
Managing a product’s lifecycle has always been complex. From ideation to development, manufacturing, and eventually to the market—every phase is critical. But what if I told you there’s a way to revolutionize this process, making it more streamlined and insightful?
That’s where data-first product lifecycle management (PLM) comes in. It’s not just a buzzword; it’s a strategy that transforms how manufacturers operate. By leveraging data at every stage, you can unlock efficiencies, reduce costs, and create a feedback loop that fuels continuous improvement. Let me walk you through this transformative approach.
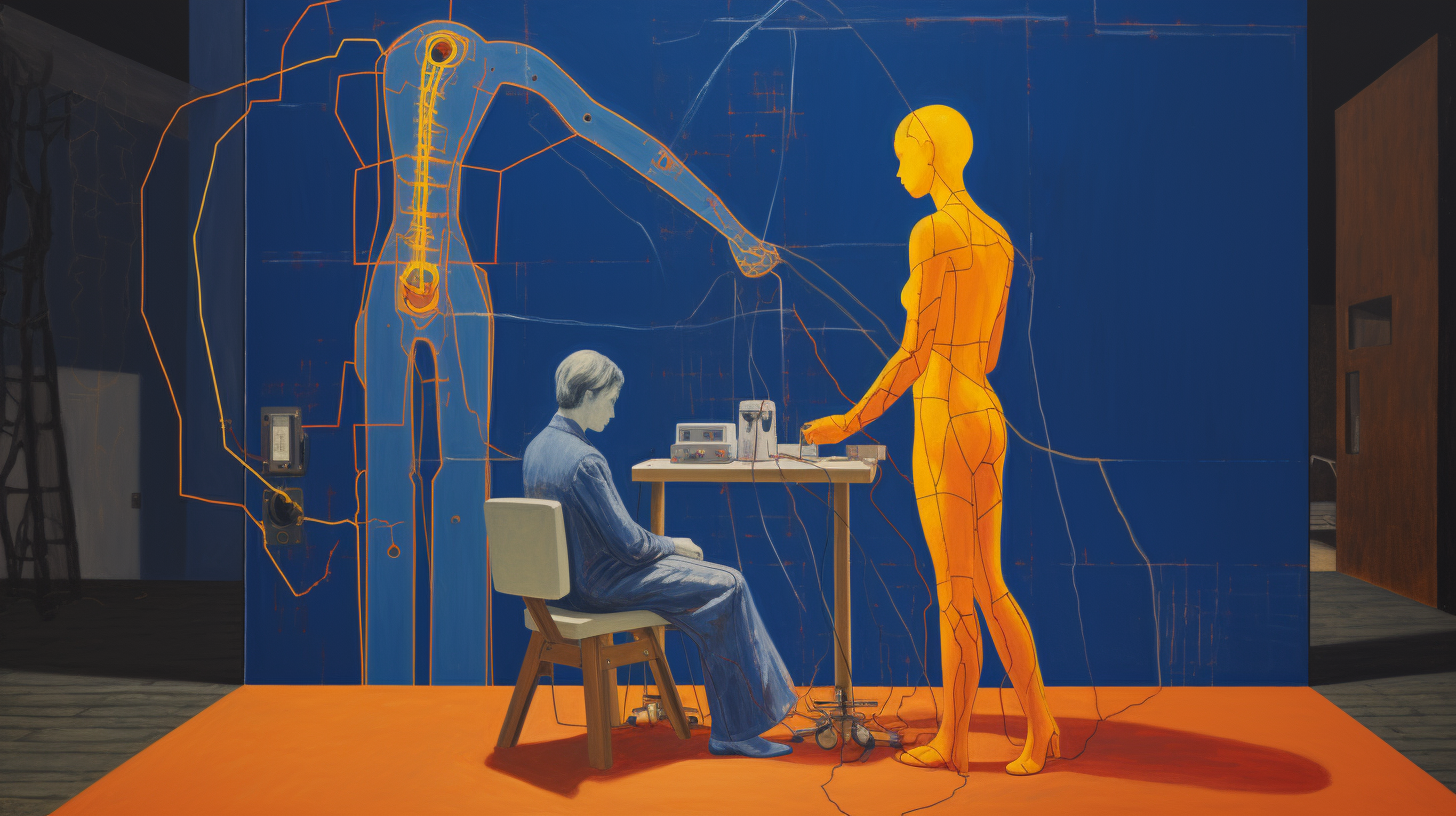
A Lifecycle Powered by Data
Ideation
Every great product starts with an idea. But in a data-first PLM model, these ideas don’t emerge in a vacuum. They’re shaped by insights derived from market trends, customer feedback, and even competitor analysis. AI tools play a pivotal role here. They sift through vast datasets to identify unmet needs, forecast future demand, and inspire innovative concepts.
Take an example: A company wants to develop a sustainable alternative to single-use plastics. By analyzing consumer sentiment and industry regulations, they can pinpoint the most viable materials and features before committing resources. This data-driven approach minimizes risks and aligns the product vision with market realities.

Design and Development
In the traditional model, design and development often operate in silos. But with a data-first approach, integration becomes seamless. Engineers and designers collaborate on platforms that aggregate data from various sources, including simulation software, material databases, and past project outcomes.

AI-powered simulations enable rapid prototyping without the need for costly physical models. Design flaws are identified early, saving both time and money. Additionally, predictive analytics guide material selection and production processes, ensuring that the final design is both functional and cost-efficient.
The result? Products that hit the sweet spot between innovation and feasibility.

Manufacturing With Precision
Manufacturing is where the rubber meets the road. In a data-first PLM framework, this stage benefits from real-time monitoring and predictive maintenance. Sensors installed on production lines feed data into AI systems that detect anomalies before they escalate into costly downtime.
Here’s a real-world scenario: A bottling company uses IoT-enabled sensors to monitor machinery. When a slight deviation in temperature is detected, the system flags it, prompting preemptive maintenance. This prevents an equipment failure that could halt production for days.
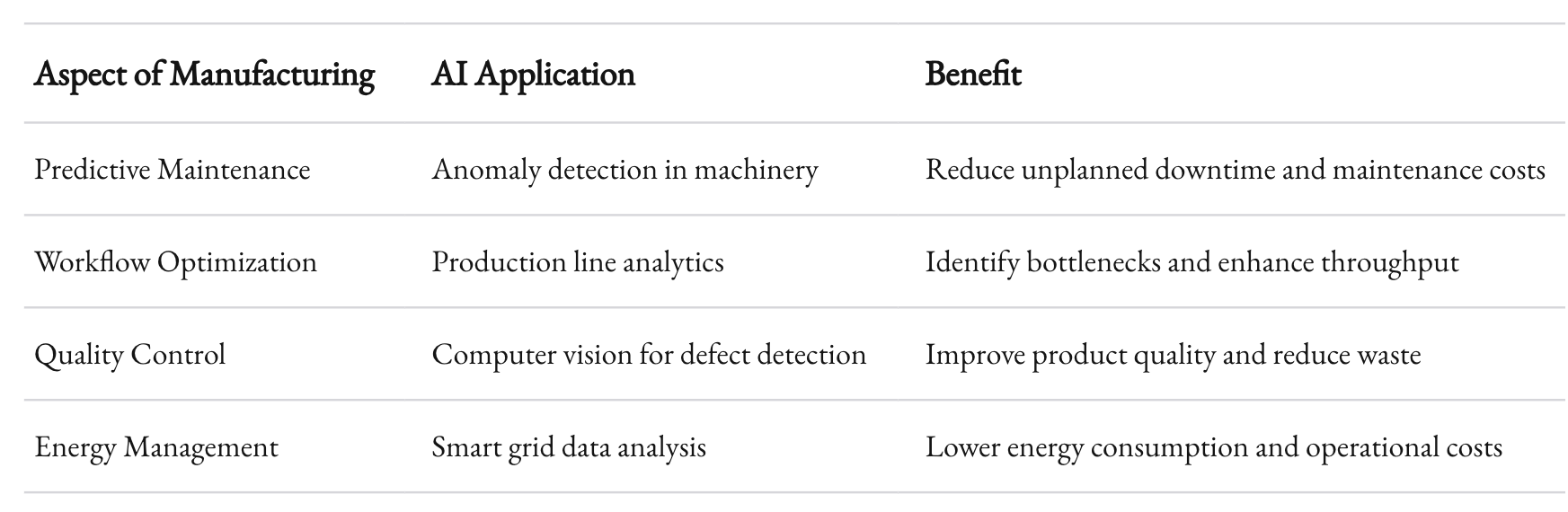
Moreover, data-first manufacturing emphasizes efficiency. By analyzing production data, companies can identify bottlenecks, optimize workflows, and even reduce energy consumption—all while maintaining high-quality standards.
Distribution and Customer Feedback
Once the product is out the door, the data journey doesn’t end. Distribution logistics benefit from AI-driven route optimization, ensuring timely deliveries while reducing fuel costs. But perhaps more importantly, customer feedback becomes a treasure trove of actionable insights.
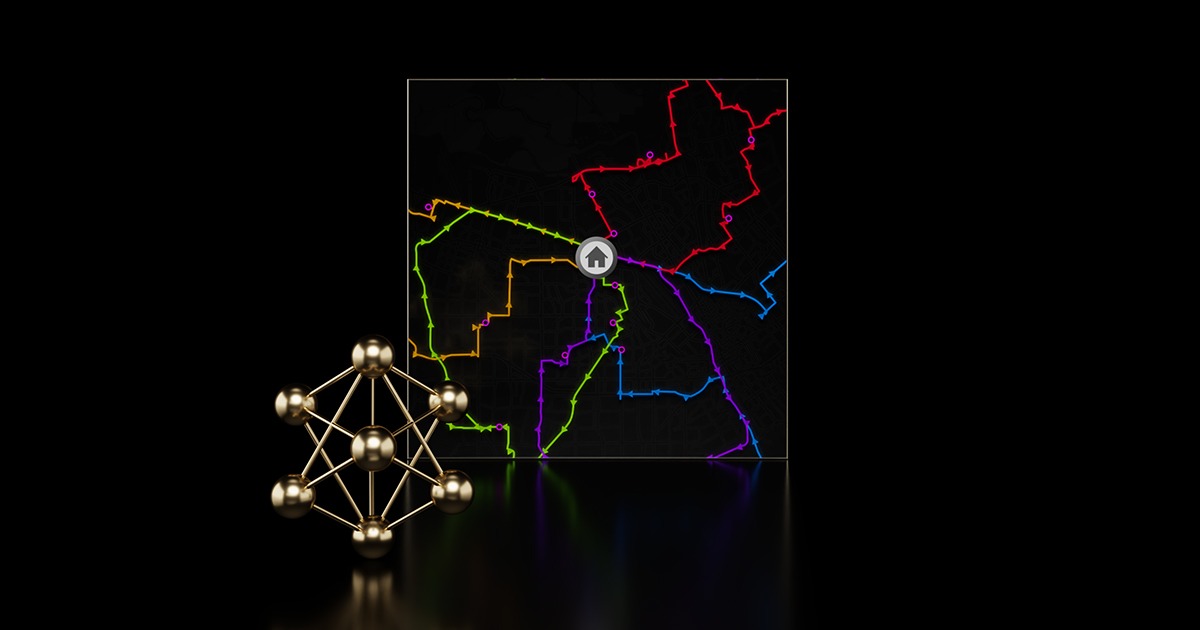
Social media sentiment analysis, product reviews, and direct customer feedback are just the tip of the iceberg. These data points feed back into the PLM system, informing updates, marketing strategies, and even future product iterations.
Consider this: A company launches a smart thermostat. Initial feedback highlights a user interface issue. Instead of waiting for the next product cycle, developers push a software update that resolves the problem. This agility not only improves customer satisfaction but also reinforces brand loyalty.

Continuous Improvement
The hallmark of a data-first PLM system is its ability to foster continuous improvement. By maintaining a feedback loop across the entire lifecycle, businesses can evolve their products and processes dynamically.
For instance, post-market data might reveal that a product performs better in certain conditions than initially tested. These insights can lead to targeted marketing campaigns or even the development of complementary products. It’s a virtuous cycle where data informs decisions, and decisions generate more data.

Challenges and How to Overcome Them
Adopting a data-first approach isn’t without hurdles. The most common challenges include:
- Data Silos: Disparate systems that don’t communicate.
- Resistance to Change: Teams accustomed to traditional workflows may be hesitant.
- Data Quality: Poor data can lead to flawed insights.
The solution lies in fostering a culture of collaboration and investing in unified platforms. Training programs can help teams adapt, while robust data governance ensures accuracy and reliability.
Why It Matters
In an era where margins are thin and customer expectations are sky-high, the traditional methods of managing a product lifecycle fall short. A data-first PLM approach not only addresses these challenges but also positions businesses for sustainable growth.
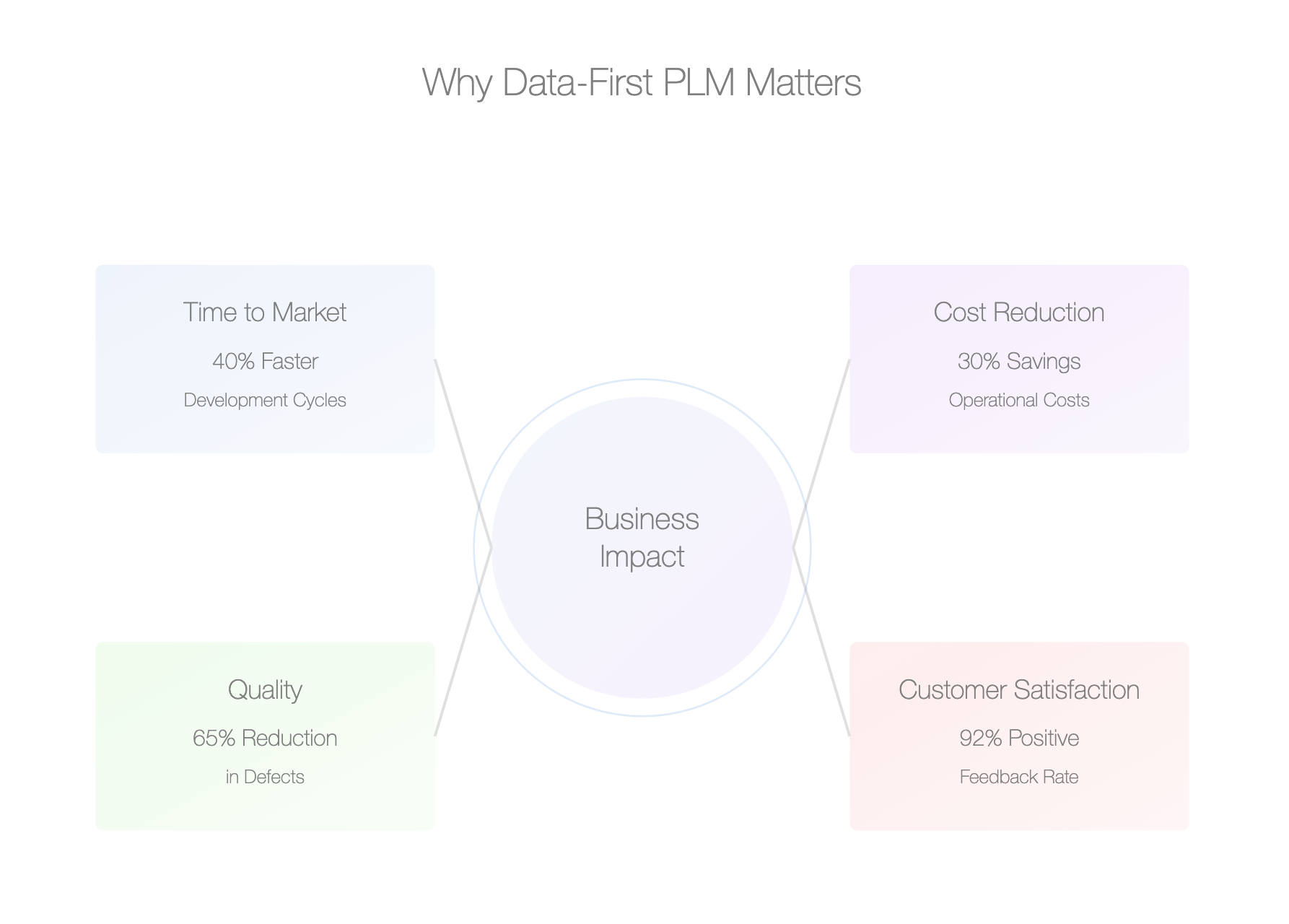
By leveraging insights from ideation to end-of-line, manufacturers can:
- Accelerate time-to-market.
- Reduce costs across the board.
- Enhance product quality and customer satisfaction.
This isn’t just about staying competitive; it’s about leading the charge.
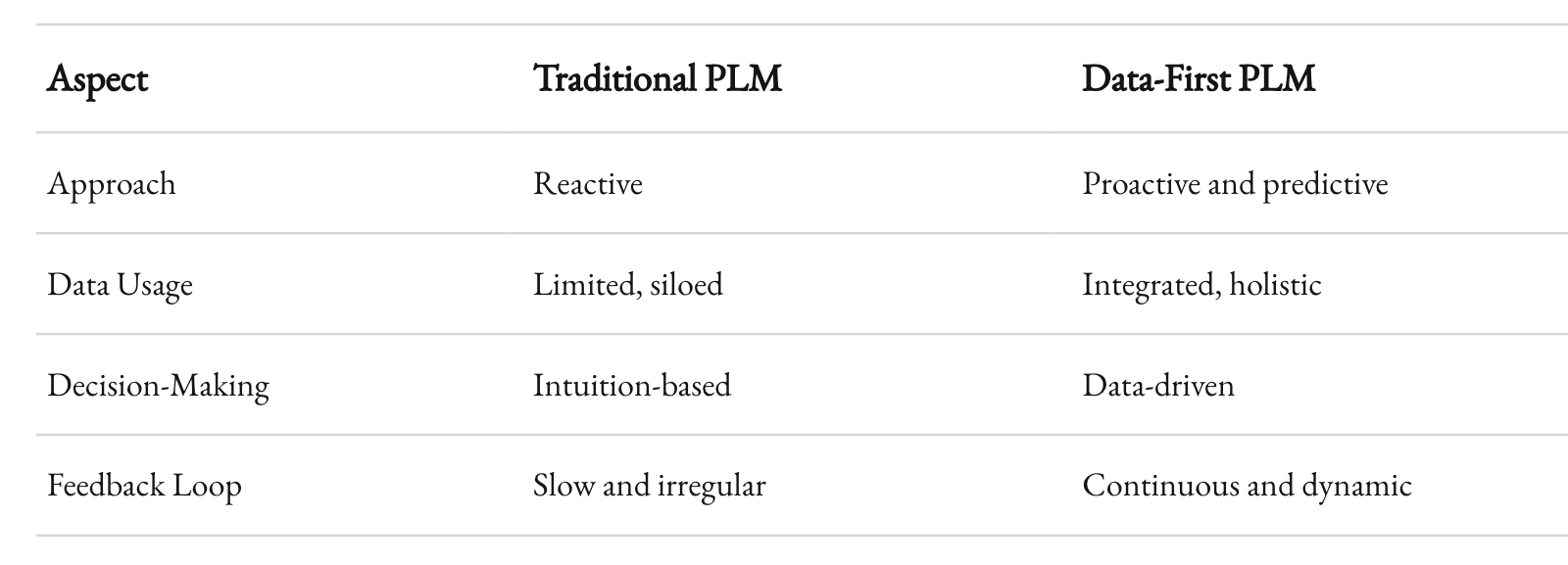
The Path Forward
Transitioning to a data-first PLM framework requires commitment, but the rewards far outweigh the investment. Begin with a pilot project to demonstrate value, then scale gradually. Leverage AI tools, cloud platforms, and IoT technologies to build a robust ecosystem.
The future of manufacturing isn’t just automated—it’s intelligent, interconnected, and driven by data. By adopting a data-first approach, you’re not just improving operations; you’re redefining what’s possible.
Let’s make it happen.
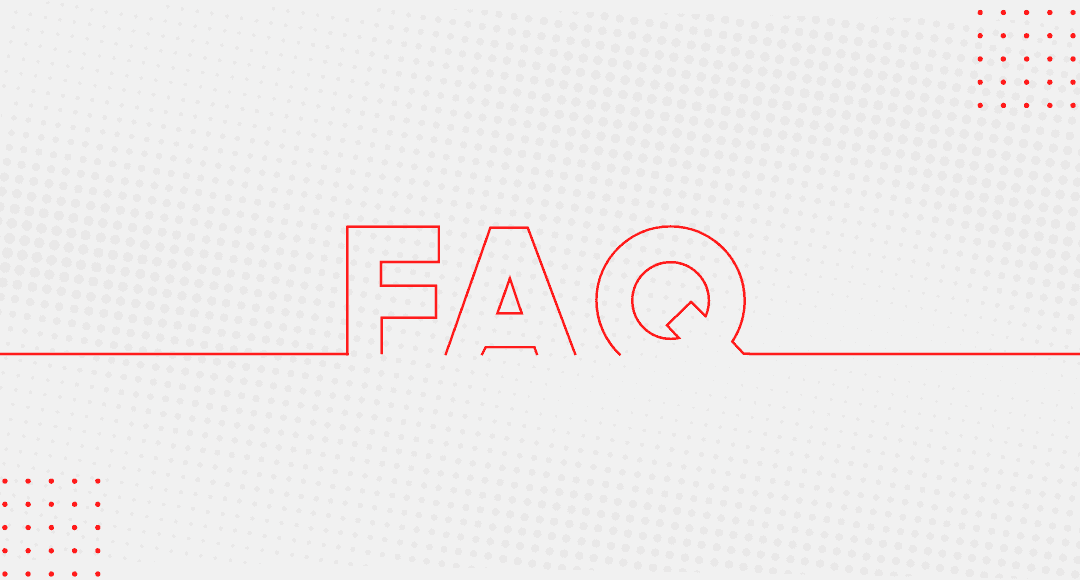
1. What is Data-First Product Lifecycle Management (PLM)?
Data-First PLM is an approach to managing the lifecycle of a product by leveraging data at every stage. It integrates insights from ideation to end-of-line, ensuring continuous improvement through feedback loops, AI-driven insights, and data analytics. This method streamlines workflows, reduces costs, and improves product quality.
2. How does AI enhance the ideation phase?
AI enhances ideation by analyzing vast datasets, including customer feedback, market trends, and competitor analysis. It identifies gaps in the market and predicts future demands. This ensures that ideas align with real-world needs and reduce the risk of pursuing unviable concepts.
3. What role does predictive analytics play in design and development?
Predictive analytics in design and development helps engineers anticipate potential issues. It guides material selection, optimizes design for manufacturing, and reduces the need for physical prototypes. This ensures that the product is not only innovative but also practical and cost-efficient.
4. Can small to mid-size manufacturers adopt data-first PLM effectively?
Absolutely. While large enterprises often lead the way, small to mid-size manufacturers can adopt scalable AI tools and cloud-based PLM systems. Starting with a pilot project is a great way to demonstrate value and build momentum for broader implementation.
5. What technologies are essential for implementing data-first PLM?
Key technologies include AI, IoT, cloud platforms, and advanced analytics tools. AI provides insights, IoT enables real-time data collection, and cloud platforms ensure data accessibility across teams. Together, they form an interconnected ecosystem to drive efficiency.
6. How does data-first PLM improve manufacturing efficiency?
Data-first PLM enhances manufacturing by leveraging real-time data from IoT sensors and AI analytics. It identifies production bottlenecks, enables predictive maintenance to prevent downtime, and optimizes energy use. This leads to higher productivity and lower operational costs.
7. What are the challenges of transitioning to data-first PLM?
Challenges include overcoming data silos, resistance to change from traditional workflows, and ensuring data quality. These can be addressed through unified platforms, training programs for teams, and robust data governance practices.
8. How does customer feedback integrate into the PLM cycle?
Customer feedback, gathered through reviews, surveys, and social media sentiment, feeds into the PLM system. It informs iterative updates, enhances product features, and guides future development. This ensures that products remain relevant and meet customer expectations.
9. Is a data-first approach suitable for all industries?
While highly beneficial in manufacturing, data-first PLM can be applied to various industries such as healthcare, technology, and retail. The principles of leveraging data for continuous improvement are universal, though the specifics may vary by sector.
10. What measurable outcomes can businesses expect from adopting data-first PLM?
Businesses can expect reduced time-to-market, significant cost savings, improved product quality, and higher customer satisfaction. Over time, the feedback loops create a self-sustaining cycle of innovation and operational excellence, driving long-term growth.
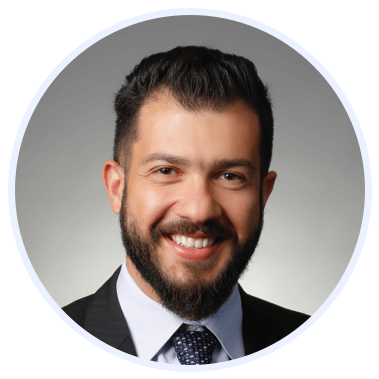
Rasheed Rabata
Is a solution and ROI-driven CTO, consultant, and system integrator with experience in deploying data integrations, Data Hubs, Master Data Management, Data Quality, and Data Warehousing solutions. He has a passion for solving complex data problems. His career experience showcases his drive to deliver software and timely solutions for business needs.