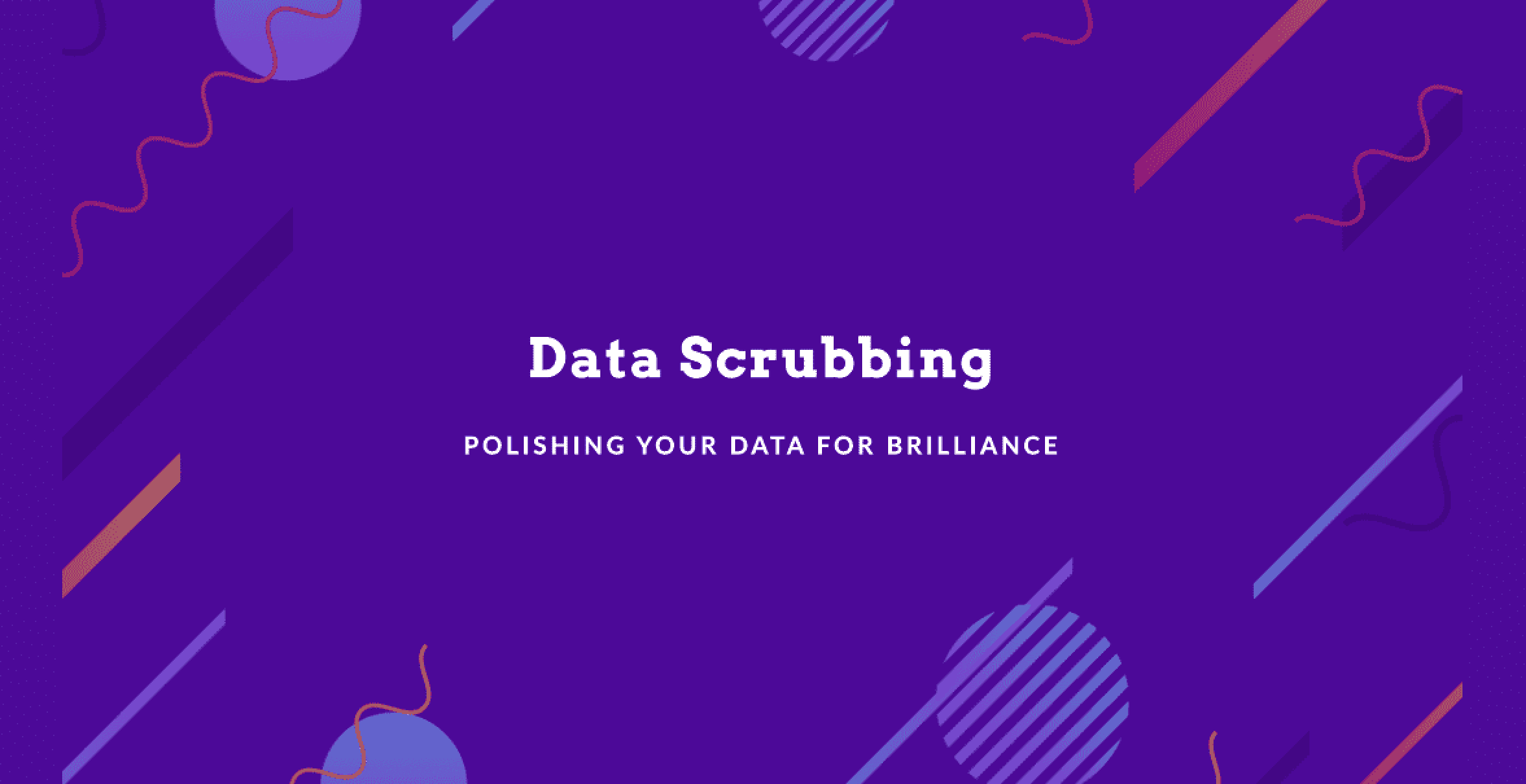

In the words of Edward R. Tufte, "Excellence in statistical graphics consists of complex ideas communicated with clarity, precision, and efficiency." In the age of data, where companies are increasingly leveraging big data and analytics to power decision-making, this quote couldn't be more poignant. Yet, to communicate complex ideas efficiently, we need clean, accurate data. Enter the realm of data scrubbing.
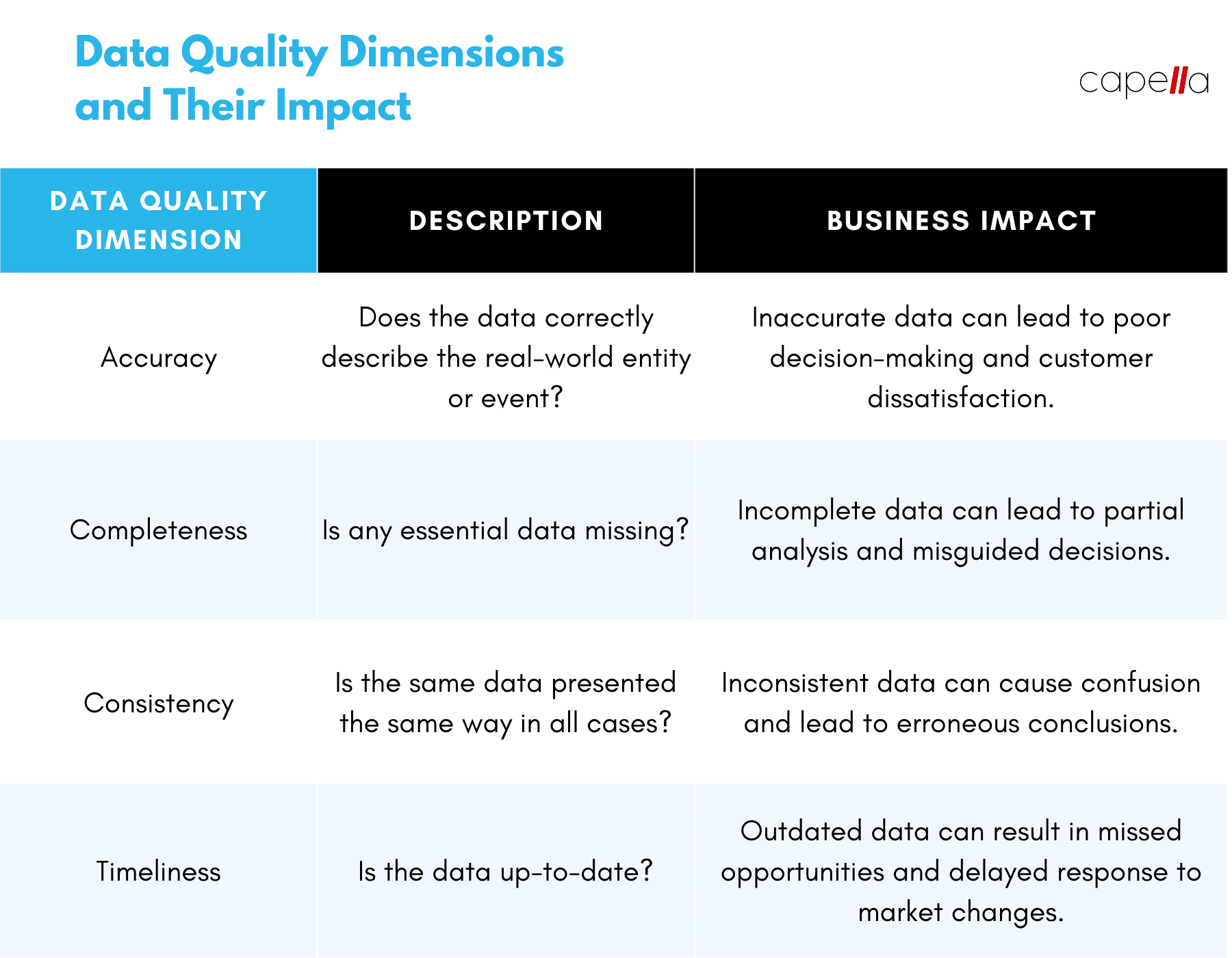
So, if you’re a top decision-maker in a large enterprise, it’s time to delve deeper into the realm of data scrubbing and understand its role in enabling smarter, more informed business decisions.
What is Data Scrubbing?
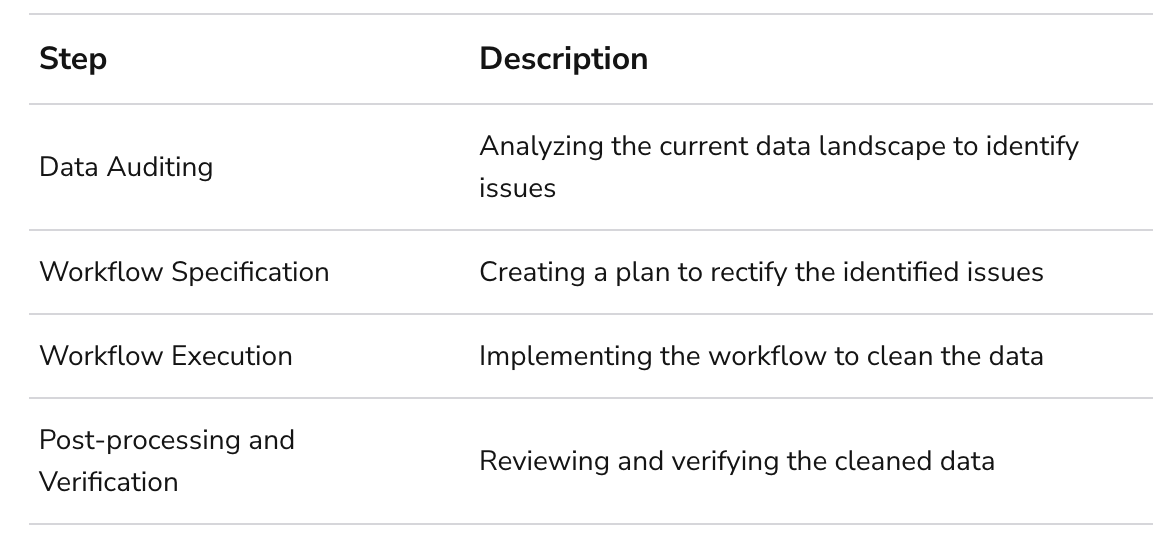
Data scrubbing, also known as data cleansing, is the process of inspecting, correcting (or removing) corrupt, inaccurate, incomplete, irrelevant, duplicated, or improperly formatted data within a dataset.
Data scrubbing is much like polishing a diamond. When mined, diamonds are rough, dirty, and unappealing. It takes a meticulous process to cut, shape, and polish them until they sparkle with unmatched brilliance.
Similarly, data collected from various sources come with numerous flaws. Data scrubbing is that meticulous process that cuts through the noise, shapes the data into a meaningful format, and polishes it to deliver clear, accurate, and actionable insights.

Why is Data Scrubbing Crucial?

Data is the new oil. But like crude oil, raw data must be refined before it can fuel business growth. Let's illustrate this with an example.
Consider an e-commerce company that collects data from its website, social media, customer reviews, and surveys. Each source offers different data formats and quality levels. Without a proper cleaning process, the company might run into several issues, such as:
- Incorrect customer data leading to flawed market segmentation
- Duplicated entries, causing skewed sales reports
- Misleading trend analysis due to incomplete historical data
In fact, according to IBM, poor data quality costs US businesses approximately $3.1 trillion every year.
Data scrubbing is thus not a choice, but a necessity. It helps enterprises maintain the quality of their data assets and make informed decisions based on accurate insights.
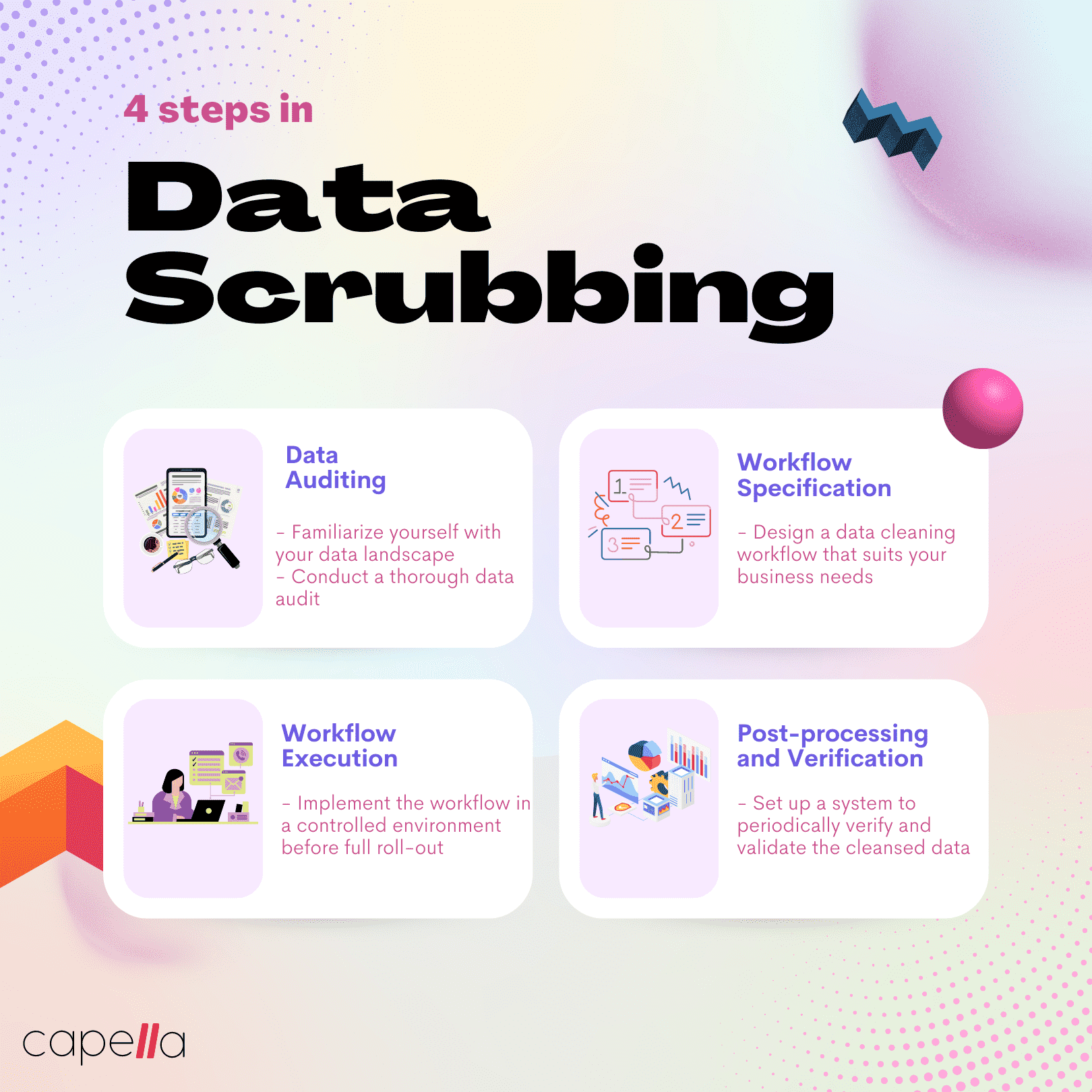
Steps in Data Scrubbing
Let's now delve into the key steps involved in data scrubbing:
1. Data Auditing

The process begins by understanding and assessing the current data landscape. During the audit, companies need to identify and document any anomalies, inconsistencies, or inaccuracies in the data.
2. Workflow Specification

Here, companies design a workflow to rectify the issues identified in the data audit. This step involves setting rules and parameters to fix, fill, or eliminate problematic data.
3. Workflow Execution

The workflow created in the previous step is then executed on the dataset. This phase should ideally include a dry run to test the effectiveness of the workflow before full execution.
4. Post-processing and Verification

After executing the workflow, it's essential to review and verify the cleansed data. Post-processing ensures the data is error-free and matches the predefined standards.
The following table summarizes the steps in data scrubbing:
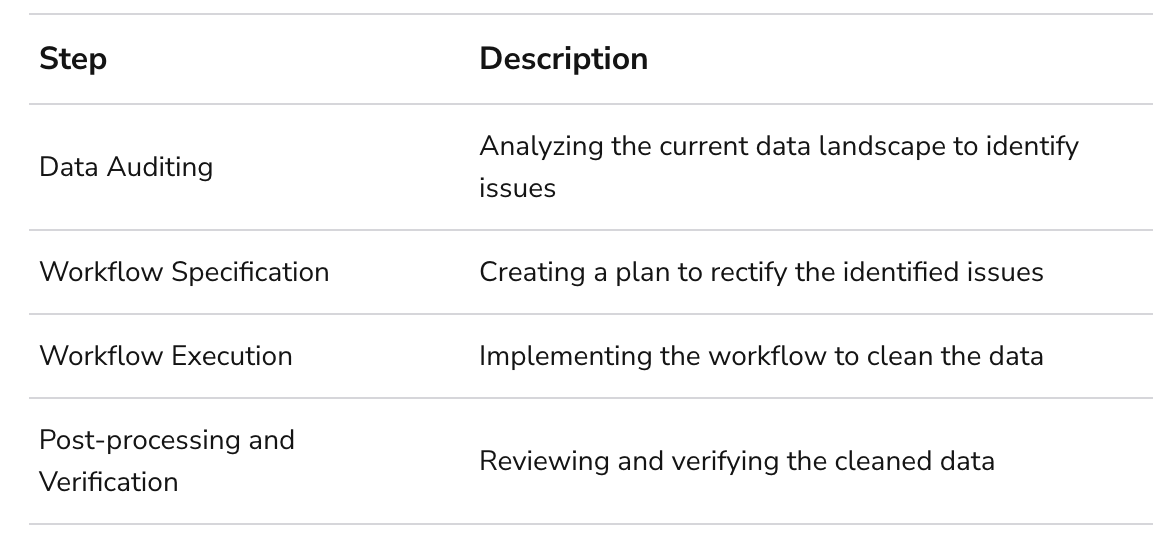
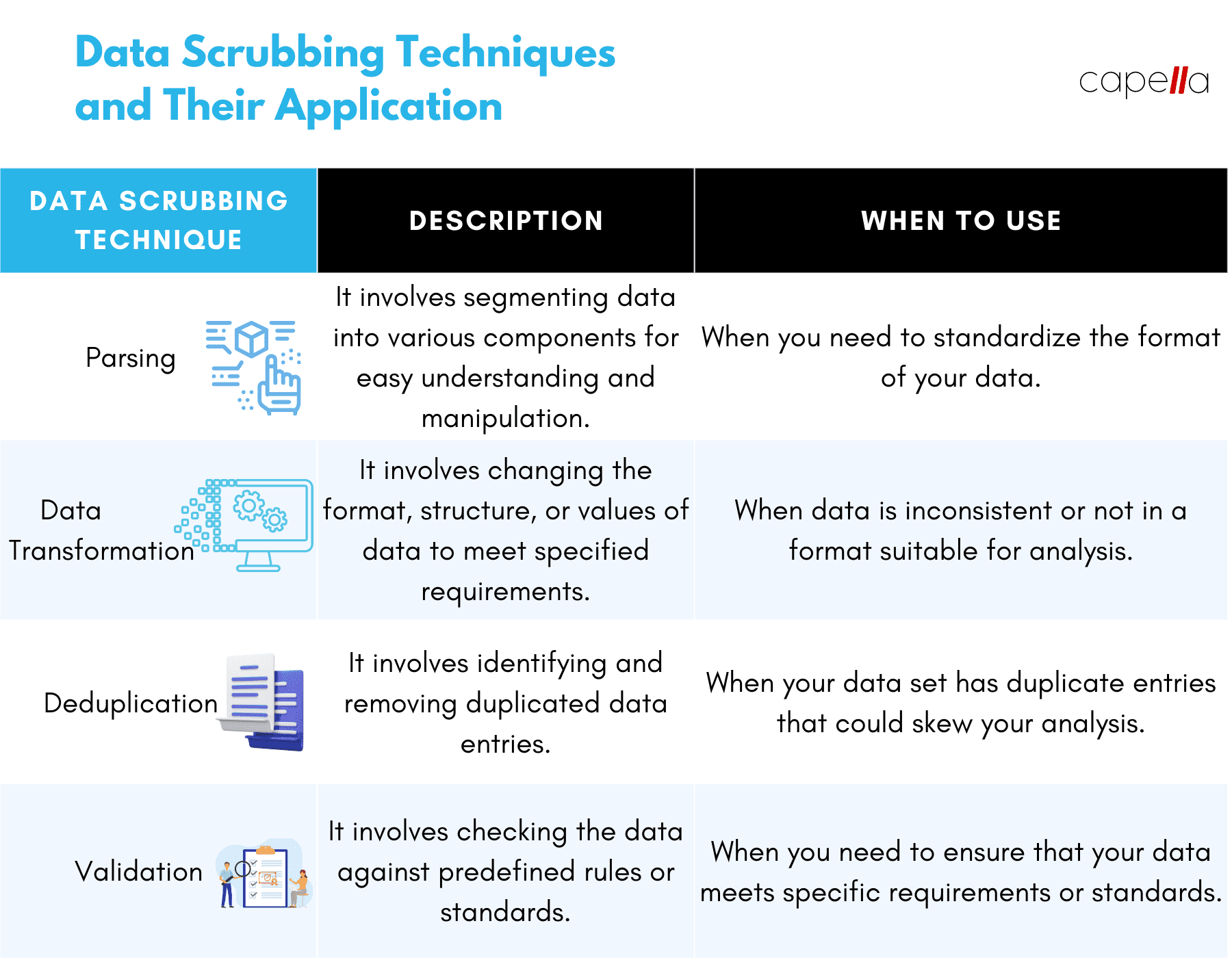
Tools for Data Scrubbing
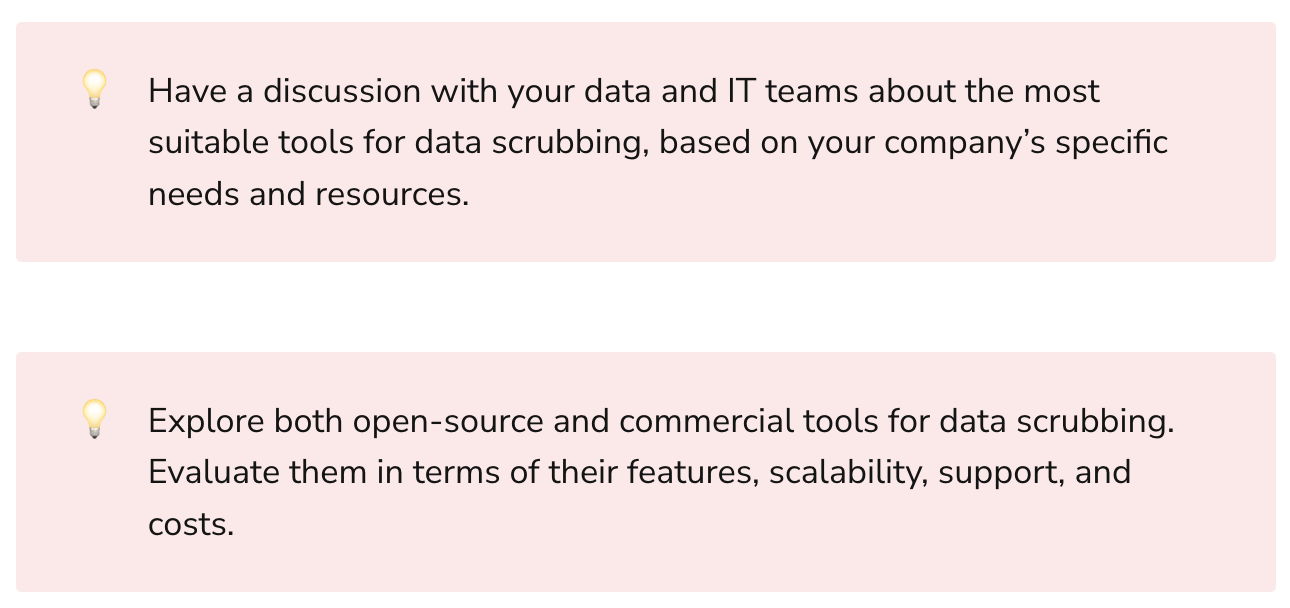
Companies can choose from an array of tools available for data scrubbing, ranging from open-source solutions like OpenRefine and Trifacta Wrangler to commercial ones like IBM InfoSphere and Talend Data Quality.
The choice of tool depends on several factors like the volume and complexity of data, business needs, budget, and technical capabilities of the team.
Practical Applications of Data Scrubbing
Let's explore a few practical examples to better understand how data scrubbing can be applied:
1. Enhancing Customer Experience in Telecom
In the telecom industry, data scrubbing plays a crucial role in enhancing customer experience. By ensuring accurate customer data, telecom companies can offer personalized services, leading to improved customer satisfaction.
For instance, an incorrect address in the customer database can result in failed product deliveries. Data scrubbing can correct these inaccuracies, thereby ensuring a smooth customer experience.
2. Optimizing Inventory in Retail
Retail businesses often struggle with inventory management due to inaccurate or duplicated product data. By scrubbing data, retailers can get an accurate picture of their stock levels and better forecast demand, thus optimizing inventory.
3. Streamlining Operations in Healthcare
Healthcare institutions deal with a vast amount of patient data, and inaccuracies can lead to serious consequences. Data scrubbing helps maintain accurate patient records, streamline operations, and facilitate better patient care.
Final Thoughts
In an age where data is at the heart of business strategy, ensuring data quality through data scrubbing is an investment that no company can afford to overlook.
After all, just as a polished diamond sparkles with unmatched brilliance, so does a company that harnesses the power of clean, accurate data.
As executives and decision-makers, the ball is in your court. Will you choose to mine raw diamonds and leave them in their rough state? Or will you commit to a rigorous polishing process to let your data shine with brilliance?
Remember, data scrubbing isn't a one-time process. It's a continuous endeavor that mirrors the iterative nature of data collection and usage. It is a journey, not a destination. It's the commitment to harness the power of data to its fullest extent, to drive your business forward, to empower decisions, and to enable growth.
In the grand scheme of things, data scrubbing is not just about cleaning data—it's about fueling business growth, enhancing customer satisfaction, and outshining the competition. So, the next time you think about data, consider this: are you just collecting it, or are you truly unleashing its potential?
Make the smart choice, embrace data scrubbing, and let your data shine with unmatched brilliance.
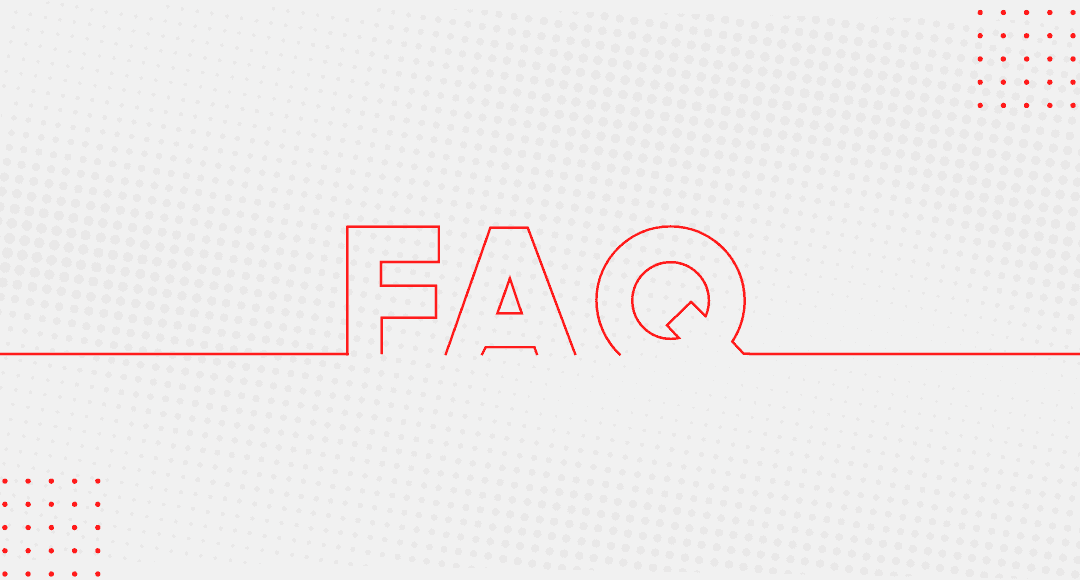
1. What exactly is data scrubbing?
Data scrubbing, also known as data cleansing, is the process of detecting, correcting or removing corrupt, inaccurate, incomplete, irrelevant, duplicated, or improperly formatted data within a dataset. It ensures that the data used in your organization is clean, consistent, and accurate, thus improving its quality and reliability for decision-making.
2. Why is data scrubbing important for businesses?
In today's data-driven world, organizations rely heavily on data to make informed decisions, forecast trends, and gain competitive advantage. If this data is incorrect or inconsistent, it can lead to inaccurate analyses, poor decision-making, and loss of business opportunities. According to IBM, poor data quality costs US businesses approximately $3.1 trillion each year. Data scrubbing helps businesses avoid these issues by ensuring the integrity and accuracy of their data.
3. How does data scrubbing work?
Data scrubbing typically involves four steps:
- Data Auditing: The current data landscape is analyzed to identify any anomalies, inconsistencies, or inaccuracies.
- Workflow Specification: A workflow is designed to rectify the issues identified in the data audit. This involves setting rules and parameters for how problematic data will be corrected, filled, or eliminated.
- Workflow Execution: The workflow is executed on the dataset to clean the data. Ideally, this step should include a dry run on a subset of the data to test the effectiveness of the workflow before full execution.
- Post-processing and Verification: After executing the workflow, the cleansed data is reviewed and verified to ensure it's error-free and matches predefined standards.
4. What are some tools used for data scrubbing?
Data scrubbing can be performed using a variety of tools, both open-source and commercial. Some popular options include OpenRefine and Trifacta Wrangler (open-source), and IBM InfoSphere and Talend Data Quality (commercial). The choice of tool depends on factors like the volume and complexity of data, business needs, budget, and technical capabilities of the team.
5. Can you provide some practical examples of data scrubbing?
Sure, let's consider three examples across different industries:
- Telecom Industry: Data scrubbing can help improve customer experience by ensuring accurate customer data. This allows telecom companies to offer personalized services and avoid issues like failed deliveries due to incorrect addresses.
- Retail Industry: Retailers can optimize inventory management through data scrubbing. By ensuring accurate and consistent product data, they can better forecast demand and manage stock levels.
- Healthcare Industry: Data scrubbing is vital for maintaining accurate patient records, which can streamline operations and improve patient care.
6. How often should data scrubbing be done?
Data scrubbing isn't a one-time task, it's an ongoing process. The frequency depends on several factors including the rate at which data is created, collected, or updated, the quality of the source data, and the criticality of data quality for your business operations and decision-making. Some organizations may need to scrub data daily, while others may do it weekly, monthly, or at other regular intervals.
7. Can data scrubbing be automated?
Yes, data scrubbing can be automated using various tools and software. Automation not only makes the process more efficient but also reduces the likelihood of human error. However, automated systems should be regularly monitored and updated to ensure they continue to meet the data quality requirements of the organization.
8. How does data scrubbing help with compliance?
In many industries, organizations must comply with regulations that require them to maintain accurate and auditable records. By ensuring the accuracy, completeness, and consistency of data, data scrubbing helps organizations meet these regulatory requirements and avoid potential penalties for non-compliance.
9. What challenges might an organization face when implementing data scrubbing?
While data scrubbing is crucial, it's not without challenges. Some common ones include:
- Large volumes of data can make the process time-consuming and resource-intensive.
- Determining which data is incorrect or inconsistent can be difficult, especially in complex datasets.
- Automation can introduce new errors if not properly monitored and managed.
- Securely handling sensitive data during the scrubbing process is essential to maintain privacy and comply with regulations.
10. How can an organization start with data scrubbing?
Here are some initial steps an organization can take:
- Understand the concept and purpose of data scrubbing.
- Conduct a preliminary assessment of the data landscape to identify potential issues.
- Discuss with the team the potential implications of poor data quality.
- Start a project for a thorough data audit.
- Design and test a data cleaning workflow.
- Evaluate tools for data scrubbing based on the organization's specific needs and resources.
- Implement data scrubbing in a controlled environment before rolling it out fully.
- Regularly verify and validate the cleansed data.
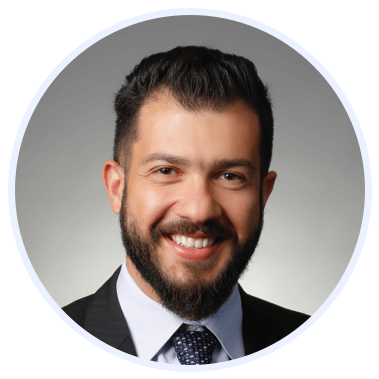
Rasheed Rabata
Is a solution and ROI-driven CTO, consultant, and system integrator with experience in deploying data integrations, Data Hubs, Master Data Management, Data Quality, and Data Warehousing solutions. He has a passion for solving complex data problems. His career experience showcases his drive to deliver software and timely solutions for business needs.