

In today's fast-paced, data-driven world, it's impossible to overstate the importance of managing and leveraging the vast amounts of data generated by large enterprises. Enter Master Data Management (MDM)—a cornerstone of data strategy that has helped organizations maintain a consistent, accurate, and complete view of their critical data. But, as the data landscape evolves, MDM must evolve with it. The key to this evolution? Embedded analytics. This powerful tool unlocks new insights, drives business value, and transforms the way organizations use their data. In this article, we'll dive into the exciting world of embedded analytics in MDM, exploring its game-changing impact and how it can help executives and decision-makers in large enterprises stay ahead of the curve.
Table of Contents
- The Rise of Embedded Analytics
- The Evolution of MDM
- Why Embedded Analytics Matters for MDM
- Key Benefits of Embedded Analytics in MDM
- Case Studies: Embedded Analytics in Action
- Looking Ahead: The Future of MDM and Embedded Analytics
- Conclusion

1. The Rise of Embedded Analytics
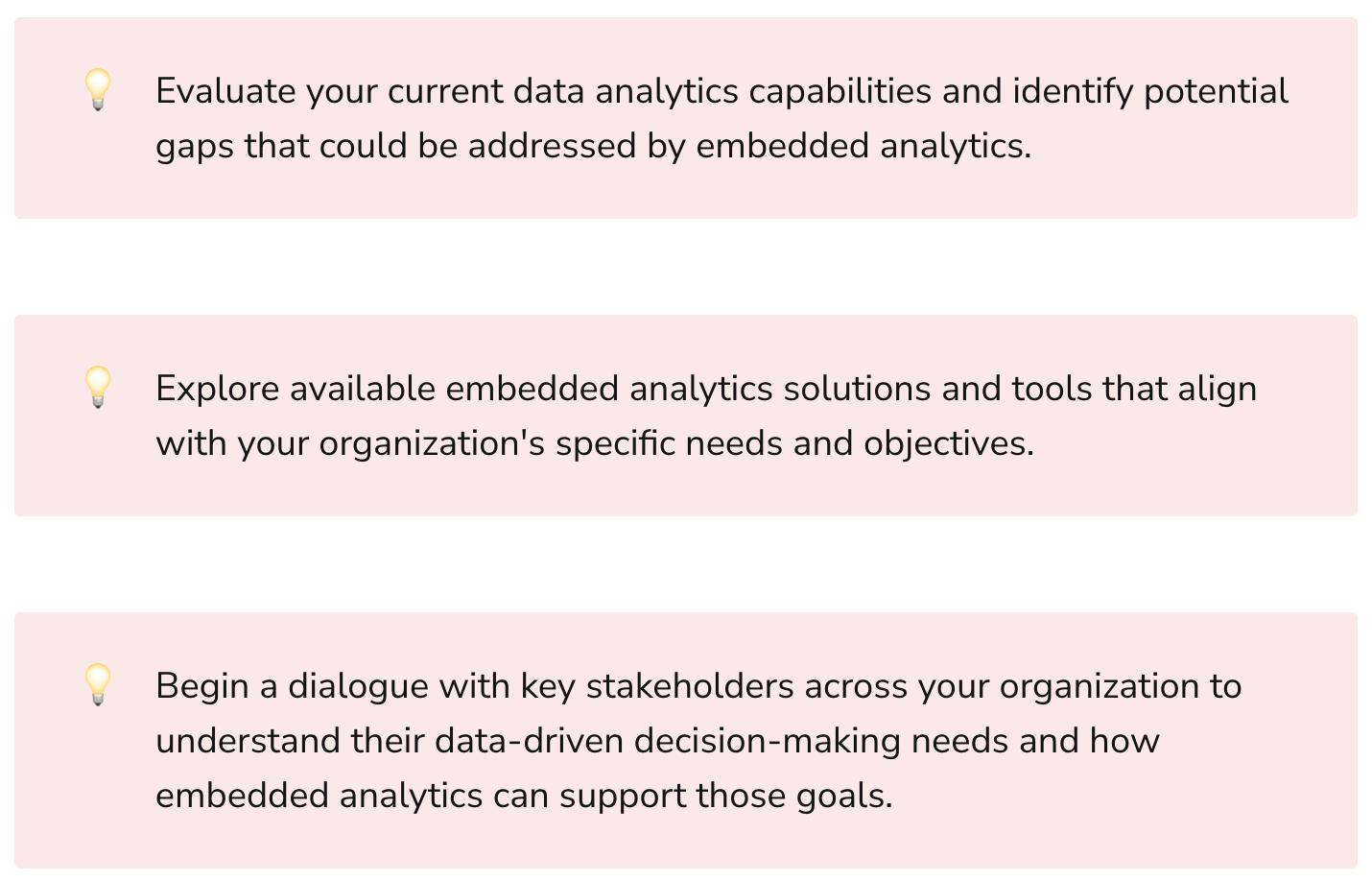
Embedded analytics refers to the integration of analytical capabilities, such as data visualization, predictive modeling, and machine learning, directly into enterprise applications and business processes. By incorporating analytics into the very fabric of an organization's operations, embedded analytics empowers users to access data-driven insights without leaving the context of their everyday tasks.
According to Gartner, by 2025, 70% of new business applications will have embedded analytics, up from just 35% in 2020. This rapid growth is driven by a combination of factors, including advances in AI and machine learning, the rise of cloud-based infrastructure, and increasing demand for real-time decision-making.
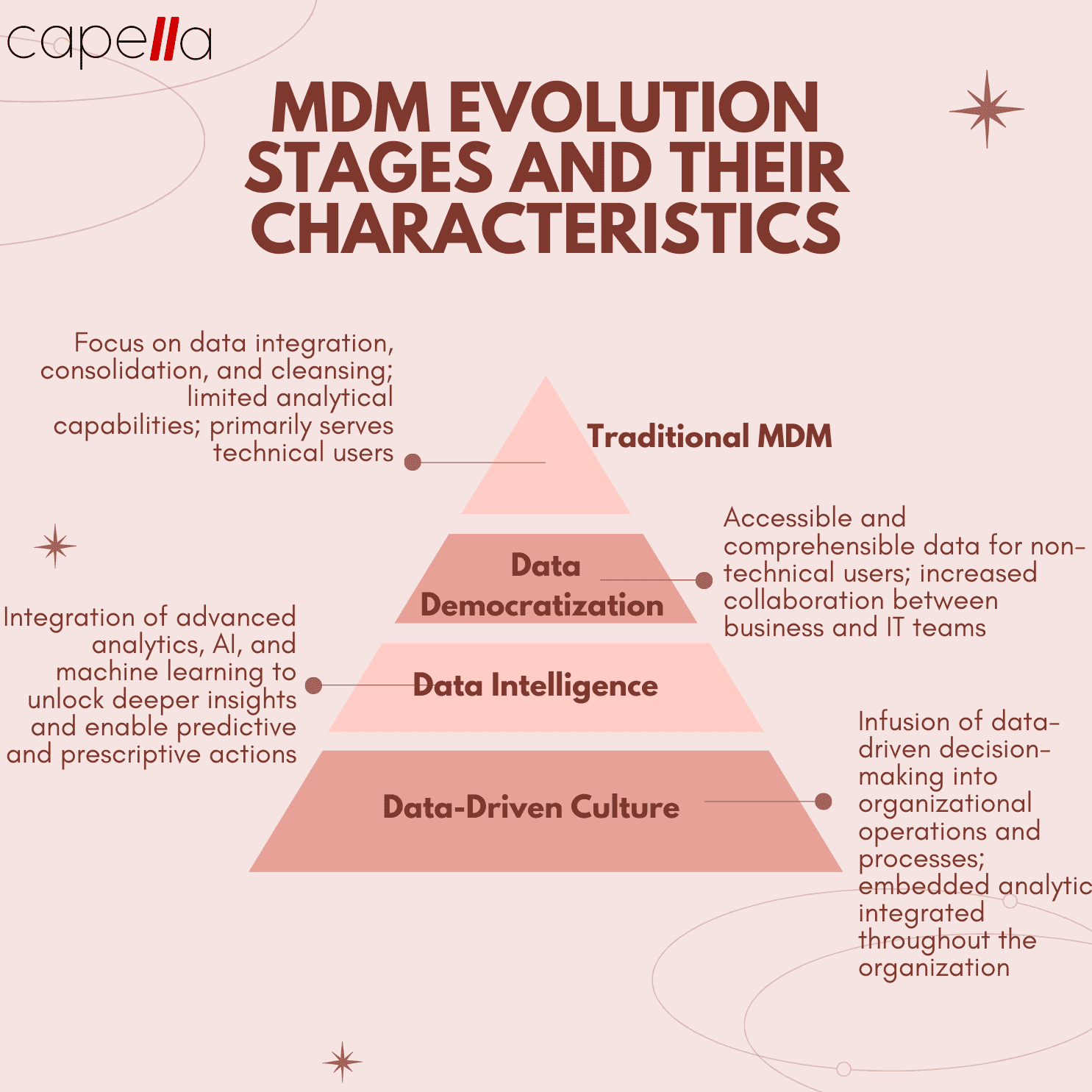
2. The Evolution of MDM

MDM has been instrumental in helping organizations manage, govern, and maintain the quality of their critical master data. However, traditional MDM solutions have focused primarily on data integration, consolidation, and cleansing. As organizations look to leverage their data more effectively, the scope and capabilities of MDM must evolve to meet these demands.
The future of MDM is centered around three key trends:
- Data Democratization: Making data accessible and comprehensible to non-technical users, enabling them to derive insights and drive decision-making.
- Data Intelligence: Leveraging advanced analytics, AI, and machine learning to unlock deeper insights and drive predictive and prescriptive actions.
- Data-Driven Culture: Embedding data-driven decision-making into the very fabric of an organization's operations and processes.
Embedded analytics plays a crucial role in enabling this evolution, transforming MDM from a static data management tool into a dynamic, insight-generating platform.

3. Why Embedded Analytics Matters for MDM

For large enterprises, the sheer volume and complexity of data can make it difficult to derive actionable insights. This challenge is compounded by the fact that data is often siloed across various systems and applications. MDM has been essential for creating a single source of truth for critical master data, but its potential goes beyond simple data consolidation.
By incorporating embedded analytics into MDM, organizations can unlock the true value of their data and drive more informed, data-driven decision-making. Embedded analytics can help organizations identify patterns, trends, and anomalies in their data, enabling them to make better decisions and optimize their operations.
4. Benefits of Embedded Analytics in MDM
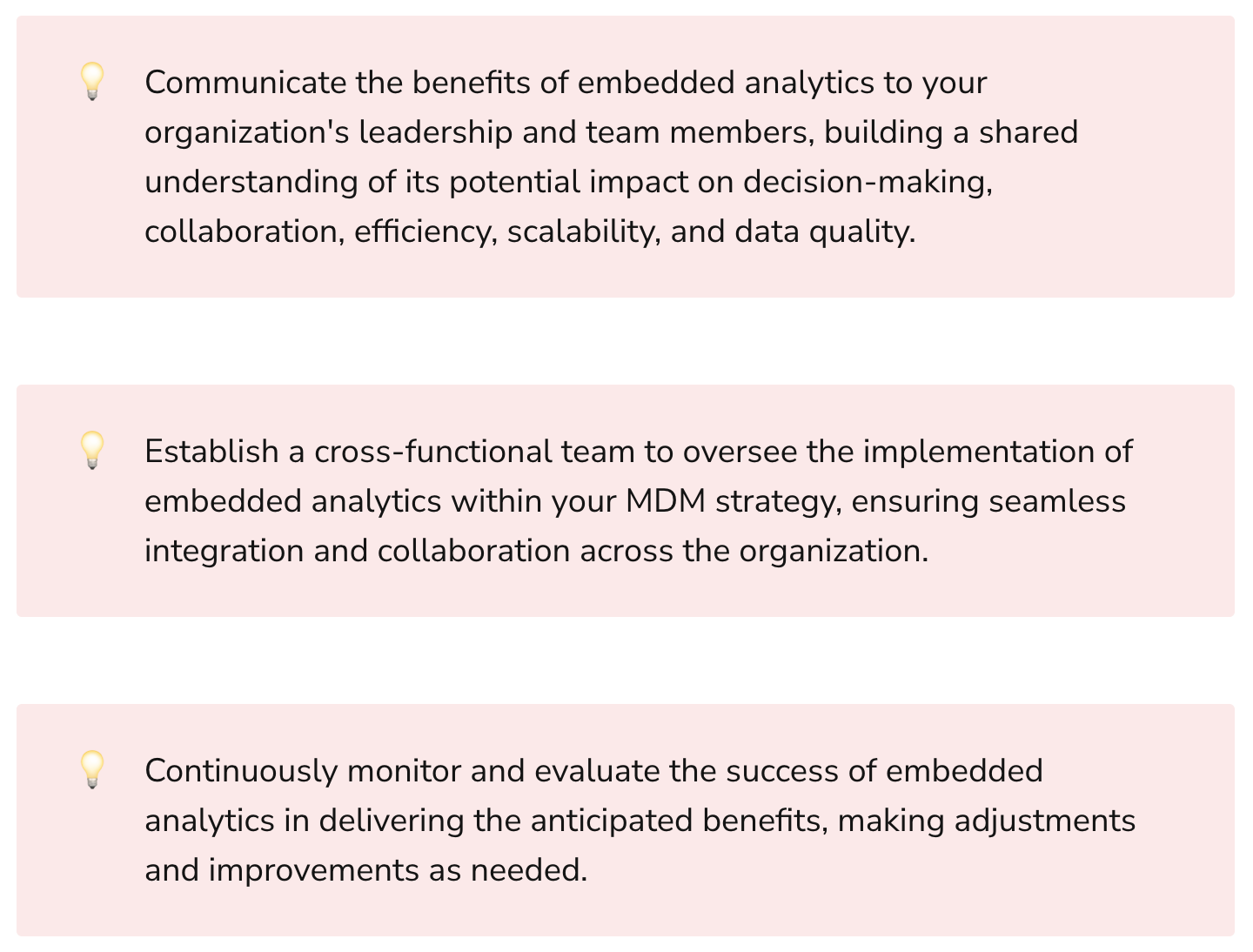
The integration of embedded analytics into MDM brings a range of benefits to large enterprises. Here, we outline some of the most significant advantages:
4.1 Enhanced Decision-Making
By providing real-time insights directly within the context of business processes and applications, embedded analytics enables users to make better-informed decisions. Users can access relevant data and insights as they perform their tasks, allowing them to act quickly and confidently.
4.2 Greater Collaboration
Incorporating embedded analytics into MDM fosters a data-driven culture by encouraging collaboration between business and IT teams. By making data more accessible and comprehensible to non-technical users, embedded analytics breaks down the barriers that have traditionally existed between these two groups, leading to more effective cross-functional collaboration.
4.3 Increased Efficiency
Embedded analytics streamlines the process of accessing and analyzing data, reducing the need for manual data extraction, manipulation, and reporting. This not only saves time but also minimizes the risk of errors associated with manual processes.
4.4 Scalability and Agility
With cloud-based infrastructure becoming increasingly prevalent, embedded analytics can be easily scaled to accommodate growing data volumes and demands. This allows organizations to stay agile and adapt to the ever-changing data landscape.
4.5 Improved Data Quality
By integrating analytics directly into MDM processes, organizations can proactively monitor and maintain data quality. Embedded analytics can help identify data quality issues in real-time, allowing organizations to address them before they impact downstream processes or decision-making.
5. Case Studies: Embedded Analytics in Action
To better understand the transformative potential of embedded analytics in MDM, let's examine a few real-world examples:
5.1 Manufacturing
A global manufacturing company integrated embedded analytics into their MDM solution to optimize their supply chain operations. By analyzing data from multiple sources, they were able to identify patterns and trends in supplier performance, inventory levels, and demand forecasts. This allowed the company to make more informed decisions about production planning, supplier relationships, and inventory management, leading to significant cost savings and improved efficiency.
5.2 Retail
A large retailer incorporated embedded analytics into their MDM platform to gain a deeper understanding of customer behavior and preferences. By consolidating and analyzing data from multiple channels, they were able to develop a comprehensive customer profile that informed targeted marketing campaigns and personalized promotions. As a result, the retailer experienced a significant increase in customer engagement and sales.
5.3 Healthcare
A leading healthcare provider integrated embedded analytics into their MDM solution to improve patient care and streamline operations. By analyzing data from electronic health records, insurance claims, and other sources, the organization gained insights into patient demographics, treatment patterns, and clinical outcomes. These insights informed targeted interventions and resource allocation, leading to improved patient outcomes and operational efficiency.
6. Looking Ahead: The Future of MDM and Embedded Analytics
As organizations continue to recognize the value of data-driven decision-making, the demand for embedded analytics in MDM will only grow. Some key developments to watch in the future include:
- Greater Adoption of AI and Machine Learning: As AI and machine learning technologies continue to advance, their integration into embedded analytics will enable more sophisticated data analysis and predictive capabilities.
- Expanded Use Cases: As embedded analytics becomes more accessible and user-friendly, organizations will find new ways to apply it to their business processes and operations, further driving the transformation of MDM.
- Increased Focus on Data Governance: As the volume and complexity of data grow, organizations will need to invest in robust data governance practices to ensure the accuracy and reliability of their data. Embedded analytics can play a vital role in supporting these efforts by providing real-time visibility into data quality issues and helping to maintain compliance with data protection regulations.
- Advancements in Data Visualization: As embedded analytics matures, we can expect to see further advancements in data visualization techniques, enabling users to more easily interpret complex data and extract actionable insights.
- Edge Analytics: With the rise of the Internet of Things (IoT) and edge computing, embedded analytics will increasingly be applied at the edge, enabling real-time analysis and decision-making on data generated by devices and sensors.
7. Conclusion
The future of Master Data Management is being redefined by the integration of embedded analytics. As organizations seek to leverage their data more effectively, MDM must evolve to meet these demands. Embedded analytics not only helps organizations unlock the true value of their data but also drives more informed, data-driven decision-making.
By embracing embedded analytics in MDM, large enterprises can experience significant benefits, including enhanced decision-making, greater collaboration, increased efficiency, improved scalability and agility, and better data quality. The integration of AI, machine learning, and advanced data visualization techniques will further drive the transformation of MDM, empowering organizations to stay ahead of the curve in an increasingly competitive and data-driven world.
As an executive or decision-maker in a large enterprise, it's crucial to recognize the potential of embedded analytics in MDM and invest in the technologies and processes that will enable your organization to harness the power of data. By doing so, you'll be well-positioned to drive innovation, growth, and success in the data-driven era.
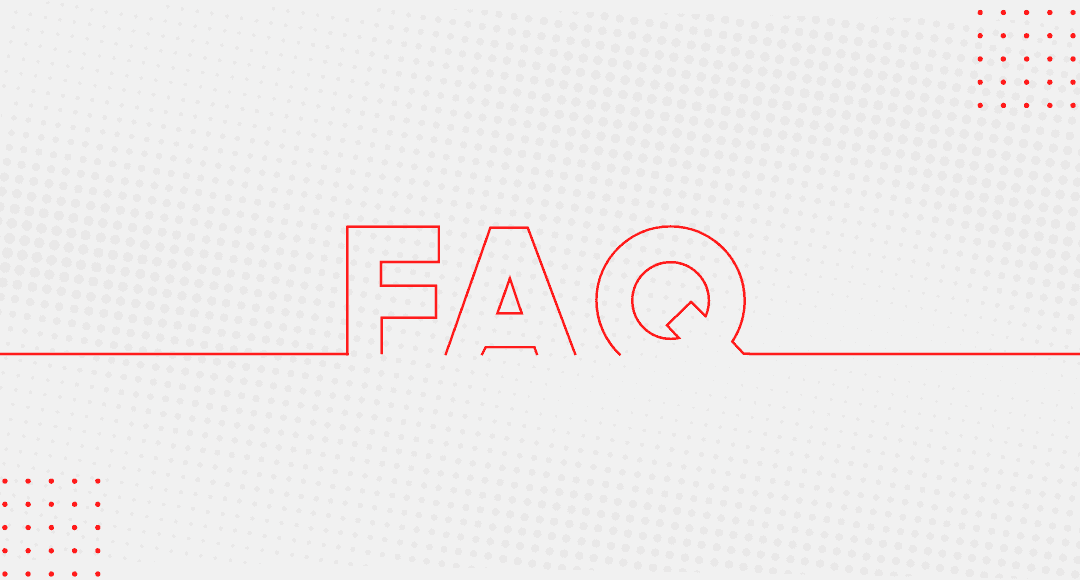
1. What is Master Data Management (MDM)?
Master Data Management (MDM) is a comprehensive approach to managing, governing, and maintaining the quality of an organization's critical data. MDM helps ensure that master data—such as customer, product, or vendor information—is consistent, accurate, and complete across various systems, applications, and processes.
2. What is embedded analytics?
Embedded analytics refers to the integration of analytical capabilities, such as data visualization, predictive modeling, and machine learning, directly into enterprise applications and business processes. By incorporating analytics within the context of users' day-to-day tasks, embedded analytics allows for real-time access to data-driven insights and streamlined decision-making.
3. How is embedded analytics changing the future of MDM?
Embedded analytics is driving the evolution of MDM by transforming it from a static data management tool into a dynamic, insight-generating platform. By integrating advanced analytics, AI, and machine learning capabilities, embedded analytics empowers organizations to unlock deeper insights, improve data quality, and drive data-driven decision-making across the enterprise. This transformation is enabled by three key trends: data democratization, data intelligence, and data-driven culture.
4. What are the benefits of integrating embedded analytics into MDM?
There are several key benefits to integrating embedded analytics into MDM:
- Enhanced decision-making: Real-time insights enable users to make better-informed decisions.
- Greater collaboration: Accessible and comprehensible data fosters collaboration between business and IT teams.
- Increased efficiency: Streamlined data access and analysis reduce manual processes and errors.
- Scalability and agility: Cloud-based infrastructure allows for easy scaling to accommodate growing data volumes and demands.
- Improved data quality: Proactive monitoring and maintenance of data quality within MDM processes.
5. How can large enterprises successfully adopt embedded analytics in MDM?
Large enterprises can adopt embedded analytics in MDM by following these steps:
- Assess the organization's MDM maturity and readiness for adopting advanced analytics, AI, and machine learning technologies.
- Develop a roadmap for evolving the MDM strategy to include data democratization, data intelligence, and data-driven culture.
- Identify potential use cases and applications within the organization that could benefit from the integration of embedded analytics.
- Implement embedded analytics solutions that align with the organization's specific needs and objectives.
- Establish key performance indicators (KPIs) to measure the impact of embedded analytics on decision-making, efficiency, and data quality.
6. Are there any industries or sectors that are particularly well-suited for embedded analytics in MDM?
While embedded analytics can benefit organizations across various industries, some sectors that stand out for their potential to leverage embedded analytics in MDM include:
- Manufacturing: Optimizing supply chain operations by analyzing data from multiple sources, including supplier performance, inventory levels, and demand forecasts.
- Retail: Gaining a deeper understanding of customer behavior and preferences by consolidating and analyzing data from multiple channels.
- Healthcare: Improving patient care and streamlining operations by analyzing data from electronic health records, insurance claims, and other sources.
7. What are some challenges organizations may face when implementing embedded analytics in MDM?
Organizations may encounter several challenges when implementing embedded analytics in MDM, including:
- Integrating embedded analytics with existing MDM solutions, applications, and business processes.
- Ensuring data security and compliance with data protection regulations.
- Managing the change associated with adopting new analytics tools and processes, including training and support for users.
- Balancing the need for customization and flexibility with the desire for standardization and consistency.
8. How can organizations measure the success of embedded analytics in MDM?
Organizations can measure the success of embedded analytics in MDM by tracking key performance indicators (KPIs) related to decision-making, collaboration, efficiency, scalability, and data quality. Some examples of success metrics include:
- Time required to make decisions and the quality of those decisions before and after implementing embedded analytics.
- Level of engagement between business and IT teams, including cross-functional meetings and joint projects.
- Time savings resulting from streamlined data access and analysis processes.
- Ability to accommodate growing data volumes and user demands, as well as the speed of adapting to changes in the data landscape.
- Data quality indicators, such as error rates and data completeness, before and after implementing embedded analytics.
9. What factors should organizations consider when selecting an embedded analytics solution for MDM?
When selecting an embedded analytics solution for MDM, organizations should consider the following factors:
- Integration: Ease of integration with existing MDM solutions, applications, and business processes.
- Scalability: Ability to scale with growing data volumes and user demands; compatibility with cloud-based infrastructure.
- User Experience: Intuitive interfaces and features designed for both technical and non-technical users.
- Customization: Flexibility to customize analytical capabilities and visualizations to meet organization-specific needs.
- Security and Compliance: Robust security features and adherence to data protection regulations.
10. How can organizations foster a data-driven culture and support the adoption of embedded analytics in MDM?
Organizations can foster a data-driven culture and support the adoption of embedded analytics in MDM by:
- Communicating the benefits of embedded analytics to the organization's leadership and team members, building a shared understanding of its potential impact on decision-making, collaboration, efficiency, scalability, and data quality.
- Providing training and support for users to help them effectively leverage embedded analytics tools and capabilities.
- Encouraging cross-functional collaboration and breaking down barriers between business and IT teams.
- Continuously monitoring and evaluating the success of embedded analytics in delivering the anticipated benefits, making adjustments and improvements as needed.
- Sharing success stories and lessons learned from early embedded analytics initiatives with the broader organization to build momentum and support for continued investment and expansion.
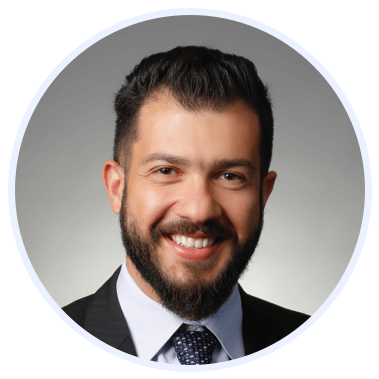
Rasheed Rabata
Is a solution and ROI-driven CTO, consultant, and system integrator with experience in deploying data integrations, Data Hubs, Master Data Management, Data Quality, and Data Warehousing solutions. He has a passion for solving complex data problems. His career experience showcases his drive to deliver software and timely solutions for business needs.