impacts production efficiency by analyzing production data to identify bottlenecks and recommend process improvements, leading to higher productivity and reduced operational costs.
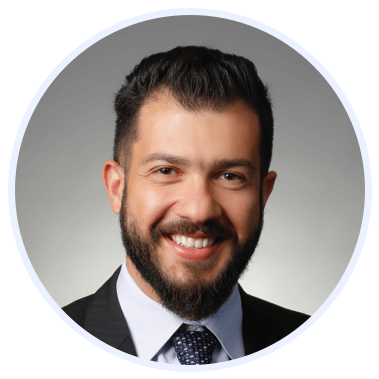
Rasheed Rabata
Is a solution and ROI-driven CTO, consultant, and system integrator with experience in deploying data integrations, Data Hubs, Master Data Management, Data Quality, and Data Warehousing solutions. He has a passion for solving complex data problems. His career experience showcases his drive to deliver software and timely solutions for business needs.