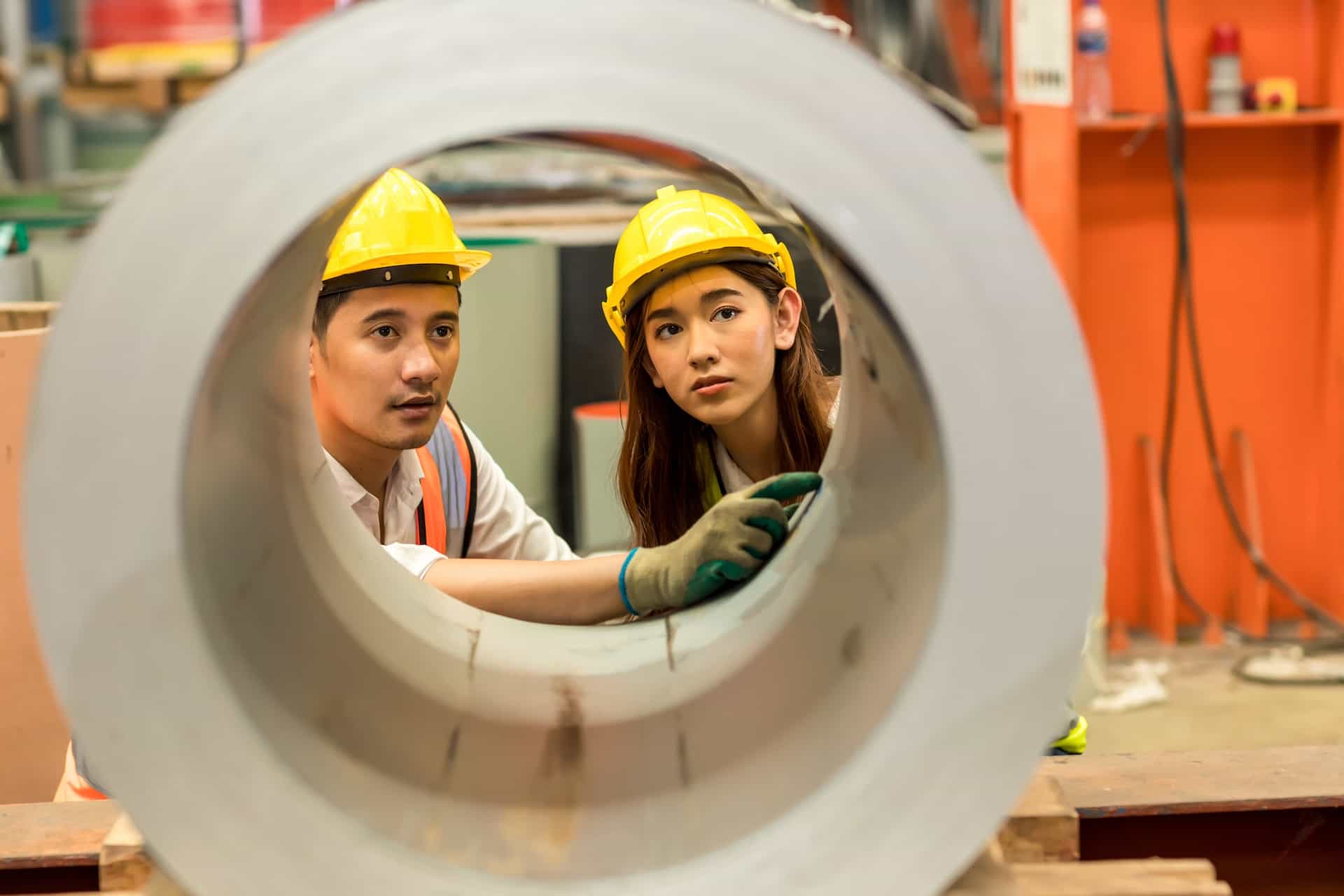
Continuous improvement isn’t just a buzzword for lean teams. It’s a survival strategy. For Operations Directors and lean teams navigating the challenges of scaling, the question isn’t whether to improve but how to sustain those improvements over time. This is where machine learning (ML) enters the scene, not as a futuristic concept, but as a practical ally.
From my work helping mid-sized businesses streamline operations, I've seen firsthand how ML can transform processes. By addressing inefficiencies, reducing costs, and driving sustainable growth, ML becomes more than a tool—it becomes a partner in continuous improvement.
Let’s explore how machine learning can support lean initiatives and unlock new levels of operational excellence.
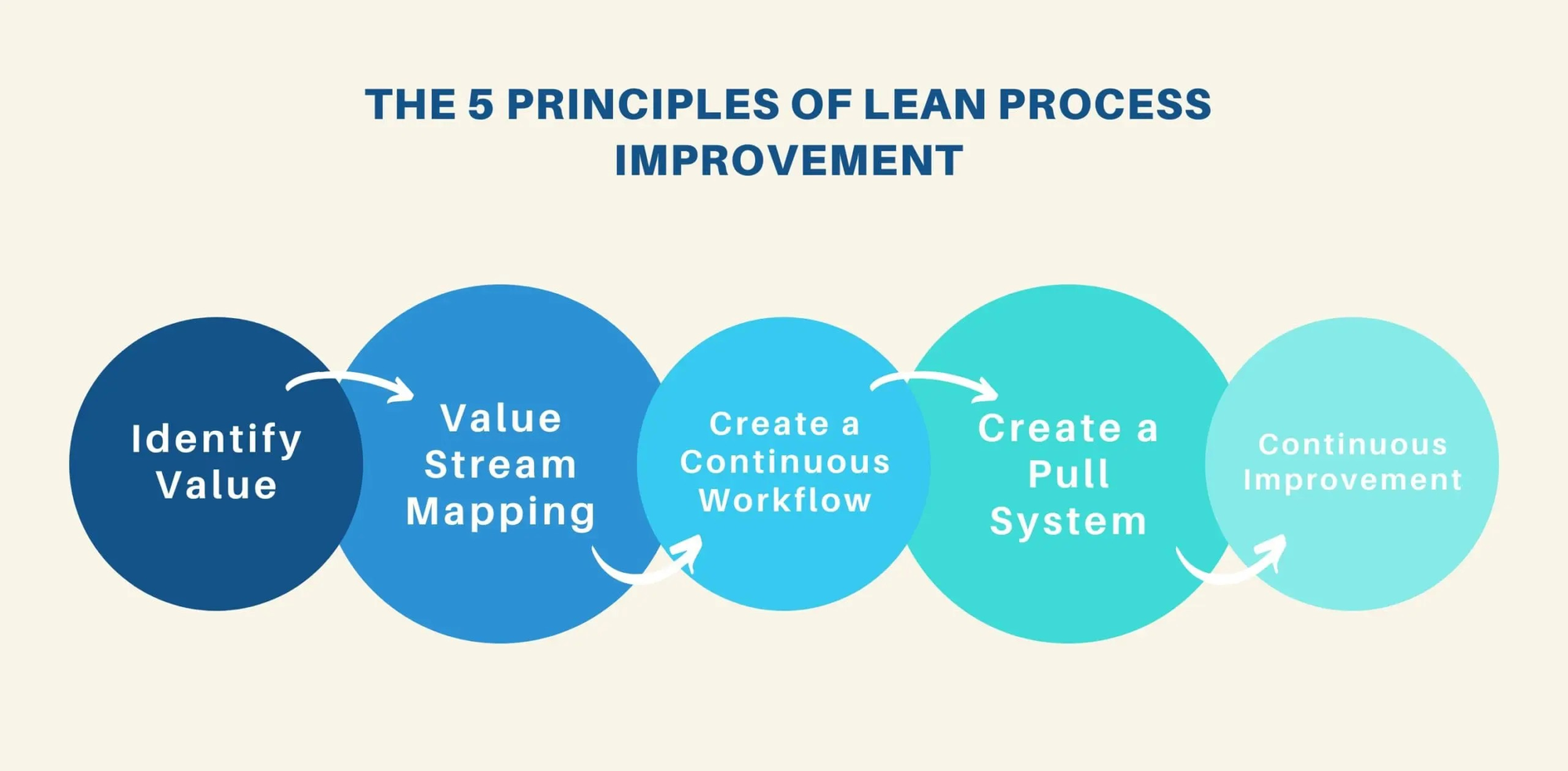
The Challenges of Scaling Lean Initiatives
Lean methodologies are built on principles of waste elimination, efficiency, and value creation. But scaling these principles isn’t always straightforward. As teams grow and processes become more complex, so do the challenges:
- Data Overload: Lean teams often generate enormous amounts of data, from production metrics to customer feedback. Sifting through this data to find actionable insights can feel like searching for a needle in a haystack.
- Sustaining Improvements: Initial lean efforts often yield quick wins. But sustaining and scaling those improvements across multiple teams or locations requires continuous monitoring and adaptation.
- Identifying Bottlenecks: In complex systems, pinpointing the root cause of inefficiencies isn’t always intuitive. Manual analysis can miss critical patterns.

These challenges aren’t insurmountable. With machine learning, they become opportunities for innovation.
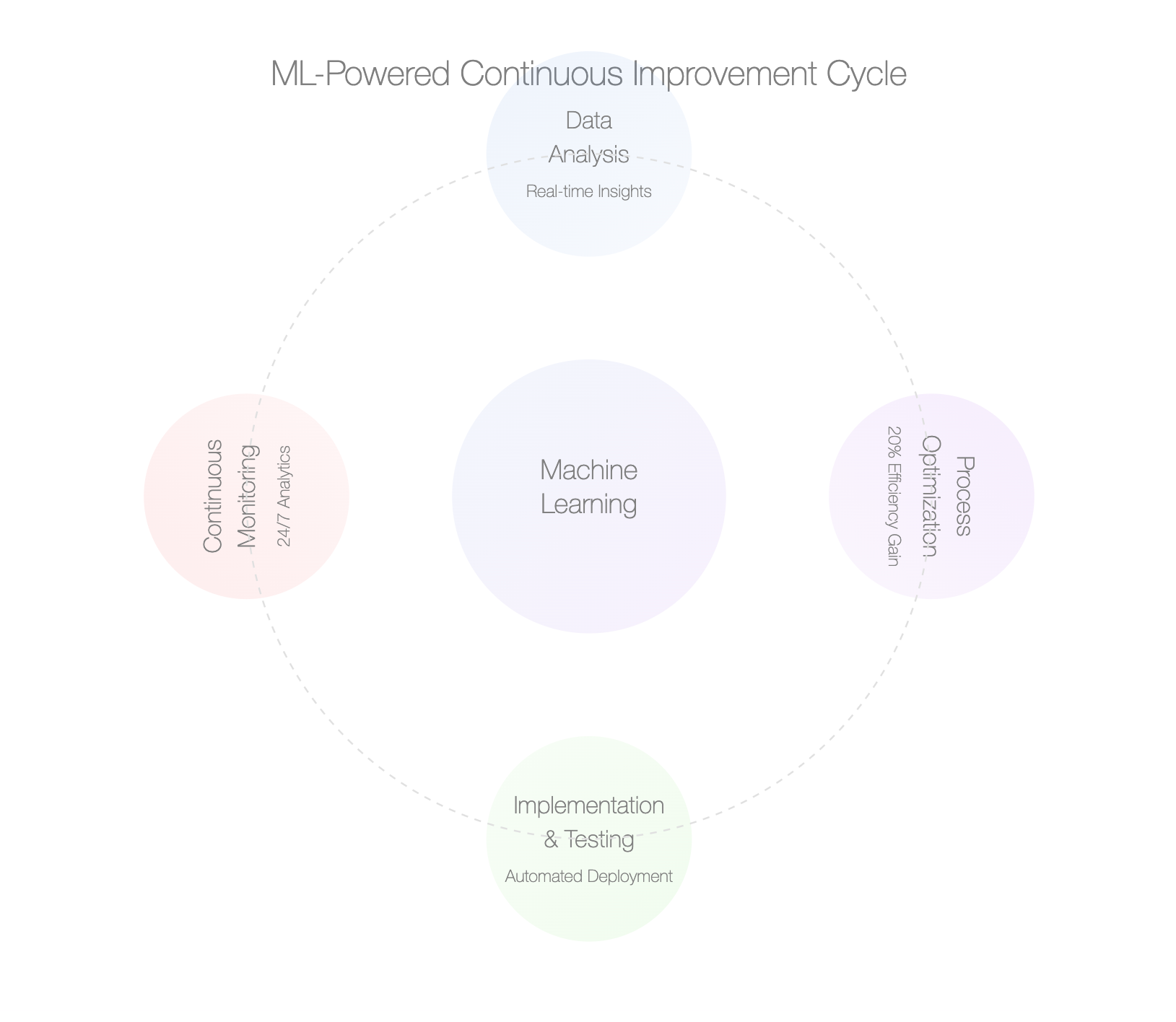
Machine Learning: A Catalyst for Lean Success
Machine learning excels at processing large datasets, identifying patterns, and making predictions. When applied to lean operations, it’s like having a virtual consultant that never sleeps.
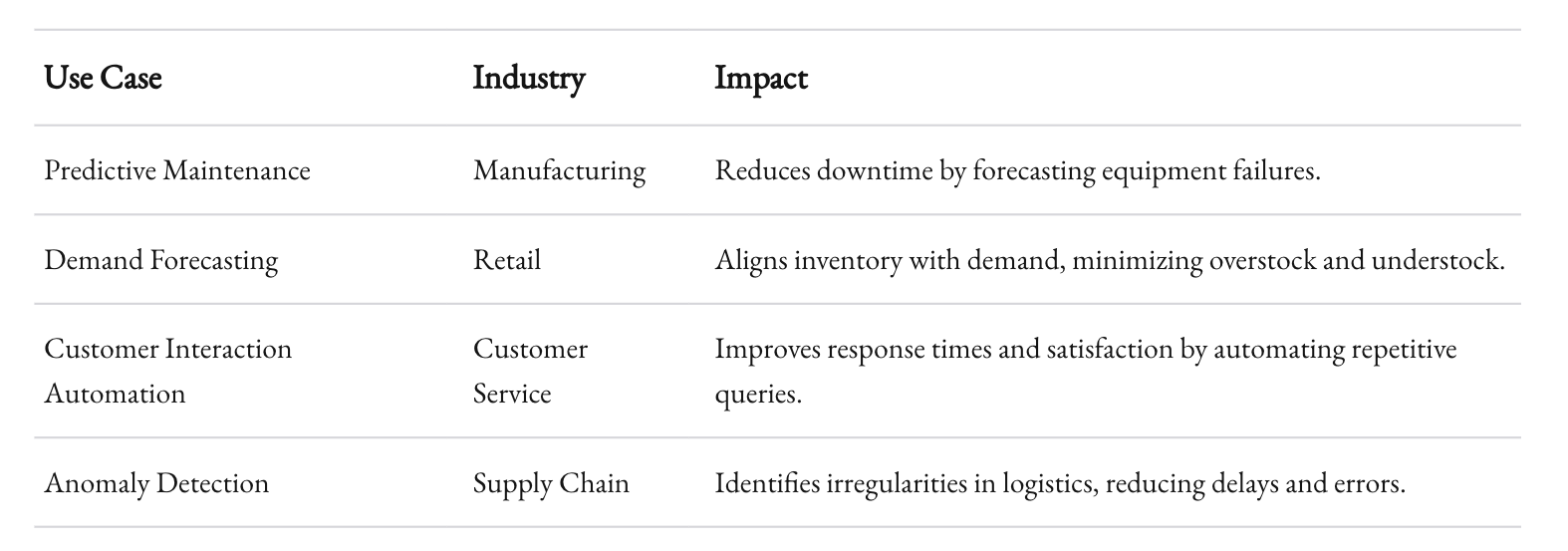
1. Turning Data into Insights
Lean teams thrive on data, but raw data alone isn’t enough. ML algorithms can:
- Automate Data Analysis: Instead of manually analyzing performance metrics, ML can surface insights automatically. For instance, anomaly detection algorithms can highlight unexpected deviations in production processes.
- Predict Trends: Predictive analytics can forecast demand, enabling teams to align resources more effectively.
- Drive Real-Time Decisions: Machine learning models can process data in real-time, empowering teams to respond instantly to changes in the system.
2. Sustaining Improvements
Continuous improvement demands ongoing vigilance. ML tools can:
- Monitor Performance: Dashboards powered by machine learning can provide a live view of operational health, flagging areas that need attention.
- Optimize Resource Allocation: ML models can recommend where to allocate resources for maximum impact, whether it’s labor, inventory, or machinery.
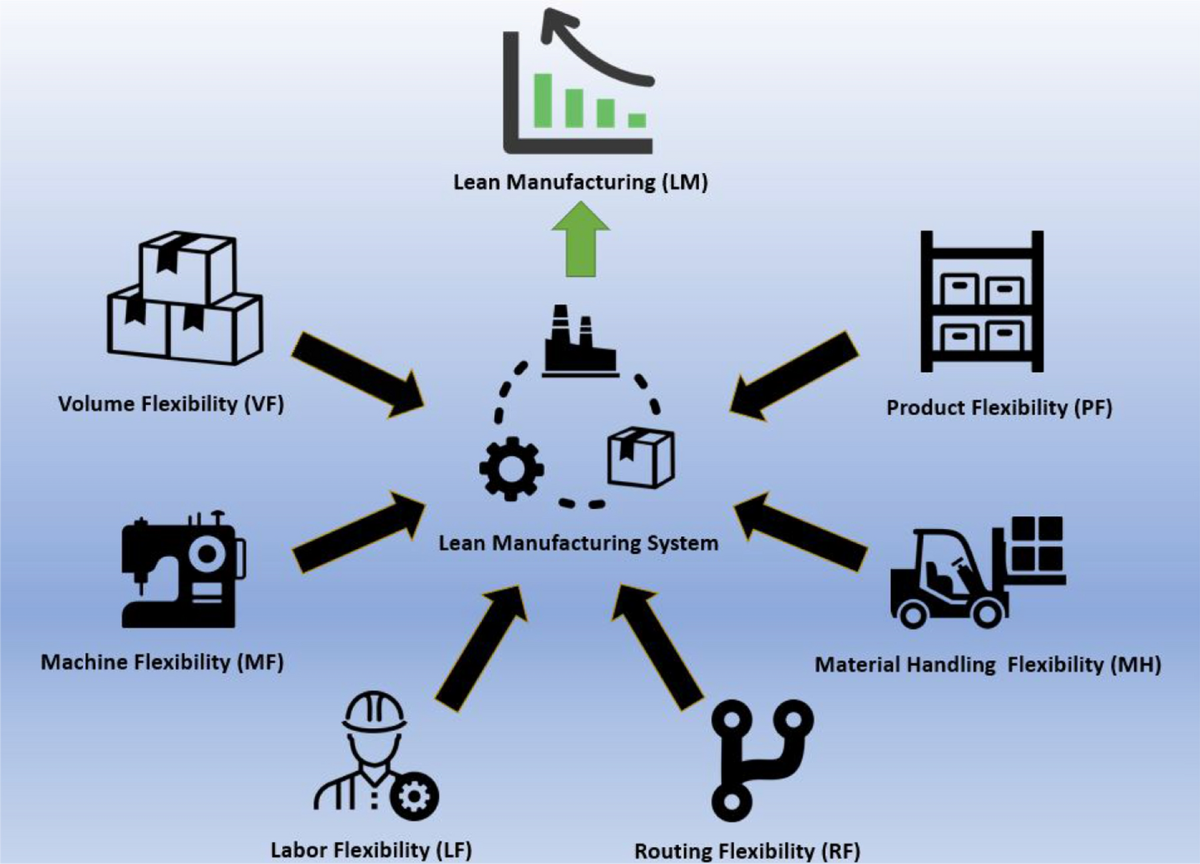
3. Finding Hidden Inefficiencies
Lean teams excel at identifying visible waste, but hidden inefficiencies often go unnoticed. ML thrives in this area:
- Identify Root Causes: By analyzing historical data, machine learning can uncover correlations and causations that humans might miss.
- Simulate Scenarios: Teams can use ML to test hypothetical changes in processes, predicting outcomes before implementing costly adjustments.
Real-World Applications
Let’s bring this to life with examples from industries embracing ML to enhance lean initiatives.
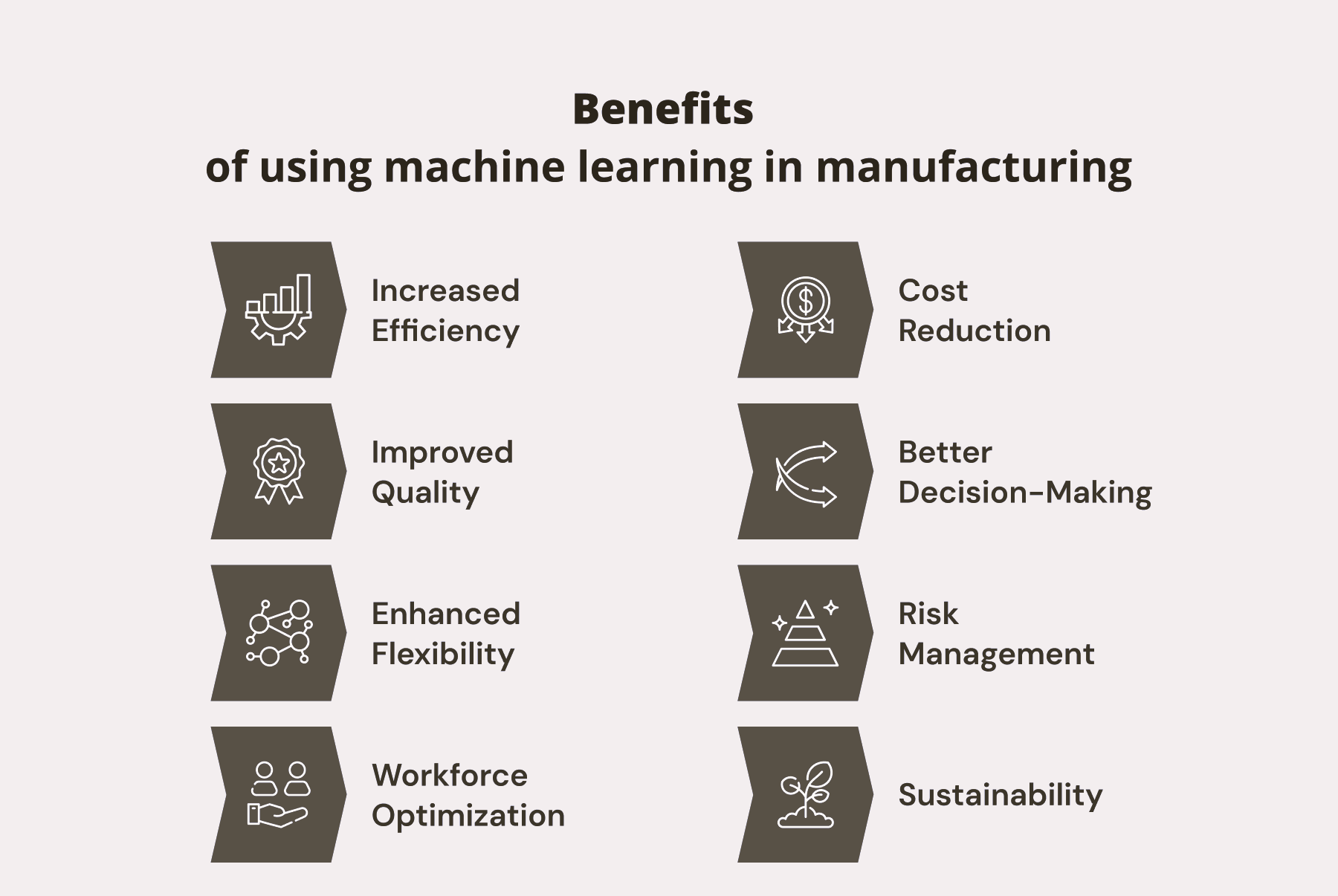
Manufacturing
A mid-sized manufacturer struggling with production delays used ML to analyze machine performance. The algorithm identified patterns in downtime, revealing a maintenance issue that traditional analysis had missed. By addressing this proactively, the company reduced downtime by 20%.
Supply Chain Optimization
A retailer with a complex supply chain used ML to predict inventory needs across its locations. The result? A 30% reduction in overstock and understock scenarios, saving millions in operational costs.
Customer Service
An organization implemented AI-driven chatbots powered by machine learning to handle repetitive customer queries. This not only improved response times but freed up human agents to focus on high-value interactions, boosting customer satisfaction scores by 40%.

Implementing ML for Lean Teams
Introducing machine learning into lean operations doesn’t require an overhaul. It starts with a strategy.

Step 1: Define Objectives
Identify the specific problems you’re trying to solve. Are you looking to reduce waste, enhance productivity, or improve customer satisfaction? Clear goals will guide your ML implementation.
Step 2: Start Small
Begin with pilot projects. Focus on a single process or team to test the waters. This minimizes risks and provides valuable insights before scaling.
Step 3: Build Cross-Functional Teams
Collaboration is key. Engage data scientists, operations managers, and front-line employees to ensure diverse perspectives and expertise.
Step 4: Choose the Right Tools
From open-source frameworks like TensorFlow to user-friendly platforms like DataRobot, selecting the right ML tools depends on your team’s technical capabilities and needs.
Step 5: Measure and Iterate
Continuous improvement applies to ML initiatives too. Monitor outcomes, gather feedback, and refine your models to ensure they’re delivering value.
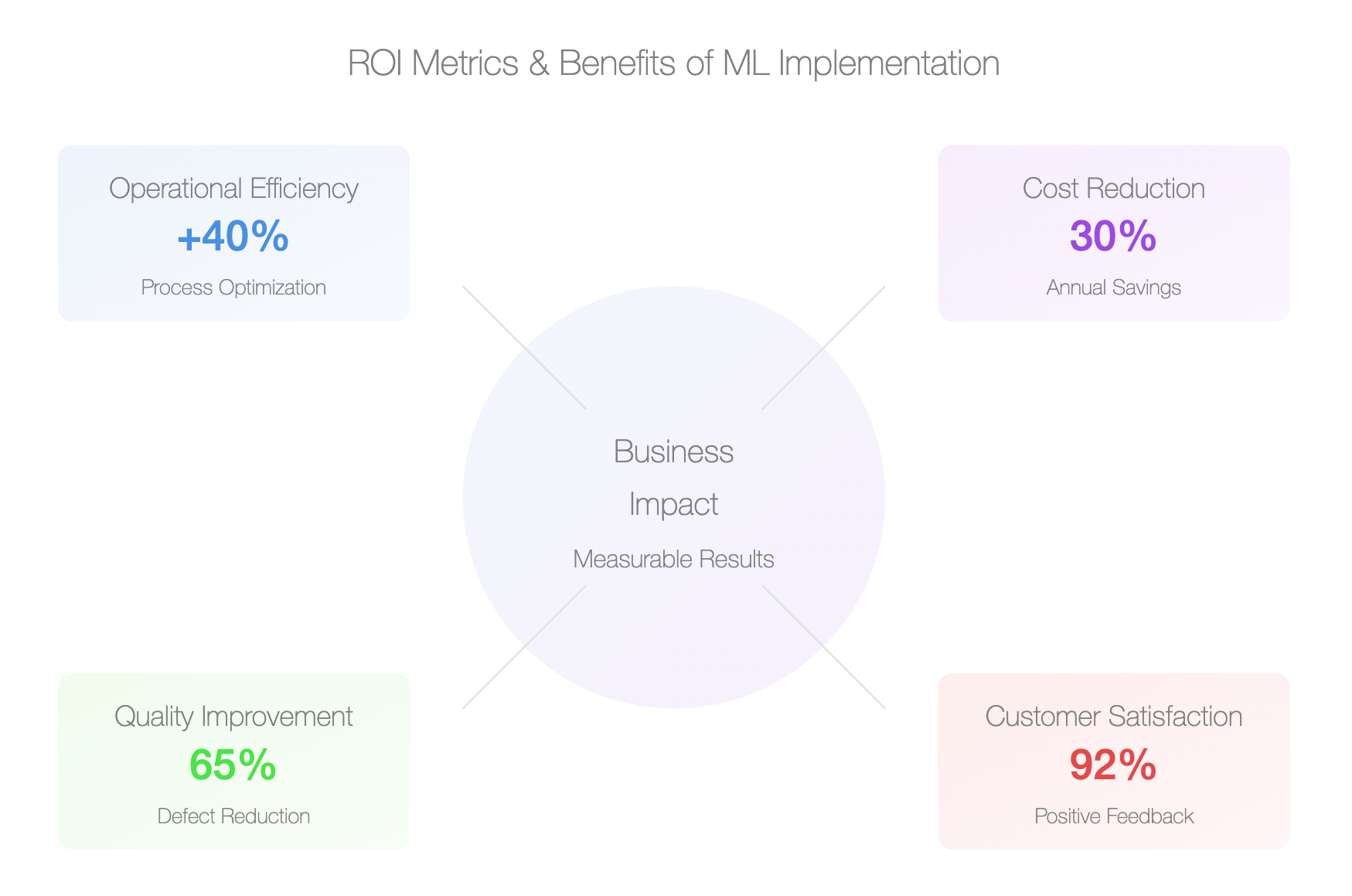
The ROI of Machine Learning
Investing in ML isn’t just about cutting-edge technology. It’s about measurable outcomes. Companies leveraging ML for lean operations often see:

These results aren’t theoretical. They’re achievable with the right approach.
Challenges to Consider
Of course, ML isn’t without its challenges. Teams must navigate:
- Data Quality: Poor data can lead to inaccurate models. Ensuring clean, reliable data is crucial.
- Change Management: Employees may resist new technologies. Strong leadership and training can ease the transition.
- Cost: While ML tools are becoming more accessible, there’s still an upfront investment in time and resources.
Addressing these challenges head-on ensures smoother adoption and better outcomes.
Recap
For lean teams and Operations Directors, sustaining and scaling improvements is a constant challenge. Machine learning offers a powerful way forward. By transforming data into insights, maintaining continuous improvement, and uncovering hidden inefficiencies, ML doesn’t just support lean initiatives—it amplifies them.
As someone who has seen the transformative impact of AI and ML on mid-sized businesses, I can confidently say the journey is worth it. The tools are ready, the opportunities are clear, and the potential for growth is immense.
Are you ready to take your lean initiatives to the next level with machine learning? Let’s make it happen.
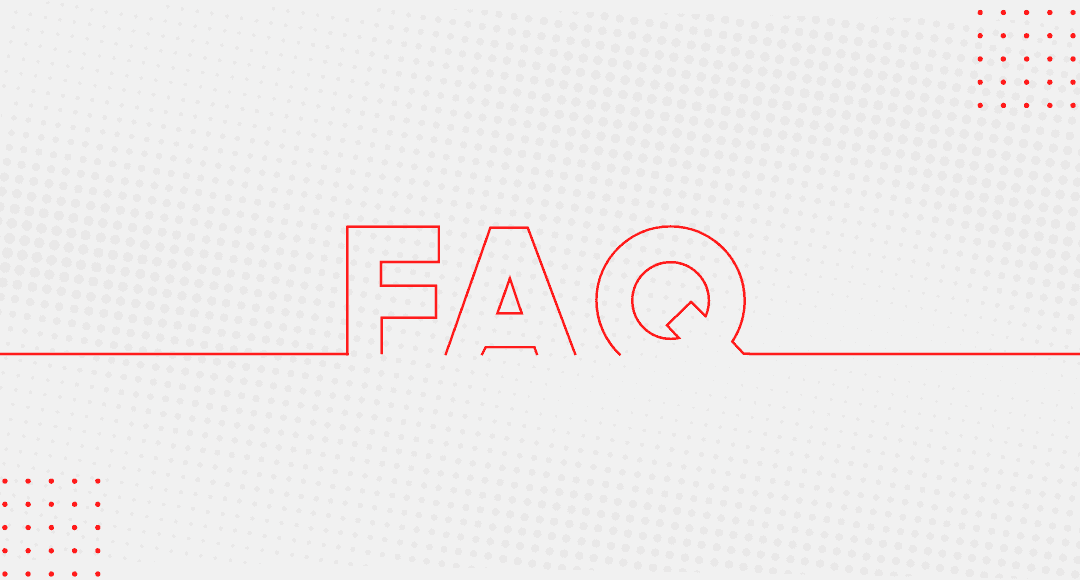
1. What is the role of machine learning in lean operations?
Machine learning (ML) enhances lean operations by automating data analysis, identifying inefficiencies, and predicting trends. It acts as a continuous monitoring tool, enabling teams to maintain and scale improvements effectively.
2. How can ML help sustain continuous improvement efforts?
ML supports continuous improvement by providing real-time insights and predictive capabilities. It identifies potential issues before they escalate, optimizes resource allocation, and ensures improvements are consistently monitored and adapted over time.
3. Is ML only useful for large-scale operations, or can smaller teams benefit too?
ML benefits both large and small teams. For smaller teams, lightweight tools and focused pilot projects can address specific challenges without overwhelming resources. Scalability makes ML adaptable to operations of any size.
4. What kind of data is required for machine learning in lean operations?
High-quality, structured data is essential for effective ML applications. This includes historical performance data, customer feedback, production metrics, and supply chain details. Clean, consistent data improves model accuracy and insights.
5. How expensive is it to integrate machine learning into lean operations?
The cost varies based on complexity, tools, and team expertise. Open-source frameworks like TensorFlow are free but require technical skills, while automated platforms like DataRobot may have subscription costs but simplify deployment.
6. What are some common challenges when adopting machine learning?
Challenges include ensuring data quality, managing employee resistance, integrating ML with existing systems, and the initial learning curve for teams unfamiliar with AI technologies. Addressing these proactively can smooth adoption.
7. How do I measure the success of ML in lean initiatives?
Success is measured by tangible metrics like productivity increases, cost reductions, improved customer satisfaction, and faster decision-making. Define clear objectives upfront and track them against these metrics.
8. Can machine learning replace human judgment in lean operations?
ML complements human judgment rather than replacing it. While it excels at identifying patterns and automating repetitive tasks, strategic decisions and nuanced problem-solving still rely on human expertise.
9. What industries can benefit most from combining lean and machine learning?
Industries with complex processes and high data volume benefit significantly. These include manufacturing, retail, logistics, healthcare, and customer service, where ML enhances operational efficiency and customer experience.
10. How long does it take to see results from ML implementation?
Timelines depend on the scope and complexity of the implementation. Pilot projects can show results in weeks, while broader deployments may take months. Consistent monitoring and iteration accelerate measurable outcomes.
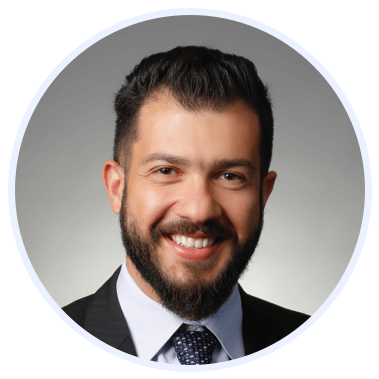
Rasheed Rabata
Is a solution and ROI-driven CTO, consultant, and system integrator with experience in deploying data integrations, Data Hubs, Master Data Management, Data Quality, and Data Warehousing solutions. He has a passion for solving complex data problems. His career experience showcases his drive to deliver software and timely solutions for business needs.