
It's a Monday morning, and imagine you're a healthcare executive, sipping your cup of morning coffee and looking at the day's schedule. You're running one of the largest healthcare enterprises, tasked with delivering top-quality care to hundreds of thousands, if not millions, of patients. You know that every decision you make could potentially affect the lives of those who entrust their health to you. It's a weighty responsibility, and the stakes are high.
Now, picture this: what if you had access to a crystal ball, one that could predict patient outcomes, optimize resource allocation, and drive operational efficiencies? Would that not be a game-changer?
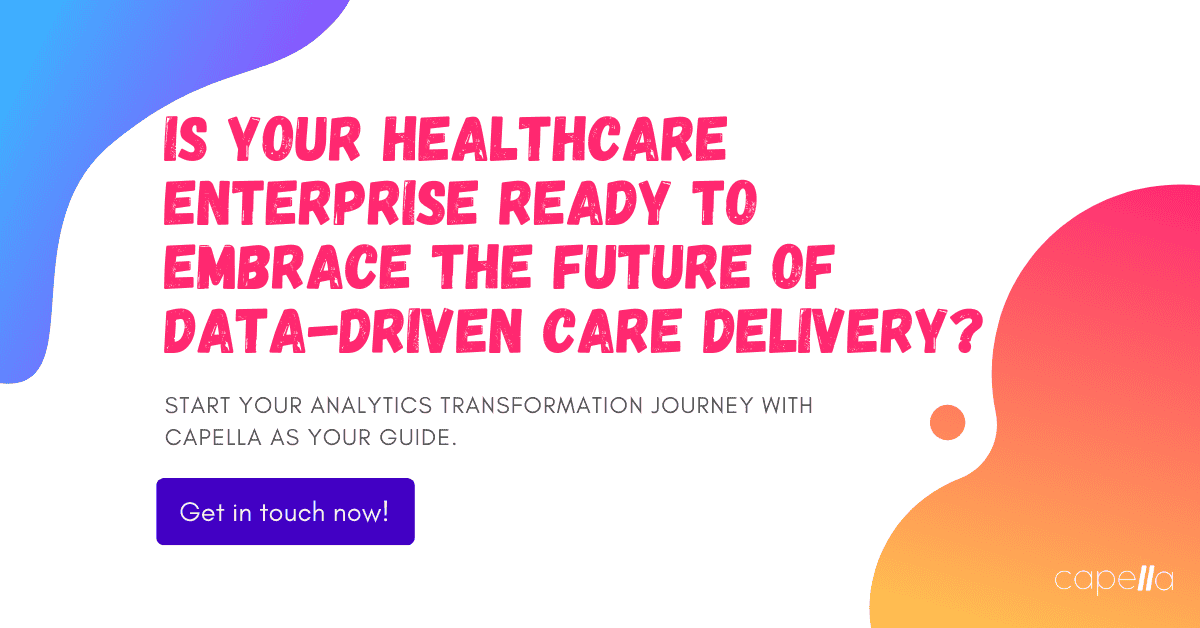
Well, data analytics in healthcare is your crystal ball. It's your secret weapon to staying ahead in the rapidly evolving healthcare landscape. This is not about conjuring numbers out of thin air, but leveraging data to make informed, strategic decisions.

The Power of Data Analytics in Healthcare

Data analytics refers to the process of examining, cleansing, transforming, and modeling data to discover useful information, suggest conclusions, and support decision-making. In the context of healthcare, this translates into predictive analytics, precision medicine, patient profiling, and more.
Consider the complexity of the modern healthcare ecosystem. It's a multi-headed hydra, spanning numerous areas from patient care to supply chain management, from regulatory compliance to workforce planning. With so many moving parts, it's easy to lose sight of the bigger picture. That's where data analytics steps in, providing a bird's eye view of the enterprise and revealing insights that were hitherto unknown.
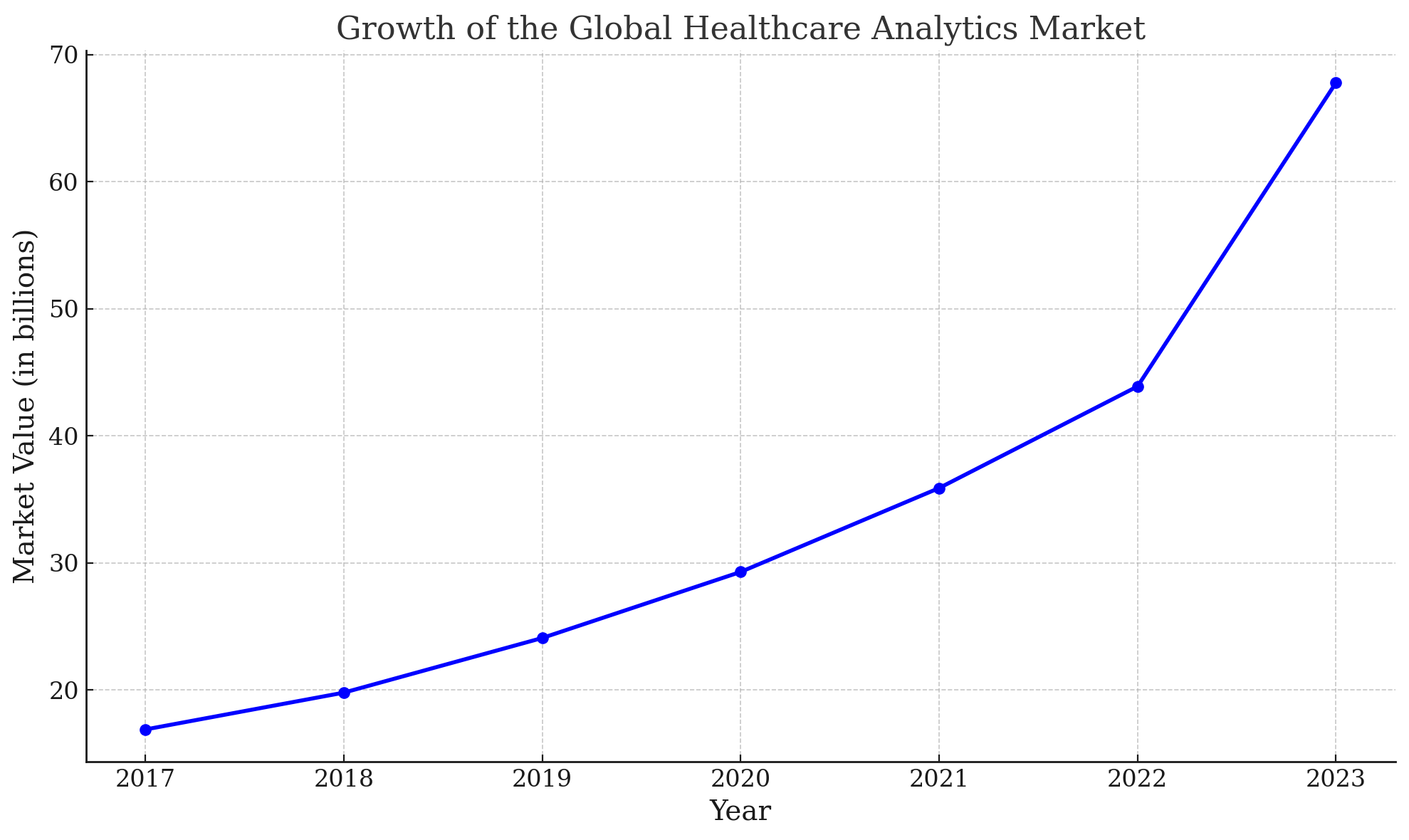
If this sounds exciting, it's because it is! In fact, according to a report by Allied Market Research, the global healthcare analytics market was valued at $16.9 billion in 2017 and is projected to reach $67.8 billion by 2023, growing at a CAGR of 19.1% from 2018 to 2023.

The Case of Cleveland Clinic: A Success Story
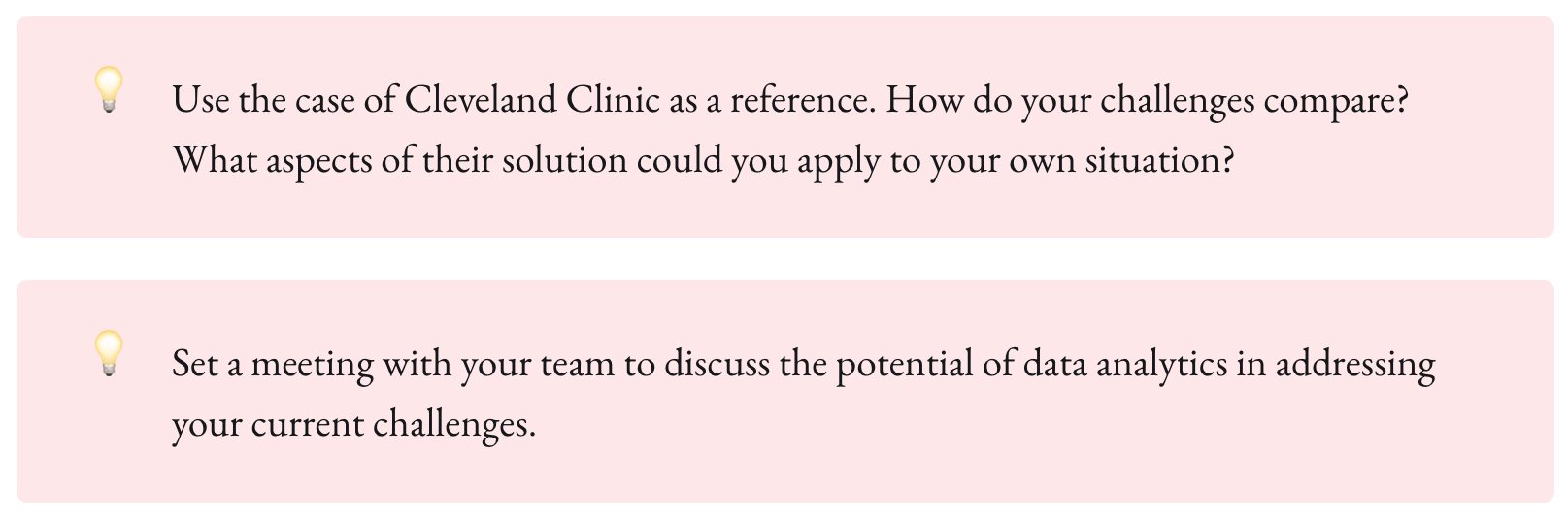
Let's explore a real-life example: Cleveland Clinic, a globally-renowned healthcare provider, constantly aiming for excellence. Like many leading healthcare institutions, Cleveland Clinic faces the challenges of ensuring top-tier care while juggling operational efficiencies and patient satisfaction.
The Challenge
Cleveland Clinic's primary challenges included:
- Rising costs: Like many of its peers, Cleveland Clinic was keen on optimizing its expenditure, especially concerning avoidable hospital readmissions. Identifying root causes and devising effective strategies was essential.
- High readmission rates: Ensuring that their readmission rates were not just below the national average but set a gold standard was a priority. This was vital for their quality metrics and their global reputation.
- Patient dissatisfaction: Even a slight percentage of patients feeling that their care was impersonal could affect the reputation of an institution like Cleveland Clinic. They constantly strive to ensure every patient feels recognized and understood.
The Solution
Cleveland Clinic, known for its forward-thinking approach, decided to delve deep into data analytics to refine its operations and patient care strategies. Collaborating with leading data analytics firms, they set up a robust data-driven framework.
The application of data analytics at Cleveland Clinic led to:
- Predictive analytics: The implemented solution unearthed patterns in patient readmissions. By scrutinizing variables such as patient demographics, disease categories, prescribed treatments, and socio-economic factors, it became possible to predict potential readmission risks. Cleveland Clinic used this data to devise personalized care strategies, playing a crucial role in minimizing readmission cases.
- Resource optimization: Through data insights, inefficiencies in resource allocation, from bed management to workforce deployment, were spotlighted. Addressing these inefficiencies allowed Cleveland Clinic to streamline operations and further elevate patient care standards.
- Patient profiling: Using comprehensive patient data, Cleveland Clinic began tailoring care plans even more intricately, ensuring that each patient felt thoroughly understood and valued.
The Results
Cleveland Clinic's foray into data analytics was predictably successful. With a notable decline in readmission rates and operational costs and a surge in patient satisfaction metrics, their commitment to data-driven insights was further solidified.
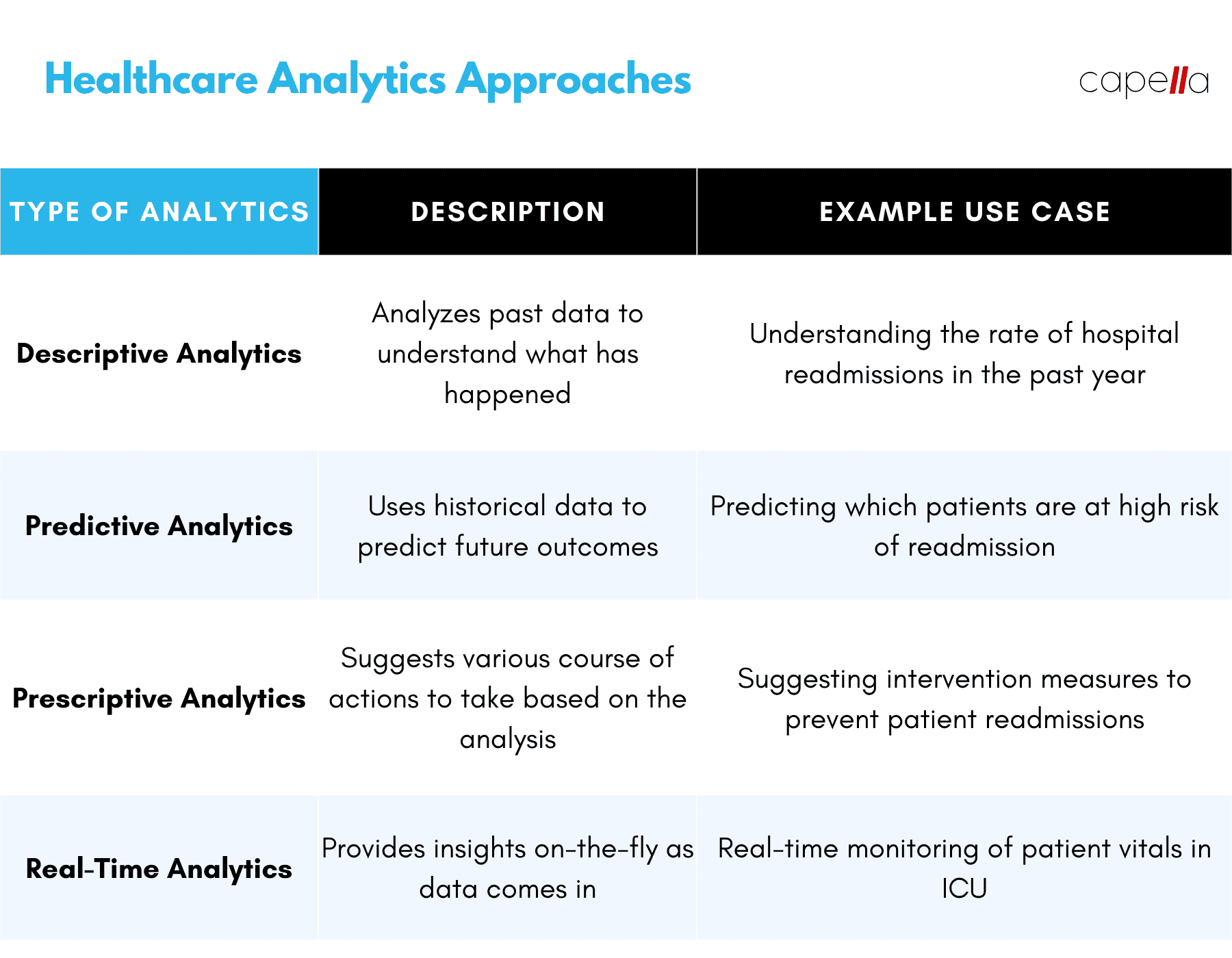
More Examples: Kaiser Permanente and NorthShore University HealthSystem
Data analytics is not just a concept; it's a practical tool that's transforming healthcare. Let's look at two real-world examples:
- Kaiser Permanente: Kaiser Permanente, a healthcare consortium, used a combination of analytics, machine learning, and AI to reduce patient waiting times and streamline operations. Their Operations Watch List (OWL), a mobile app, provides a near real-time view of key hospital metrics, including hospital census, bed demand and availability, and patient discharges. The app has reduced patient wait times for admission to the emergency department by an average of 27 minutes per patient and saved hospital managers an average of 323 minutes per month spent manually preparing data for operational activities.
- NorthShore University HealthSystem: NorthShore used data and predictive analytics to determine which chest pain patients should be admitted for observation and which should be sent home. They developed a "Technology-driven Chest Pain Management in the ED" program, which puts predictive analytics directly into physicians' and nurses' workflow. This program has helped NorthShore reduce unnecessary hospitalizations and optimize patient care. Their Chest Pain Observation Days rate decreased by 10% without increasing the rate of ED returns, mortality, or morbidity.
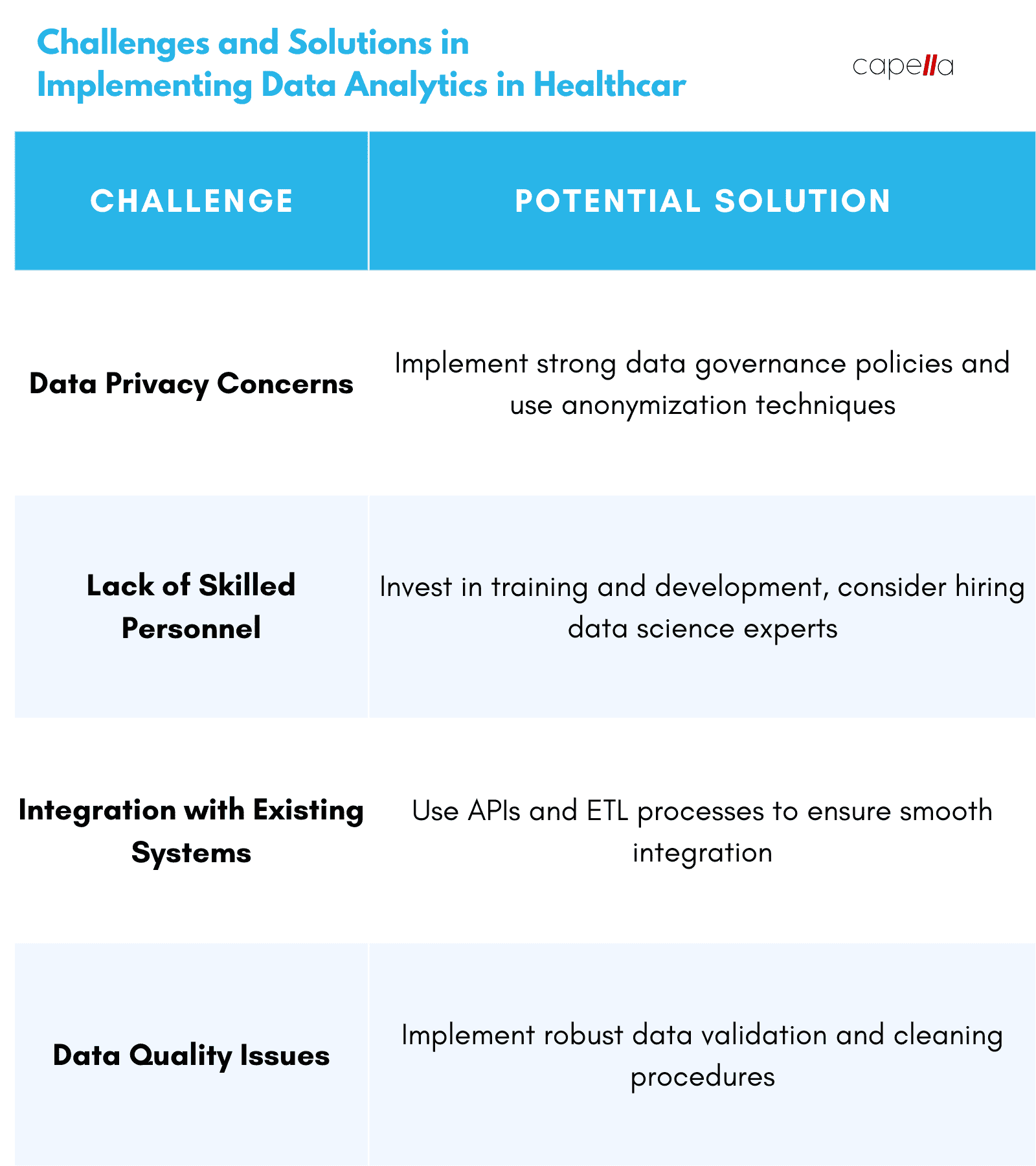
Lessons Learned and Best Practices
In the journey of Cleveland Clinic, Kaiser Permanente, and NorthShore University HealthSystem, we find valuable lessons and best practices for other healthcare executives considering the use of data analytics:
- Continuous feedback is key: Regularly incorporating feedback from end users allows for continuous refinement of solutions, delivering the most relevant information and functionality to support ease of use and maximize value delivered.
- Focus on user experience: Getting clinicians to adopt tools like this requires making the experience seamless. Integration of any new analytics-driven technology must be in the clinical chart or the value will diminish.
- Leverage predictive analytics: Predictive analytics can help identify patterns in patient readmissions, resource utilization, and more. This can enable healthcare providers to develop personalized care plans and preventive measures, significantly improving patient care.

Conclusion
In an industry where life-altering decisions are made every day, healthcare executives cannot afford to ignore the power of data analytics. It's not just about big data or fancy algorithms; it's about harnessing this data to drive actionable insights, optimize resources, and ultimately, deliver better patient care.
As a healthcare executive, your job isn't just about managing a hospital or a healthcare system. It's about making a difference in the lives of your patients. And data analytics can be your secret weapon to do just that.
So, as you sip your coffee, consider this: are you ready to embrace the power of data analytics in your healthcare enterprise? The future of healthcare is here, and it's data-driven.
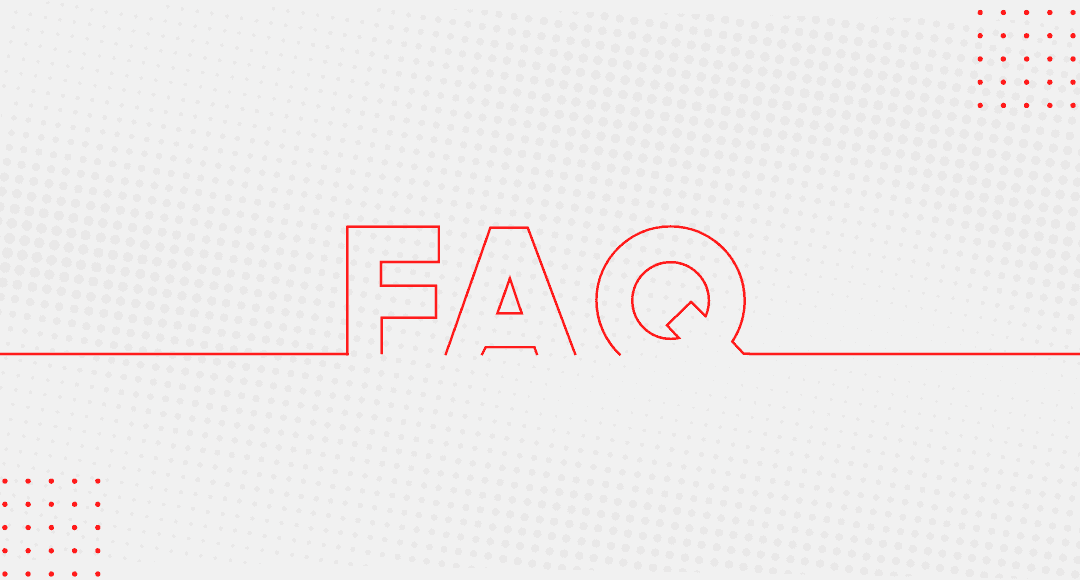
What is data analytics in healthcare?
Data analytics in healthcare is the practice of using data-driven findings to predict and solve health-related issues. It involves collecting and interpreting health data to improve care, reduce costs, and enhance patient experiences. This can range from analyzing patient records to predict disease outcomes to using machine learning algorithms to automate administrative tasks.
Why is data analytics important in healthcare?
Data analytics is essential in healthcare for several reasons. It allows healthcare providers to offer personalized patient care based on individual health data. It can help predict outbreaks of epidemics and improve the efficiency of health services. Data analytics can also reduce costs by identifying inefficiencies in healthcare delivery and predicting patient outcomes to guide treatment plans.
How is data analytics used in healthcare decision making?
In healthcare decision-making, data analytics can be used to provide evidence-based answers. This can involve analyzing patient data to determine the best treatment plan, using predictive models to identify high-risk patients and guide resource allocation, or interpreting operational data to improve service delivery and patient satisfaction.
What are some real-world examples of data analytics in healthcare?
There are numerous examples of data analytics in healthcare. For instance, Kaiser Permanente used data analytics to reduce patient wait times and streamline operations. They developed a mobile app called Operations Watch List (OWL) that provided real-time data on key hospital metrics, helping hospital leaders make informed decisions. Another example is NorthShore University HealthSystem, which used predictive analytics to determine which chest pain patients should be admitted for observation, reducing unnecessary hospitalizations.
What are some challenges in implementing data analytics in healthcare?
Challenges in implementing data analytics in healthcare include data privacy concerns, integration with existing systems, lack of skilled personnel, and data quality issues. Overcoming these challenges often involves investing in data governance, hiring or training data science experts, ensuring smooth system integration, and implementing robust data validation and cleaning procedures.
What are the steps in the data analytics process in healthcare?
The data analytics process in healthcare involves several steps, including data collection, data cleaning and preparation, data analysis, insight generation, and decision making. Each step is crucial for delivering reliable, actionable insights.
How can healthcare organizations start implementing data analytics?
Healthcare organizations can start implementing data analytics by first assessing their current situation and understanding the challenges they face. They can then research the latest trends and applications of data analytics in healthcare and develop a roadmap for implementation. Starting with small projects can help gather feedback and refine the approach before scaling the use of data analytics across the organization.
What is the future of data analytics in healthcare?
The future of data analytics in healthcare looks promising, with advancements in technologies like machine learning and AI set to revolutionize healthcare delivery. Potential future applications include personalized medicine, predictive diagnostics, real-time alerting, and advanced imaging analytics.
What are the different types of analytics in healthcare?
In healthcare, there are typically four types of analytics: descriptive, predictive, prescriptive, and real-time analytics. Descriptive analytics analyzes past data to understand what has happened, predictive analytics uses historical data to predict future outcomes, prescriptive analytics suggests various course of actions to take based on the analysis, and real-time analytics provides insights on-the-fly as data comes in.
How can data analytics improve patient satisfaction in healthcare?
Data analytics can enhance patient satisfaction in healthcare by providing personalized patient care, reducing wait times, and improving health outcomes. By analyzing patient feedback, healthcare providers can identify areas for improvement and take action to enhance the patient experience. Predictive analytics can also enable proactive care, addressing potential health issues before they become serious problems.
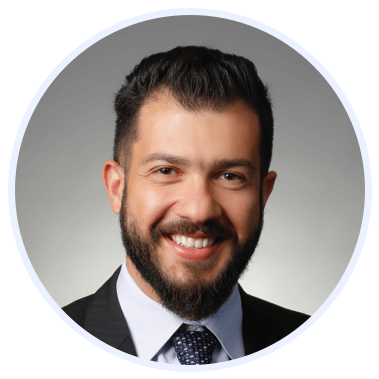
Rasheed Rabata
Is a solution and ROI-driven CTO, consultant, and system integrator with experience in deploying data integrations, Data Hubs, Master Data Management, Data Quality, and Data Warehousing solutions. He has a passion for solving complex data problems. His career experience showcases his drive to deliver software and timely solutions for business needs.