
Manufacturing has historically taken a heavy toll on the environment. From mining raw materials to powering factories, the manufacturing process has contributed significantly to issues like pollution, waste, and climate change. However, with advanced technologies like artificial intelligence (AI), there is an opportunity to transform manufacturing into a more sustainable industry.
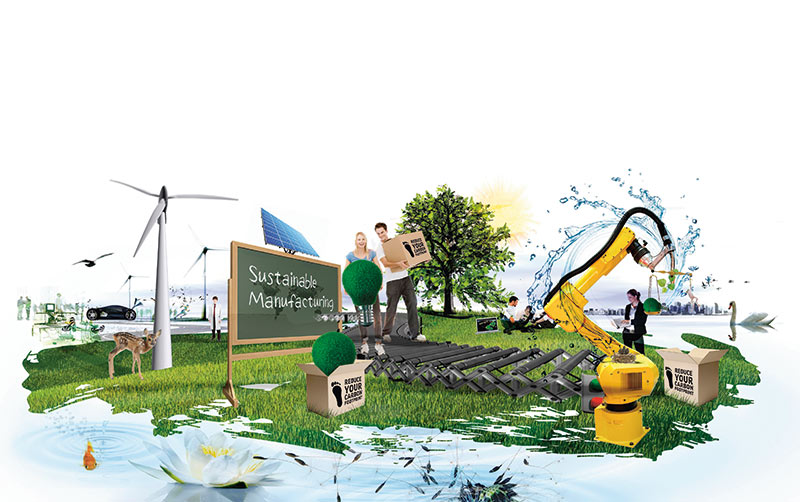
In this blog post, we'll explore how AI can enable more eco-friendly manufacturing practices. We'll cover topics like:
- Reducing energy usage and emissions through predictive analytics and automation
- Optimizing supply chains and logistics for sustainability
- Monitoring product quality and extending lifespan to reduce waste
- Enabling circular manufacturing with advanced robotics and 3D printing
- Tracking and analyzing sustainability metrics across operations
By deploying AI strategically, manufacturers can minimize their environmental impact while still producing high-quality goods profitably. Let's dig in!
Reducing Energy Usage Through AI

Manufacturing facilities require massive amounts of energy to power machinery, maintain operations, and regulate indoor environments. This contributes heavily to greenhouse gas emissions. AI presents opportunities to dramatically improve energy efficiency:
Predictive Maintenance
Unexpected downtime is expensive - lost production can cost over $260,000 per hour for some manufacturers. AI-enabled predictive maintenance analyzes data from sensors and equipment to forecast problems before they occur. This allows manufacturers to plan maintenance optimally, minimizing disruptions and energy waste.
According to ARC Advisory Group, predictive maintenance can reduce downtime by 30-50% and cut maintenance costs by 8-12%. The associated energy savings are significant.
Dynamic Optimization
AI can dynamically optimize energy usage across manufacturing operations. Algorithms analyze production schedules, equipment loads, and facilities data to spot opportunities to:
- Shift energy usage to off-peak times to reduce peak demand charges
- Scale equipment up or down to match real-time requirements
- Tune HVAC settings based on weather and occupancy patterns to reduce electricity waste
This level of smart energy optimization can generate over 10% in energy savings according to the U.S. Department of Energy.
Predictive Analytics for Renewables
For manufacturers using renewable energy like solar or wind, AI predictive analytics can forecast weather patterns and renewable energy production. This allows energy managers to optimize consumption around these fluctuations in renewable output.
AWS notes that this approach can increase renewable energy utilization by up to 20% for some manufacturers. The less carbon-intensive grid energy needed, the lower the emissions.
By leveraging techniques like predictive maintenance, real-time optimization, and renewable energy forecasting, manufacturers can significantly reduce energy consumption across their operations via AI.
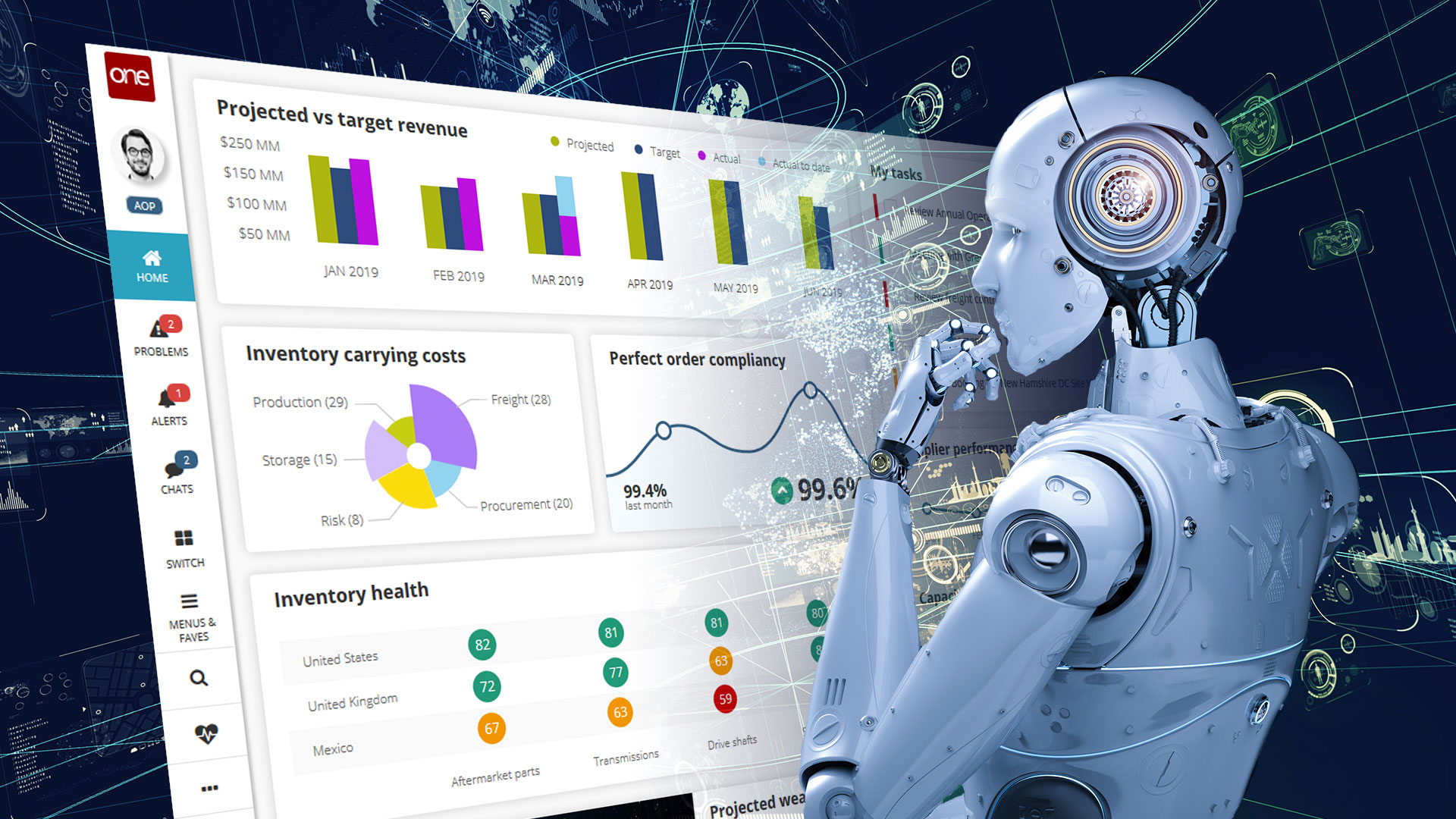
Optimizing Supply Chains via AI

Manufacturing supply chains account for over 75% of a product's emissions on average, according to CarbonTrust. AI presents opportunities to optimize supply chain management and logistics for sustainability:
Strategic Sourcing Optimization
AI algorithms can analyze parameters like cost, emissions, lead times, and risk to model sourcing scenarios and identify optimal suppliers. This allows manufacturers to select vendors and locations that minimize carbon footprints.
FarEye shares an example of a fast food chain using this approach to choose regional produce suppliers. By sourcing locally, they reduced supply chain miles by over 284,000 km per year.
Dynamic Logistics Optimization
AI can enable dynamic optimization of logistics like transportation routes and vehicle loads. For example, algorithms can:
- Consolidate shipments with higher fill rates to reduce the number of trips needed
- Sequence routes to minimize mileage and avoid congestion
- Select energy-efficient transport like rail or electric vehicles for legs of journeys
- Optimize loads and capacity to avoid half-empty trucks or containers
According to Shipwell, AI-powered logistics optimization can improve freight efficiency over 25% and reduce emissions by 20 tonnes per shipment.
Automated Warehouses
AI robotics enable automated storage and retrieval in smart warehouses, avoiding human errors that lead to overpacking and product damage. This reduces materials waste and the need for re-shipments.
For example, Ocado's automated warehouses have 50% less ambient temperature change than typical warehouses. This drastically cuts refrigeration needs and energy waste.
Monitoring Product Quality via AI

Product defects lead to waste when items are scrapped or returned. AI quality inspection offers new ways to boost quality and product lifespan:
Automated Visual Inspection
AI-powered sensor systems can visually inspect items on production lines for micro-defects. This real-time feedback allows issues to be corrected instantly, before more waste is generated.
According to Matrox, AI visual inspection can detect 6 times more defects than manual inspection. The higher quality leads to less scrapped items.
Predictive Quality Analytics
By analyzing product testing data and machine sensor data with AI, manufacturers can predict failures before they happen. This allows maintenance and adjustments to avoid costly defects.
For example, see how AI improved quality for an optical products manufacturer, reducing rejects by over 35%. Fewer faulty products shipped means less waste.
Extended Lifespan with ML
AI techniques like reinforcement learning allow robots to be trained by trial-and-error to assemble items perfectly. This leads to products that last longer with fewer flaws.
Check out this BMW example where AI-guided assembly robots created cars matching strict 10-year lifespan requirements. Tighter quality results in longer useful product lifetimes.
Towards Circular Manufacturing
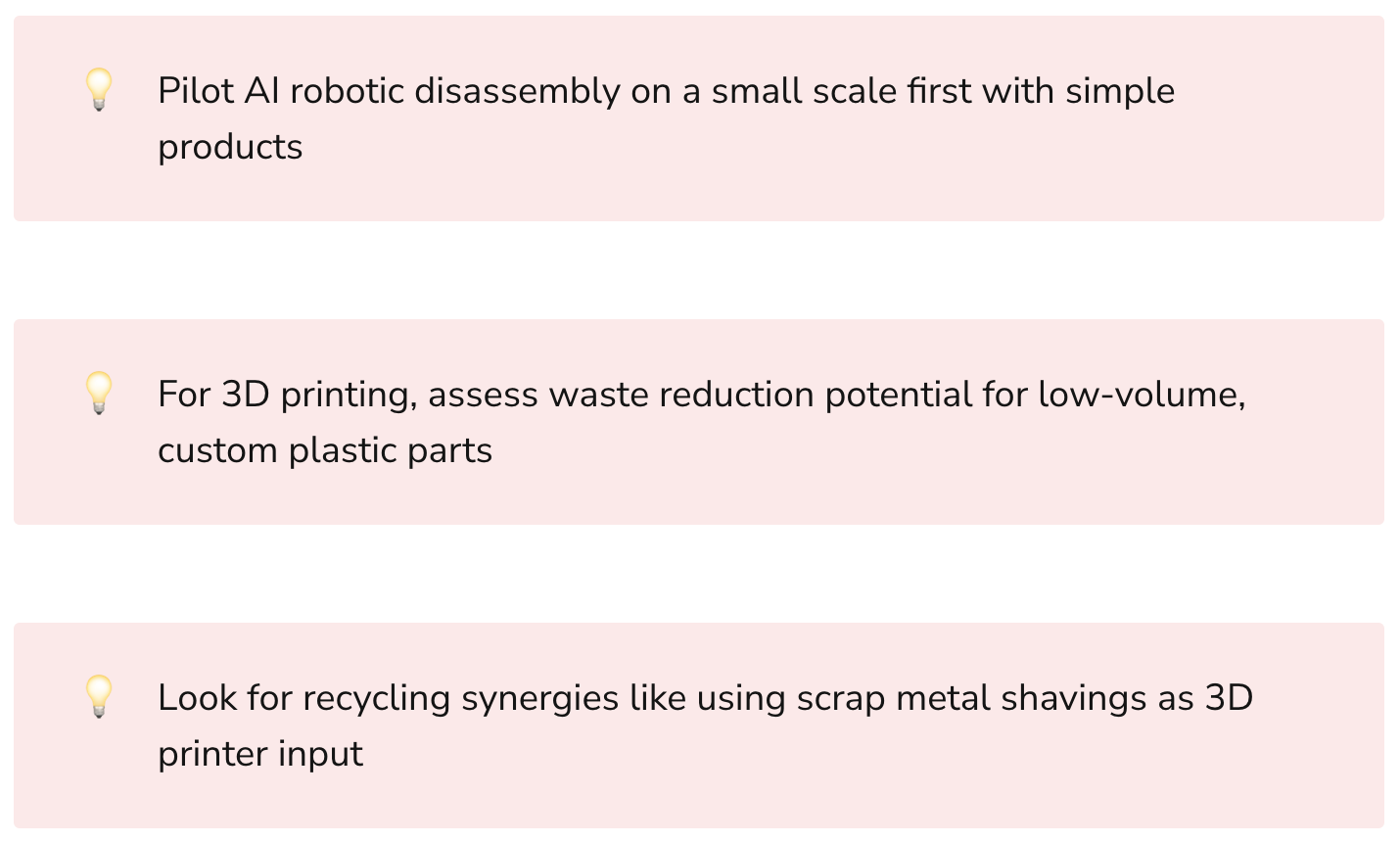
The circular economy aims to eliminate waste by recycling materials into new products. AI is enabling innovations like autonomous disassembly and 3D printing with recycled materials:
Autonomous Disassembly Robots
Recycling requires separating and sorting materials, which is often still done manually. AI robotics can now automate disassembly for products like electronics or vehicles into reusable components.
For example, ZenRobotics builds robots that use AI vision and grippers to dismantle construction waste safely. This automates recycling prep work traditionally done manually.
AI-Optimized 3D Printing
3D printing with recycled plastics is an emerging circular solution. AI algorithms can optimize print designs, schedules, and material mixes to minimize print waste.
Check out this HP example where AI-powered 3D printing with recycled materials reduced waste by 65% versus traditional methods.
Tracking Sustainability via AI
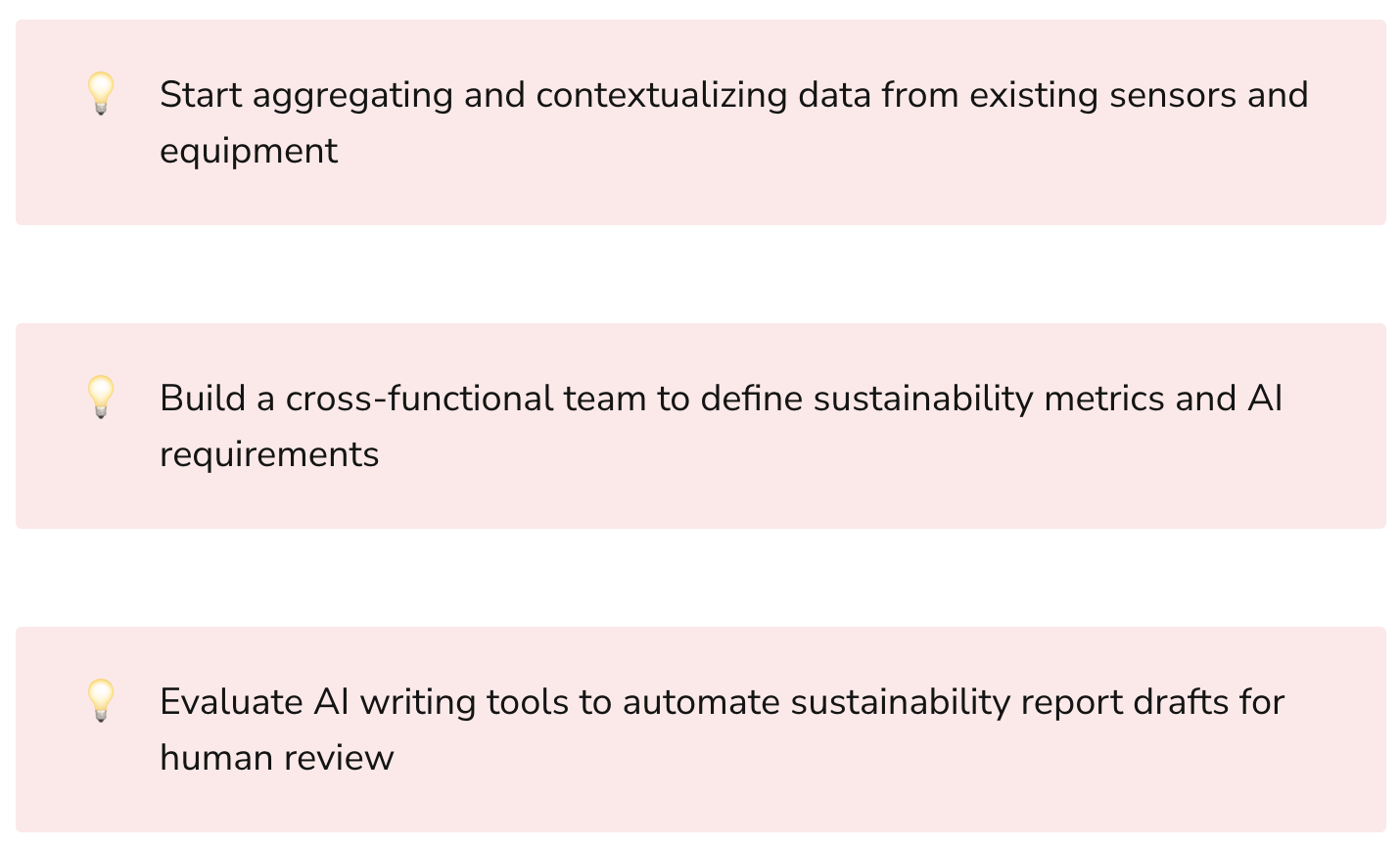
To manage sustainability performance, manufacturers need to monitor metrics like energy use, emissions, waste generation, and material toxicity. AI offers new ways to track these metrics:
Real-time Data Collection
IoT sensors connect manufacturing equipment for constant data collection, managed via cloud analytics. This allows real-time monitoring of electricity use, waste levels, output quality, and other metrics.
Automated Sustainability Reporting
Natural language generation AI can turn IoT sensor data into written sustainability reports. This automates much of the manual reporting process for metrics like energy efficiency or scrap rates.
For example, see how AI reporting tools could save 4,000 man-hours per year for a large manufacturer by autogenerating sustainability reports.
AI Carbon Accounting
New AI solutions can automatically calculate carbon footprints across supply chains using data like fuel types, logistics modes, and energy sources.
For instance, see how Amazon leverages AI to optimize delivery routes for lower emissions and power warehouses using the cleanest energy sources.
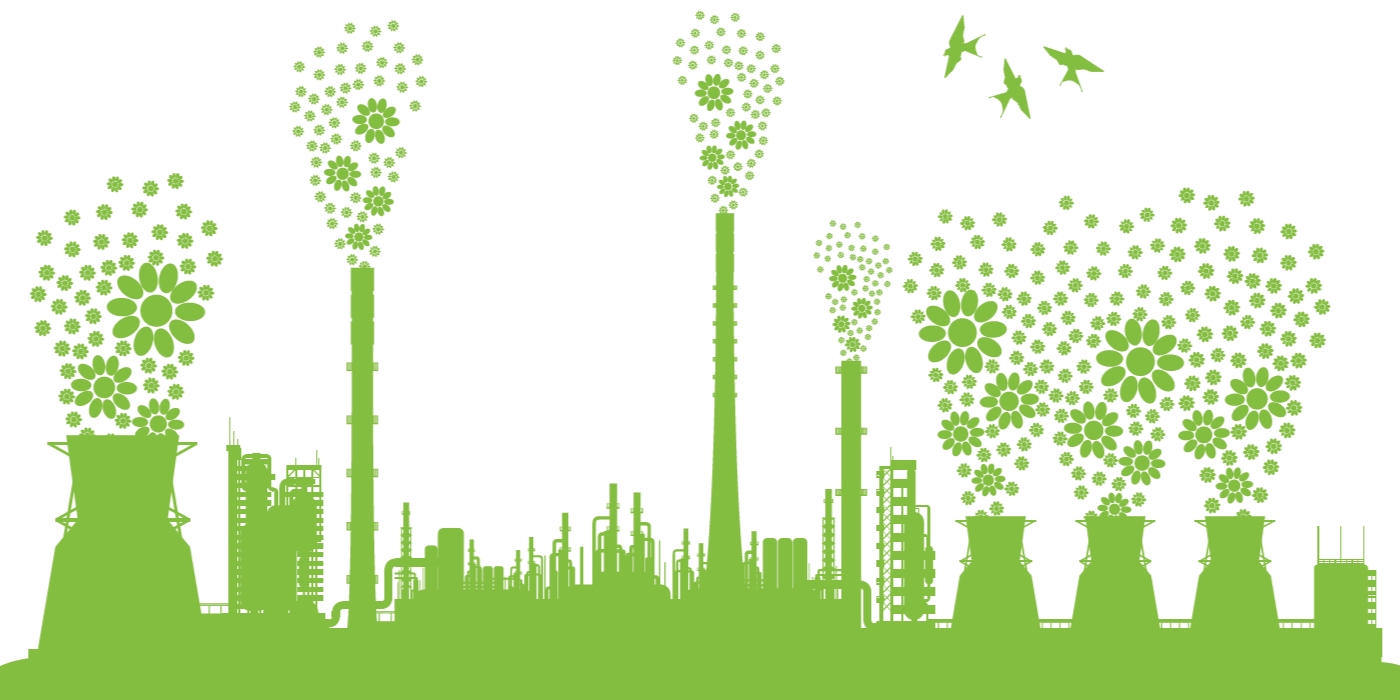
The Future of AI in Manufacturing
As the examples above demonstrate, AI has huge potential to enable more sustainable manufacturing across energy management, supply chains, quality control, circular production, and sustainability reporting.
Adoption is still in the early stages, but we expect accelerated investment in manufacturing AI as corporations commit to greener operations. Key enablers will include:
- Cheaper sensor technology - Cutting the costs of IoT sensors to collect rich data
- Expanded cloud analytics - Managing massive sensor data streams via cloud
- Investment in sustainability metrics - Building datasets to train AI models
- Open data sharing - Enabling collaboration on sustainability use cases
As AI capabilities continue improving while sensor and cloud costs decrease, AI-driven sustainability will become an imperative, not just an option. Companies failing to leverage manufacturing AI risk competitive disadvantage as leaders create efficiencies and cost advantages from optimized green operations.
The next decade will see manufacturers race to embed intelligence throughout their operations using techniques like industrial IoT, digital twins, and ultimately AI-guided self-optimizing factories. Those putting sustainability at the center of their AI roadmap will lead the pack.
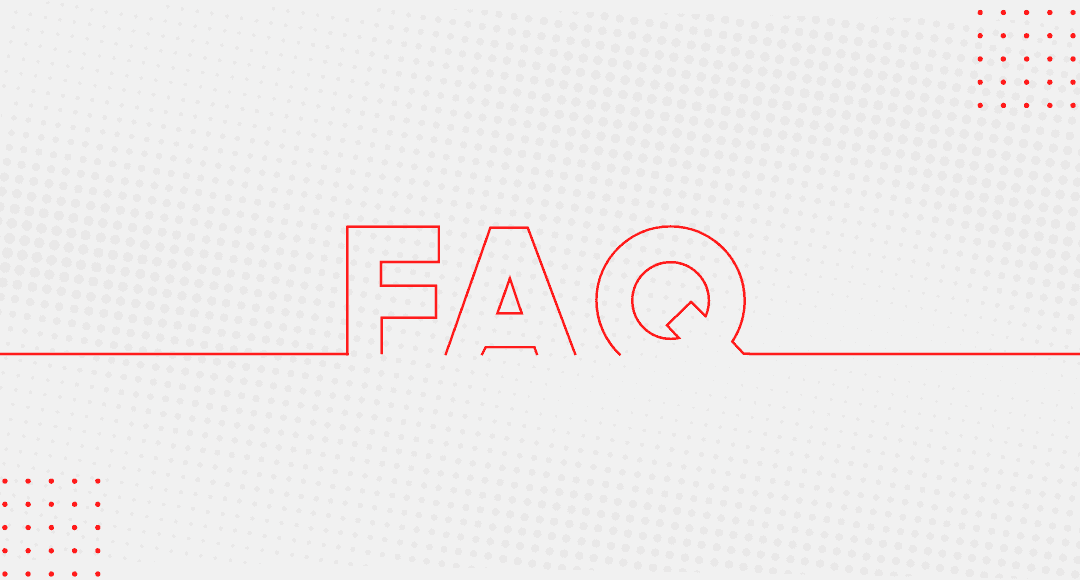
What are some of the main environmental impacts of traditional manufacturing?
Traditional manufacturing is energy-intensive and generates significant greenhouse gas emissions. Key environmental impacts include:
- Energy consumption - Manufacturing accounts for about 25% of primary energy use globally. Fossil fuels like coal and natural gas are still predominant.
- Air pollution - Toxic air emissions come from powering facilities, operating equipment, and production processes. Particulates, VOCs, and other hazardous air pollutants are released.
- Waste generation - Manufacturing waste includes hazardous solid/liquid waste from processes, leftover raw materials, and defective products. Much of this waste ends up in landfills.
- Water usage - Manufacturing utilizes large volumes of water for heating, cooling, cleaning, and as a raw material. Discharge leads to freshwater depletion and ocean/waterway pollution.
- Supply chain emissions - Raw material extraction, logistics, and product lifecycles have massive carbon footprints. Manufacturing drives these expansive supply chains.
How does AI enable manufacturers to be more energy efficient?
AI improves energy efficiency in manufacturing operations through:
- Predictive maintenance - Algorithms analyzing equipment data can forecast failures before they occur, allowing maintenance to be scheduled proactively. This minimizes costly downtime where energy is still expended.
- Real-time optimization - AI systems can continuously adjust parameters like equipment loads, building temperatures, and production schedules to optimize energy use.
- Renewables integration - Predictive analytics on weather data and renewable energy output allows consumption to be shifted to match clean energy availability.
- Smart grid interactions - Utilities apply AI to better predict demand, integrate renewable energy, and incentivize large consumers like manufacturers to shift usage and flatten peak loads.
How can AI make manufacturing supply chains more sustainable?
AI optimizes supply chain sustainability by:
- Selecting optimal suppliers and locations based on emissions, logistics costs, renewables usage etc.
- Consolidating shipments and optimizing routes dynamically to reduce transport distances.
- Orchestrating transport across modes like rail and electric vehicles to lower emissions.
- Reducing overpacking and enabling automated warehousing to cut waste.
- Increasing recycling by automating disassembly of products using AI robotics.
What are some key benefits of applying AI for quality control and inspection?
AI delivers multiples improvements in quality control:
- Automated visual inspection powered by deep learning has far higher defect detection rates than human inspectors.
- Predictive maintenance algorithms reduce equipment failures that lead to defects.
- Reinforcement learning enables robots to optimize assembly procedures, avoiding human errors.
- Earlier defect detection and prevention results in less waste, scrap, and returns.
- Higher quality products have longer useful lifespans, reducing the resources needed to replace them frequently.
How can manufacturers start tracking their sustainability metrics using AI?
Manufacturers can leverage AI for sustainability tracking by:
- Using IoT sensors and cloud analytics to collect real-time data like energy use, waste levels, and hazards.
- Building datasets with contextualized sensor data to train AI models to derive insights.
- Using natural language generation algorithms to turn IoT data into narrative sustainability reports.
- Evaluating AI carbon accounting tools to calculate emissions across operations and the supply chain.
- Applying techniques like digital twins and simulations to model the environmental impact of changes pre-emptively.
What are some key challenges manufacturers face in adopting AI for sustainability?
Adoption obstacles include:
- Lack of high-quality labeled training data for tasks like defect detection. Capturing and contextualizing sensor data is key.
- Difficulty integrating legacy equipment and IT systems with new connected IoT infrastructure and cloud analytics.
- Cultural resistance from workers fearing job loss from automation, requiring change management.
- Scarcity of AI talent, especially with manufacturing domain expertise. Partnerships are often necessary.
- Difficulty tracking ROI on sustainability improvements to justify AI investments. Managing short-term KPI tradeoffs.
How can manufacturers assemble the data needed to train AI models for sustainability use cases?
Strategies include:
- Conducting a full audit of sensors on existing equipment and assessing connectivity needs.
- Deploying additional IoT sensors to collect missing real-time operational data.
- Building dashboards for engineers to easily label sensor data for tasks like quality control.
- Purchasing datasets like weather data to complement internally captured IoT data.
- Exploring industry data sharing consortiums to obtain valuable collective datasets.
Which manufacturing processes are the best candidates for initial AI sustainability pilots?
Some promising starting points include:
- Using AI vision systems for defect detection on high value components or major pain points.
- Applying AI optimization for energy-intensive processes like painting, drying, melting, or heating.
- Piloting robotic disassembly initially on simpler products with less variety like electronics.
- Leveraging simulation and digital twinning to model emissions for proposed upstream supply chain changes.
What are some key factors determining the ROI from AI sustainability initiatives?
Factors influencing ROI include:
- The emissions intensity of the processes optimized by AI - bigger environmental footprint means more potential impact.
- Cost savings associated with improvements like reduced energy, downtime, and defects.
- Current margin profile - initiatives earning positive ROI may still displace other more profitable activities.
- Data availability - significant investment is often needed to deploy sensors and build models.
- Applicability across multiple sites vs single-site solutions - allows reuse of data and models.
How can manufacturers align AI sustainability initiatives with corporate ESG commitments?
Strategies include:
- Conducting audits to identify operations with the largest environmental footprints to focus AI.
- Setting public emissions reductions targets that AI optimization projects can tangibly help achieve.
- Allocating R&D budget specifically for AI focused on sustainability.
- Adding sustainability metrics to executive incentive plans linked to AI adoption.
- Joining industry groups and policy efforts promoting sustainability via advanced technologies.
- Issuing green bonds earmarked for investing in AI and IoT infrastructure for environmental gains.
- Highlighting AI case studies demonstrating sustainability success in ESG disclosures.
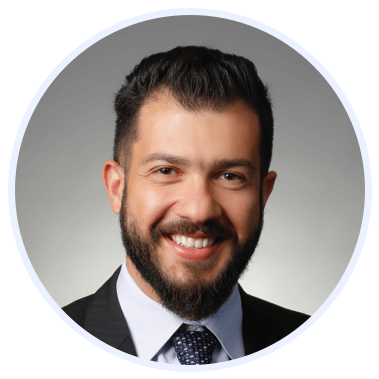
Rasheed Rabata
Is a solution and ROI-driven CTO, consultant, and system integrator with experience in deploying data integrations, Data Hubs, Master Data Management, Data Quality, and Data Warehousing solutions. He has a passion for solving complex data problems. His career experience showcases his drive to deliver software and timely solutions for business needs.