
The oil and gas industry faces unique challenges in maintaining the highest standards of health, safety, and environment (HSE) performance. Despite significant investments in HSE management systems and processes, incidents continue to occur, often with severe consequences for workers, communities, and the environment. As a former CTO and technology leader, I believe that emerging technologies like large language models (LLMs) offer immense potential to transform HSE incident prevention and management in the oil and gas sector.
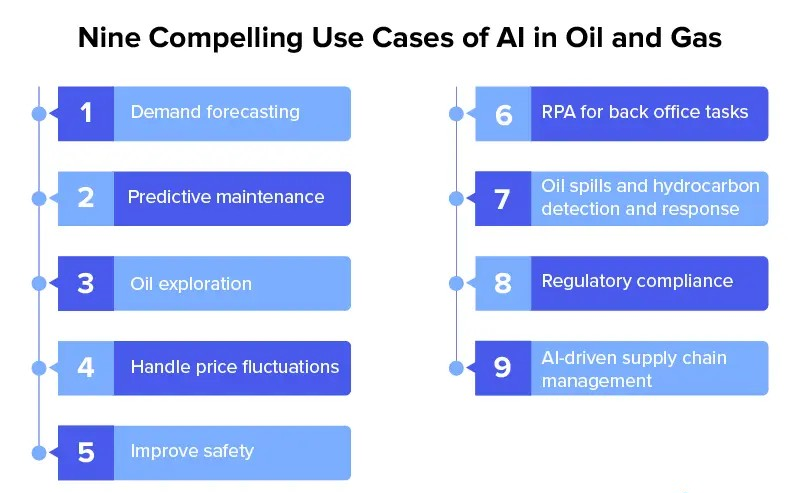
In this blog post, I will explore the applications of LLMs in HSE, share real-world examples of companies making use of these powerful tools, and provide practical insights on how to integrate LLMs into existing HSE management systems. By harnessing the power of AI and natural language processing, we can create safer, more resilient operations and foster a culture of continuous improvement in HSE performance.
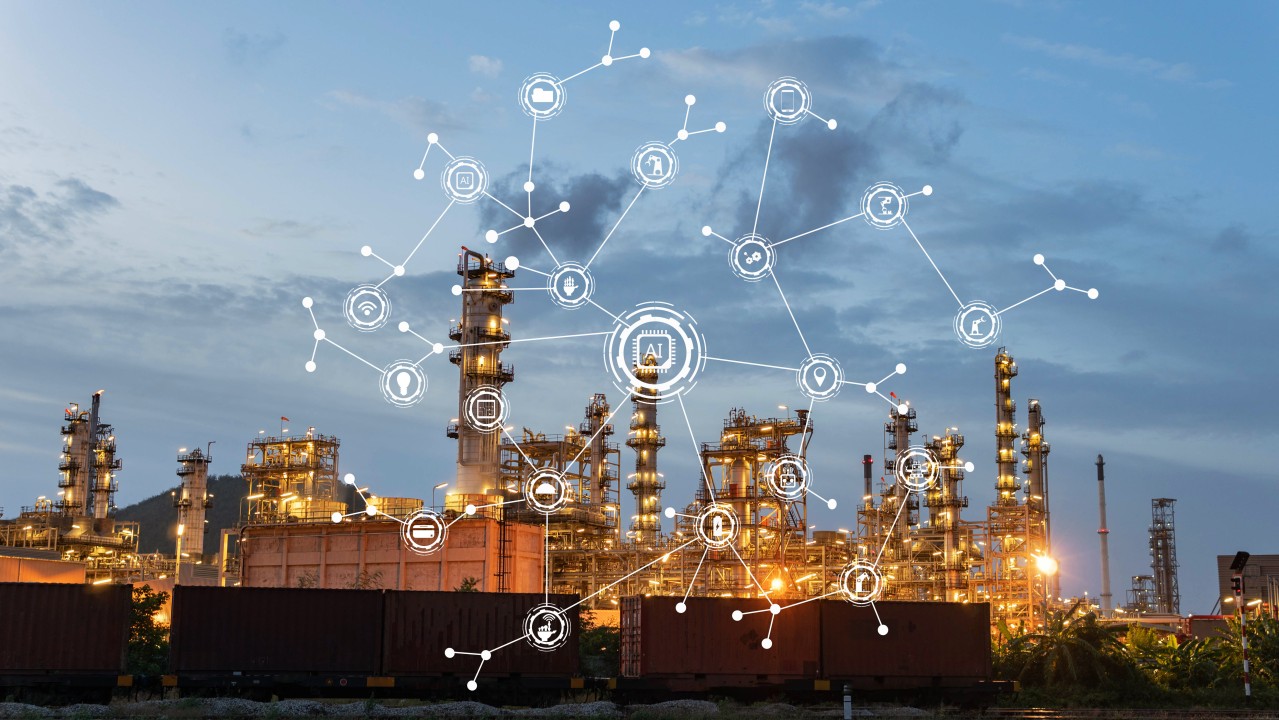
The Importance of HSE in Oil and Gas


The oil and gas industry operates in high-risk environments, with complex facilities, hazardous materials, and challenging operating conditions. Even minor incidents can escalate quickly, leading to serious injuries, fatalities, environmental damage, and reputational harm. According to the International Association of Oil & Gas Producers (IOGP), the industry experienced 25 fatalities and 991 lost work day cases in 2020 alone.
"Safety is not an intellectual exercise to keep us in work. It is a matter of life and death. It is the sum of our contributions to safety management that determines whether the people we work with live or die."
Effective HSE management is not only a moral imperative but also a business necessity. Incidents can result in significant financial losses, regulatory penalties, and project delays. Moreover, stakeholders, including investors, employees, and local communities, increasingly expect companies to demonstrate a strong commitment to HSE excellence.
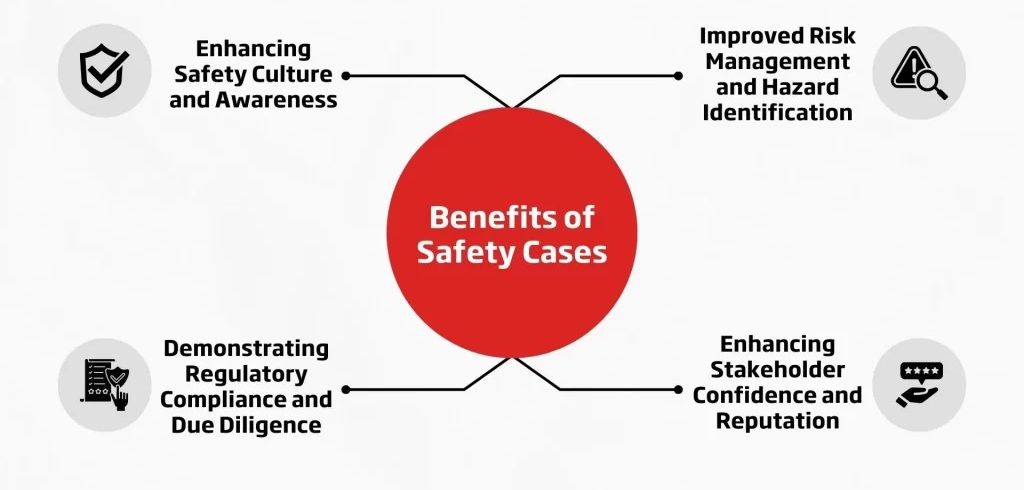
However, preventing incidents in the oil and gas industry is a complex challenge. It requires a proactive, data-driven approach that integrates people, processes, and technology. Traditional HSE management systems, such as behavior-based safety, root cause analysis, and management of change, have proven effective but can be time-consuming and resource-intensive. This is where LLMs can make a significant impact.

The Potential of LLMs in HSE Incident Prevention
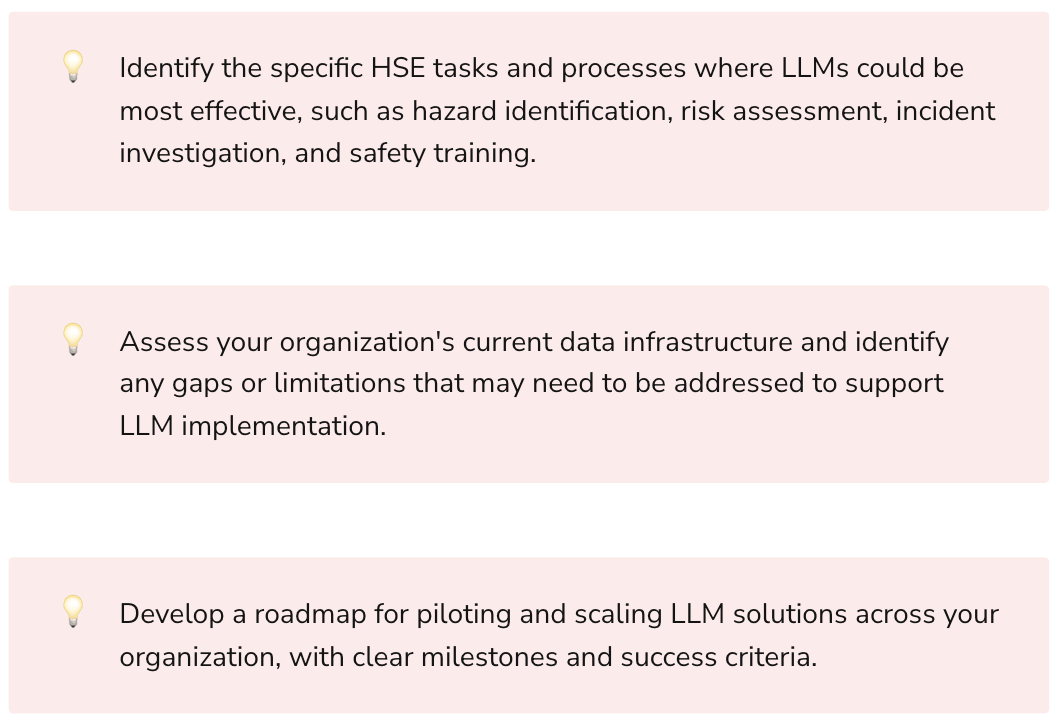
LLMs are a type of AI that can understand, generate, and analyze human language with remarkable accuracy and fluency. By training on vast amounts of text data, LLMs can extract insights, identify patterns, and provide recommendations that would be impossible for humans to uncover manually. In the context of HSE incident prevention, LLMs can be applied to a wide range of tasks, such as:
Hazard Identification: LLMs can analyze incident reports, safety observations, and other unstructured data to identify potential hazards and risk factors that may have been overlooked by traditional methods.
Risk Assessment: By combining data from multiple sources, such as equipment sensors, maintenance records, and environmental conditions, LLMs can provide real-time risk assessments and alert operators to potential incidents before they occur.
Incident Investigation: LLMs can assist in the investigation of incidents by analyzing witness statements, root cause analysis reports, and other relevant documents to identify common themes, contributing factors, and corrective actions.
Safety Training: LLMs can be used to develop personalized safety training programs based on an individual's job role, experience level, and learning style. They can also provide real-time feedback and coaching to reinforce safe behaviors and correct unsafe practices.
Knowledge Management: LLMs can help capture and disseminate safety knowledge across the organization by automatically categorizing and tagging content, generating summaries and FAQs, and recommending relevant information to users based on their search queries.
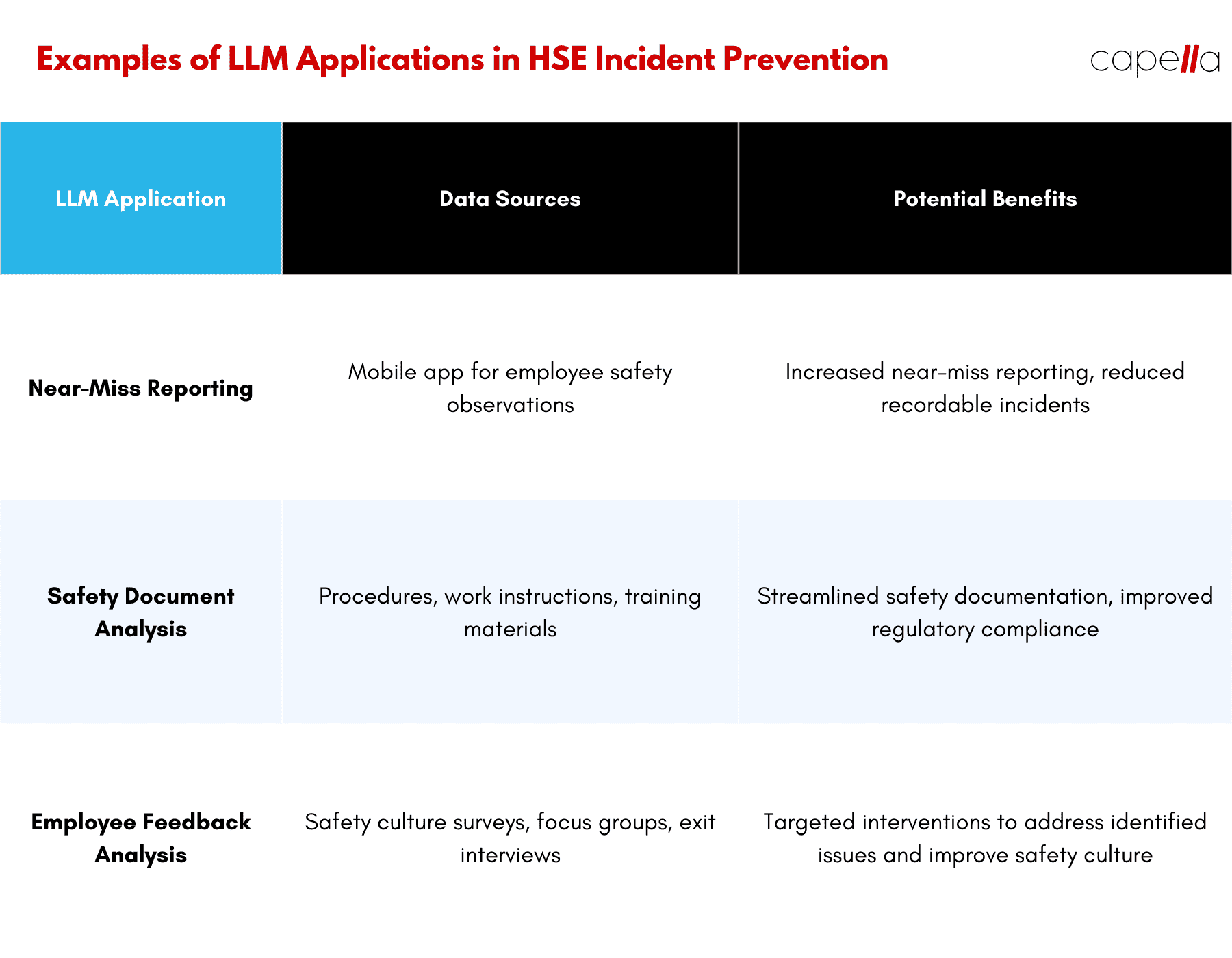
Real-World Examples and Case Studies


Several oil and gas companies have already begun to explore the potential of LLMs in HSE incident prevention. Here are a few examples:
Near-Miss Reporting: A major oil and gas company implemented an LLM-based system for near-miss reporting, which allowed employees to submit safety observations via a mobile app. The LLM analyzed the reports in real-time, categorized them by hazard type and severity, and alerted relevant stakeholders for follow-up action. As a result, the company saw a 50% increase in near-miss reporting and a 25% reduction in recordable incidents.[3]
Safety Document Analysis: Another company used LLMs to analyze a large corpus of safety documents, including procedures, work instructions, and training materials. The LLM identified inconsistencies, gaps, and outdated information, which were then updated to ensure alignment with current best practices. This initiative helped streamline the company's safety documentation and improve compliance with regulatory requirements.
Employee Feedback Analysis: A third company deployed an LLM to analyze employee feedback from safety culture surveys, focus groups, and exit interviews. The LLM uncovered key themes and sentiment trends, such as concerns about management commitment to safety, inadequate resources for training, and perceived pressure to prioritize production over safety. Based on these insights, the company developed targeted interventions to address the identified issues and improve its safety culture.

Benefits of LLM-Based Incident Prevention
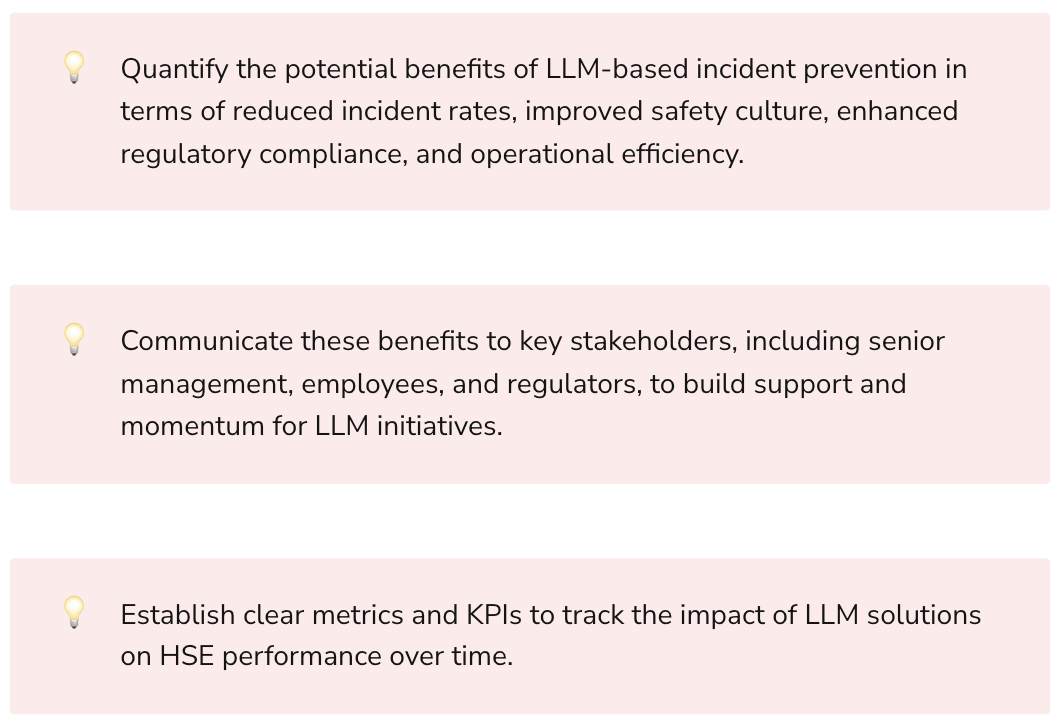

The potential benefits of LLM-based incident prevention in the oil and gas industry are significant, including:
Reduced Incident Rates: By proactively identifying hazards, assessing risks, and providing real-time alerts, LLMs can help prevent incidents from occurring in the first place. Companies that have implemented LLM-based systems have reported significant reductions in recordable incidents and lost time injuries.[4]
Improved Safety Culture: LLMs can help foster a culture of safety by engaging employees in the incident prevention process, providing personalized training and feedback, and demonstrating management commitment to HSE excellence. By making safety a priority at all levels of the organization, companies can create a more resilient and accountable workforce.
Enhanced Regulatory Compliance: LLMs can help companies stay ahead of evolving regulatory requirements by automatically monitoring changes to standards and guidelines, identifying gaps in compliance, and generating audit-ready documentation. By proactively managing compliance, companies can avoid costly penalties and reputational damage.
Operational Efficiency: LLMs can streamline HSE management processes by automating routine tasks, such as incident reporting, data entry, and document management. This can free up valuable time and resources for more strategic initiatives, such as risk assessment and continuous improvement.

Data Requirements and Technical Considerations

To effectively implement LLMs for HSE incident prevention, companies must have access to high-quality, diverse, and relevant data. This data can come from various sources, such as:
- Incident reports and investigations
- Safety observations and near-miss reports
- Equipment sensors and maintenance records
- Employee training records and certifications
- Regulatory standards and industry best practices
- Social media and news feeds related to safety incidents
However, collecting, integrating, and preparing this data for LLM training can be a significant challenge. Companies must ensure that the data is accurate, complete, and representative of the various scenarios and contexts in which incidents can occur. They must also address issues related to data privacy, security, and governance, especially when dealing with sensitive information such as employee health records.
From a technical perspective, implementing LLMs for HSE incident prevention requires a robust infrastructure for data storage, processing, and analysis. Companies must select the appropriate LLM architecture and training approach based on their specific use case, data characteristics, and performance requirements. They must also consider factors such as scalability, latency, and interpretability when deploying LLMs in production environments.

Strategies for Integrating LLMs into Existing HSE Management Systems


To realize the full potential of LLMs in HSE incident prevention, companies must integrate them into their existing HSE management systems and processes. This requires a holistic approach that considers the following strategies:
Alignment with HSE Objectives: LLMs should be aligned with the company's overall HSE objectives and key performance indicators (KPIs). This ensures that the insights generated by LLMs are actionable and relevant to the company's priorities.
Stakeholder Engagement: Engaging key stakeholders, such as HSE professionals, operations personnel, and data scientists, is critical for the success of LLM initiatives. Companies must foster a culture of collaboration and communication to ensure that LLMs are developed and deployed in a way that meets the needs of all stakeholders.
Change Management: Implementing LLMs may require significant changes to existing processes, roles, and responsibilities. Companies must develop a clear change management plan that includes training, communication, and support for employees affected by the transition.
Continuous Improvement: LLMs are not a one-time solution but rather a continuous process of learning and adaptation. Companies must establish a feedback loop that allows them to monitor the performance of LLMs, identify areas for improvement, and update the models based on new data and insights.
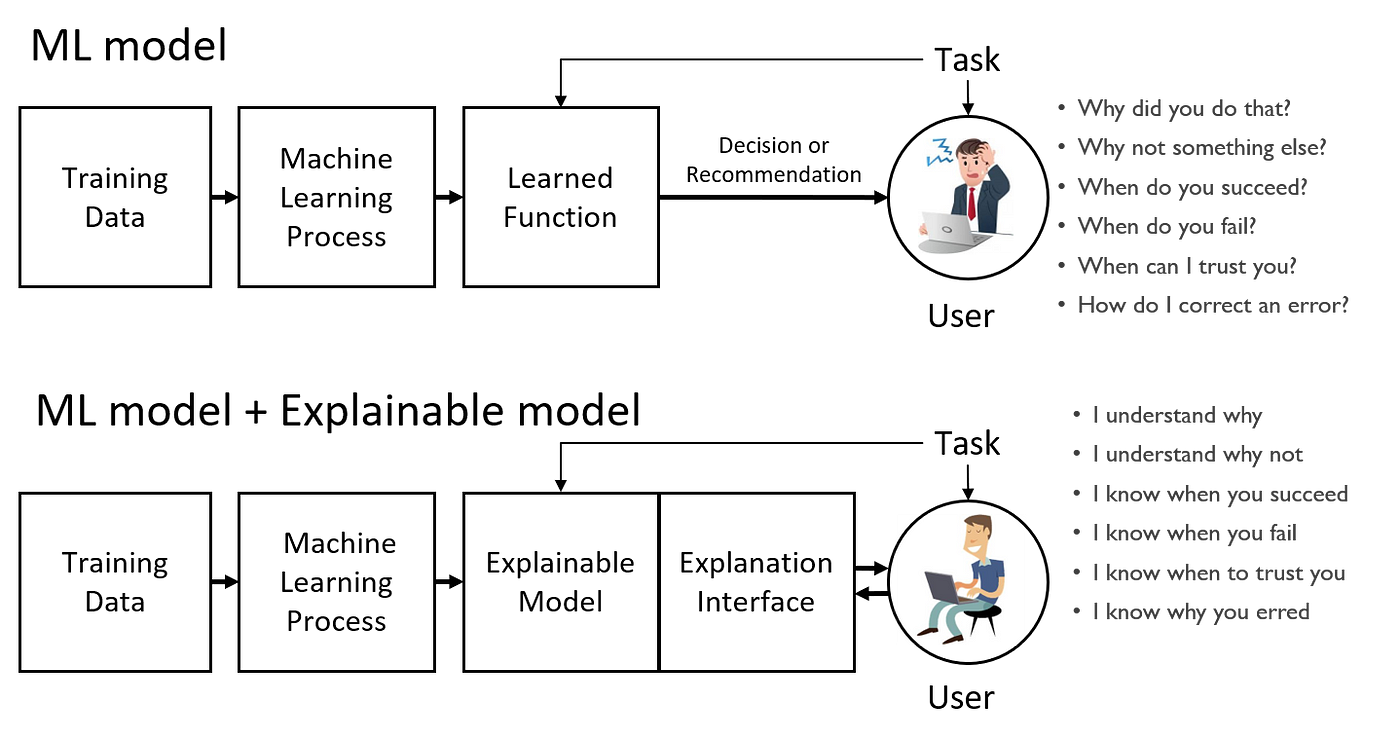
Best Practices for Developing and Deploying LLMs
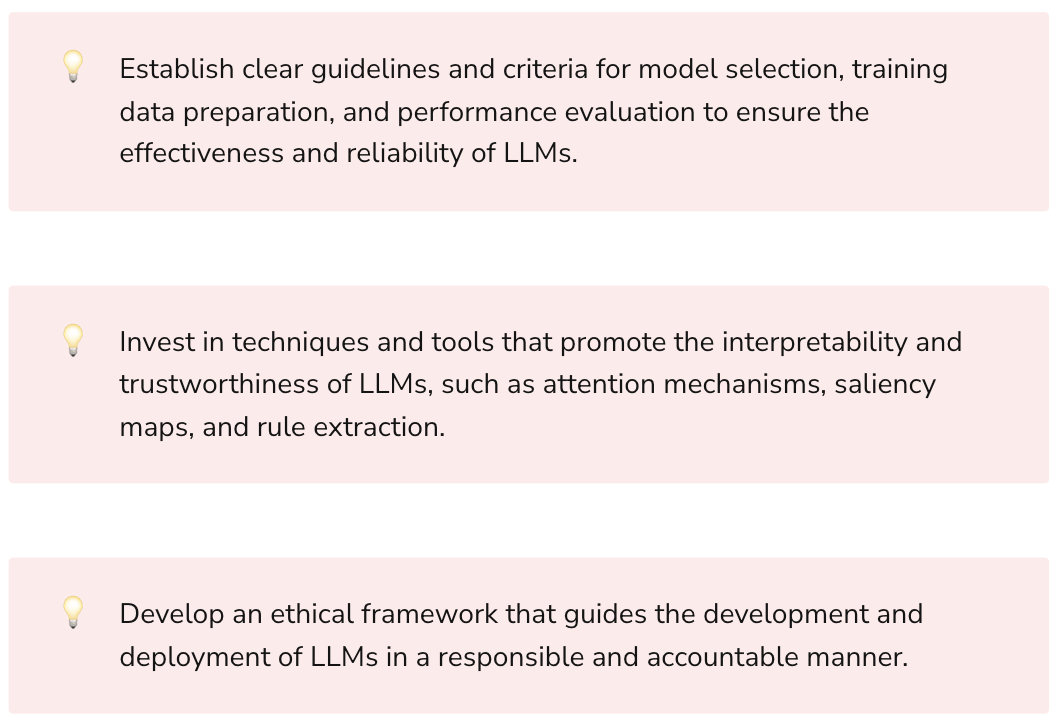
To ensure the effectiveness and reliability of LLMs for HSE incident prevention, companies should follow these best practices:
Model Selection: Choose an LLM architecture that is appropriate for the specific use case and data characteristics. Consider factors such as model size, training time, and inference speed.
Training Data Preparation: Ensure that the training data is diverse, representative, and high-quality. Use techniques such as data augmentation, cross-validation, and stratified sampling to improve the robustness and generalizability of the model.
Performance Evaluation: Evaluate the performance of LLMs using relevant metrics such as accuracy, precision, recall, and F1 score. Use techniques such as confusion matrices and ROC curves to visualize the model's performance and identify areas for improvement.
Interpretability and Trust: Use techniques such as attention mechanisms, saliency maps, and rule extraction to make LLMs more interpretable and trustworthy. This can help build confidence in the model's predictions and facilitate collaboration between data scientists and domain experts.
Ethical Considerations: Ensure that LLMs are developed and deployed in an ethical and responsible manner. Consider issues such as bias, fairness, transparency, and accountability when designing and implementing LLM solutions.
The Role of Business Leaders
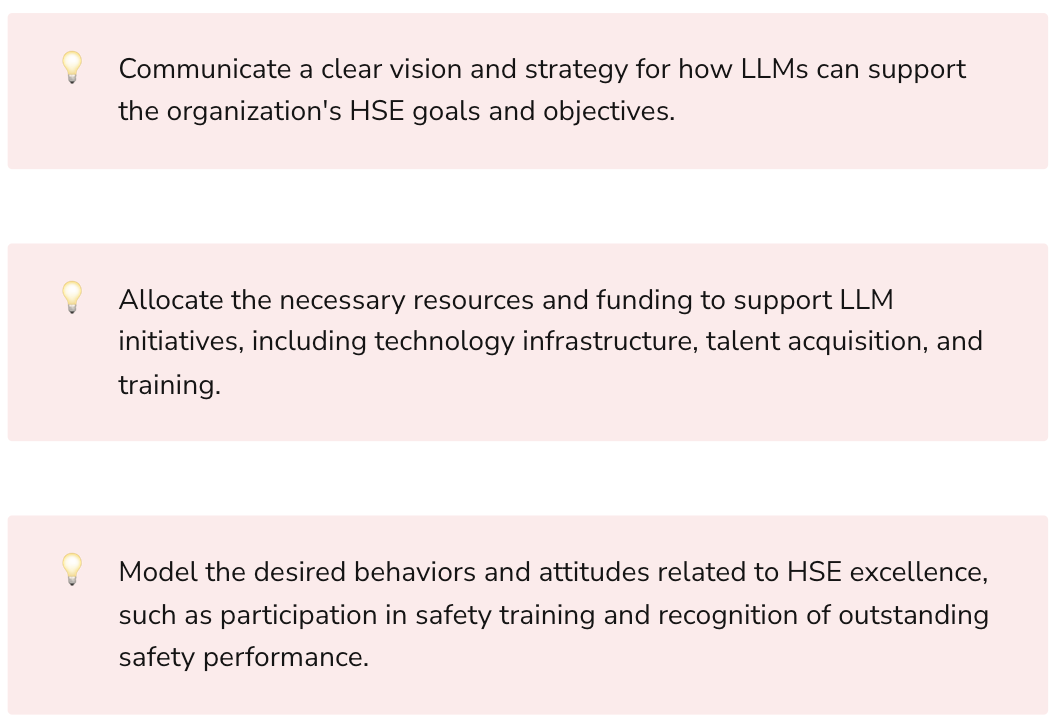

Business leaders play a critical role in driving the adoption of LLMs and digital technologies for HSE improvement. They must provide the vision, resources, and support necessary to create a culture of safety and innovation. This includes:
Setting Clear Expectations: Leaders must set clear expectations for HSE performance and communicate the importance of incident prevention to all employees. They must also hold themselves and their teams accountable for meeting these expectations.
Investing in Technology: Leaders must invest in the necessary technology infrastructure and talent to support LLM initiatives. This includes providing adequate funding, resources, and training for data scientists, HSE professionals, and other key stakeholders.
Fostering Collaboration: Leaders must foster a culture of collaboration and knowledge sharing across the organization. They must break down silos between departments and encourage cross-functional teams to work together on HSE initiatives.
Leading by Example: Leaders must demonstrate their commitment to HSE excellence through their actions and behaviors. They must model safe practices, participate in safety training and observations, and recognize employees who demonstrate outstanding safety performance.
Conclusion
The oil and gas industry faces significant challenges in maintaining the highest standards of HSE performance. However, emerging technologies like LLMs offer immense potential to transform HSE incident prevention and management. By harnessing the power of AI and natural language processing, companies can create safer, more resilient operations and foster a culture of continuous improvement.
To realize this potential, companies must take a holistic approach that integrates LLMs into their existing HSE management systems and processes. They must engage key stakeholders, develop a clear change management plan, and establish a feedback loop for continuous improvement. Business leaders must also provide the vision, resources, and support necessary to create a culture of safety and innovation.
I am excited about the potential of LLMs to transform HSE incident prevention in the oil and gas industry. By drawing on our expertise in data management and AI, we are committed to helping our clients unlock the full potential of these powerful tools. Together, we can create a safer, more sustainable future for our industry and the communities we serve.
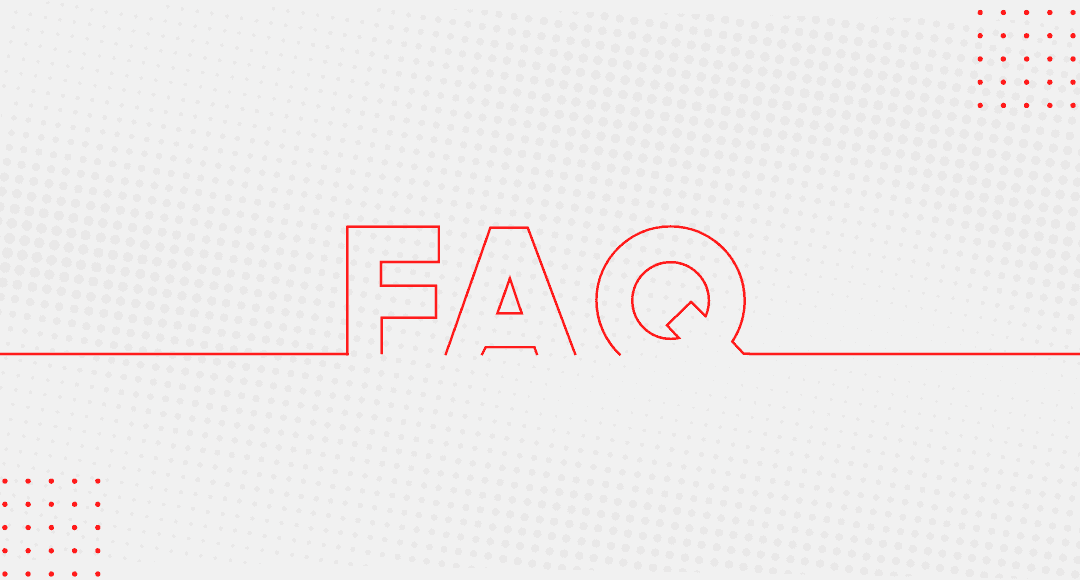
What are Large Language Models (LLMs) and how do they apply to HSE incident prevention?
Large Language Models (LLMs) are advanced AI systems that can understand, generate, and analyze human language. In the context of HSE incident prevention, LLMs can be used to automatically identify hazards, assess risks, investigate incidents, and provide personalized safety training based on vast amounts of textual data.
How can LLMs help improve safety culture in the oil and gas industry?
LLMs can help foster a strong safety culture by engaging employees in the incident prevention process, providing targeted feedback and guidance, and demonstrating management's commitment to HSE excellence. By making safety a data-driven and proactive endeavor, LLMs can help create a more resilient and accountable workforce.
What are some real-world examples of LLM applications in HSE management?
Some examples include:
- Using LLMs to analyze near-miss reports and identify potential hazards
- Applying LLMs to safety document analysis to ensure consistency and compliance
- Using LLMs to gain insights from employee feedback and improve safety culture
What kind of data is required to train LLMs for HSE incident prevention?
Training LLMs for HSE incident prevention requires a wide range of data sources, such as incident reports, safety observations, equipment maintenance records, employee training records, and regulatory standards. The data must be accurate, complete, and representative of the various scenarios and contexts in which incidents can occur.
How can companies integrate LLMs into their existing HSE management systems?
Integrating LLMs into existing HSE management systems requires a holistic approach that considers factors such as alignment with HSE objectives, stakeholder engagement, change management, and continuous improvement. Companies should start with pilot projects and gradually scale up LLM deployment based on lessons learned and business impact.
What are some best practices for developing and deploying LLMs in HSE?
Some best practices include:
- Selecting appropriate model architectures and training approaches
- Ensuring data quality, diversity, and representativeness
- Evaluating model performance using relevant metrics and techniques
- Promoting model interpretability and trustworthiness
- Addressing ethical considerations such as bias, fairness, and transparency
What is the role of business leaders in driving LLM adoption for HSE improvement?
Business leaders play a crucial role in setting the vision, allocating resources, fostering collaboration, and leading by example when it comes to LLM adoption for HSE improvement. They must communicate a clear strategy, provide necessary support, and demonstrate their commitment to HSE excellence through their actions and decisions.
How can LLMs help companies comply with HSE regulations and standards?
LLMs can assist companies in staying up-to-date with evolving HSE regulations and standards by automatically analyzing regulatory documents, identifying compliance gaps, and generating audit-ready reports. They can also help streamline and standardize HSE processes across the organization to ensure consistent compliance.
What are some potential challenges or risks associated with implementing LLMs in HSE?
Some potential challenges or risks include:
- Ensuring data quality, privacy, and security
- Addressing the potential for bias or errors in LLM predictions
- Managing the change impact on workforce roles and processes
- Ensuring the interpretability and explainability of LLM decisions
- Balancing the benefits and costs of LLM implementation
How can companies measure the success and ROI of LLM-based HSE incident prevention initiatives?
Companies can measure the success and ROI of LLM-based HSE incident prevention initiatives by tracking key performance indicators such as incident rates, near-miss reporting, safety audit scores, and employee engagement. They should also consider the financial benefits of reduced incidents, such as lower insurance premiums, legal costs, and production downtime. Regular review and adjustment of LLM initiatives based on performance data is crucial for long-term success.
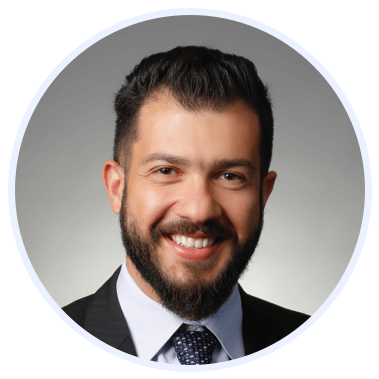
Rasheed Rabata
Is a solution and ROI-driven CTO, consultant, and system integrator with experience in deploying data integrations, Data Hubs, Master Data Management, Data Quality, and Data Warehousing solutions. He has a passion for solving complex data problems. His career experience showcases his drive to deliver software and timely solutions for business needs.