
However, data is only as valuable as it is accurate. Inaccurate data can lead to bad decision-making, unsatisfied customers, and decreased productivity. This is why data quality is so important.
In this article, we will explore what data quality is, why it matters, and some tips to improve it.
What Is Data Quality?
Data quality is a term used to describe the condition of data. It is often used to measure how accurate and consistent data is.
The quality of data can be affected by many factors, such as data entry errors, incorrect or missing data, and outdated information.
Data Entry Errors
These are the most common causes of poor data quality. They can be caused by human error, such as when data is entered into a system incorrectly. Data entry errors can also be caused by technical issues, such as when a system is not able to read data correctly.
Incorrect or Missing Data
This is another common cause of poor data quality. This can happen when data is not collected correctly, or when it is not entered into a system properly. Incorrect or missing data can also be caused by errors in the way data is processed.
Outdated Information
This is the other major cause of poor data quality. This can happen when data is not updated regularly, or when it is not kept in sync with other systems. Outdated information can also be caused by errors in the way data is stored.
How Do You Measure Data Quality?
Data quality is often measured by how accurate and consistent data is, using a set of standards known as Data Quality Dimensions. These dimensions include:
Accuracy
This refers to how close data is to the truth. In other words, it measures how close data is to reality.
Completeness
Simply put, it refers to the percentage of data that is complete. This measures how much data is missing.
Timeliness
Timeliness refers to how up-to-date data is. In other words, it measures how current data is.
Uniformity
Uniformity refers to how consistent data is. Simply put, it measures how similar data is across different systems.
Validity
This refers to whether data is valid. In other words, it measures whether data is accurate and complete.
Uniqueness
Uniqueness refers to how often data is duplicated. In simple terms, it measures how many copies of data are in a system.
There may be other factors that contribute to data quality, but if your data meets these six criteria, it is high-quality.
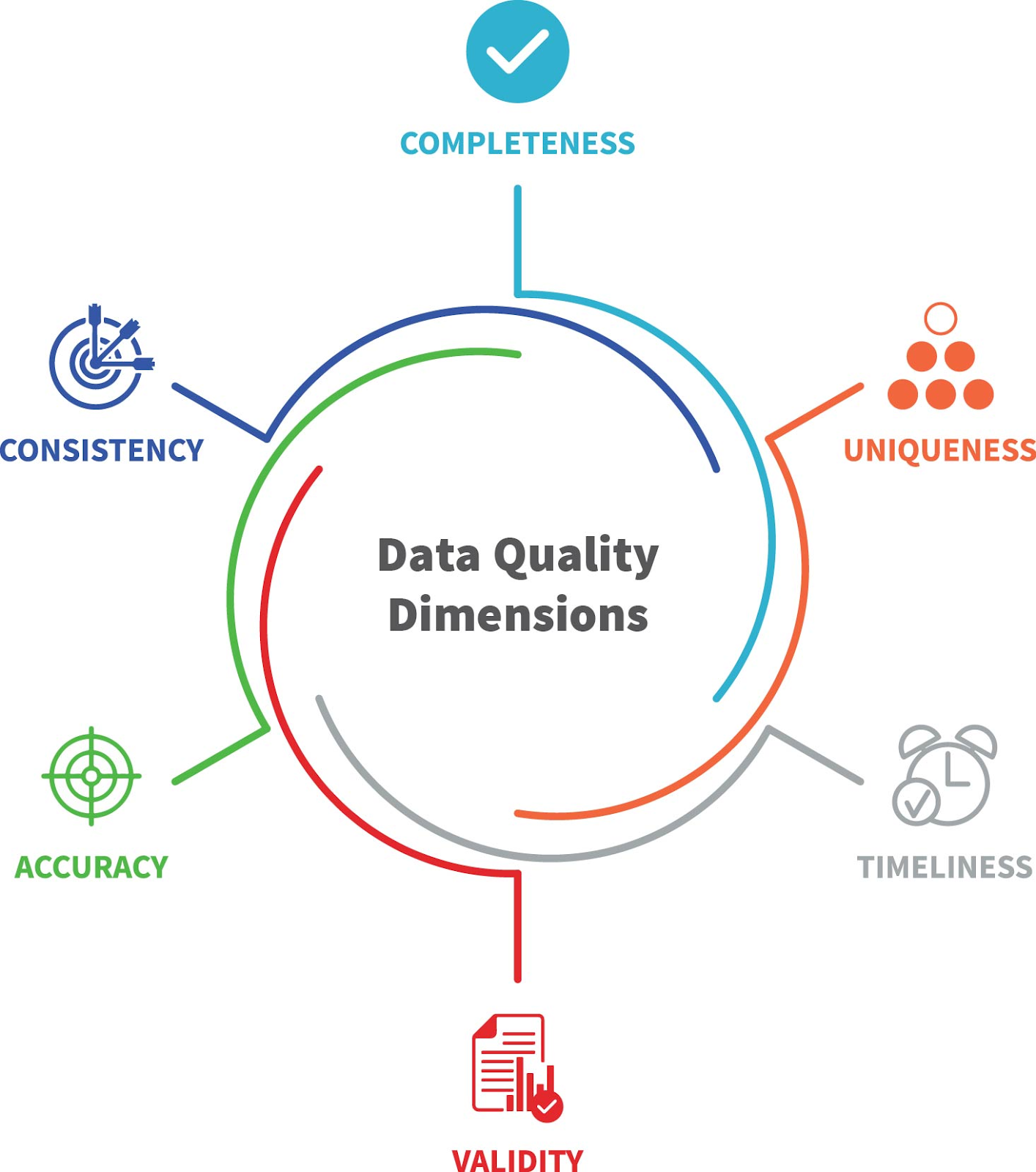
Why Is Data Quality So Important?
Data quality is so important because it directly impacts the success of any business. Inaccurate data can lead to poor decision-making, missed opportunities, and even costly mistakes.
A Gartner research estimated that poor data quality costs US businesses an average of $15 million annually.
Also, an IBM research estimated that businesses in the US alone lose an average of $3.1 trillion annually as a result of poor data quality.
This is why data quality is so important for the success of any business, and why data scientists around the world are dedicating their time and resources to improving it.
How To Improve Your Data Quality
A variety of factors can affect the quality of your data. However, there are some steps you can take to improve the quality of it. Here are 10 tips to help you improve your data quality:
1. Assess And Understand Your Data
The first step to improving your data quality is to understand what you have. This means taking inventory of your data, understanding where it comes from, and assessing its quality.
You can do this by conducting a data audit. This is an assessment of your data that will help you understand its quality and where it needs to be improved.

2. Evaluate And Define What Your Data Standard Should Be
Data standard refers to a set of criteria that data must meet to be considered high-quality. Before you can improve your data quality, you need to set a standard for what high-quality data looks like.
This will help you identify areas where your data needs to improve and set a goal for what you want to achieve.

3. Start By Correcting Data Errors
As we mentioned earlier, data entry errors are one of the most common causes of poor data quality. The first step to improving your data quality is to identify and correct these errors. You can do this by auditing your data regularly and using data cleansing tools to automate the process.
Correcting data errors will help improve the accuracy of your data and, as a result, the quality of your data.
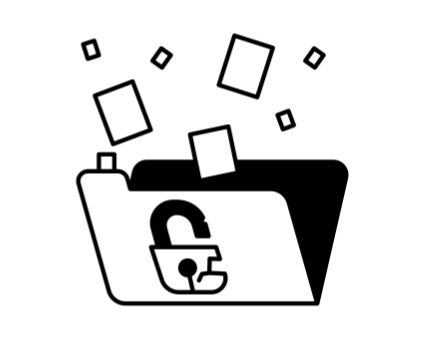
4. Foster A Data Quality Mindset Across Your Organization
Another step to improving your data quality is to foster a data quality mindset across your organization. This means creating a culture of quality where everyone is responsible for the quality of data.
This can be done by creating data quality standards and procedures, and training employees on how to follow them.

5. Make Sure All Users Have Access To The Data
One of the best ways to improve data quality is to make sure all users have access to the data they need. This means giving them the ability to view, edit, and update data. It also means giving them the ability to add new data. This will help ensure that data is accurate and up-to-date.
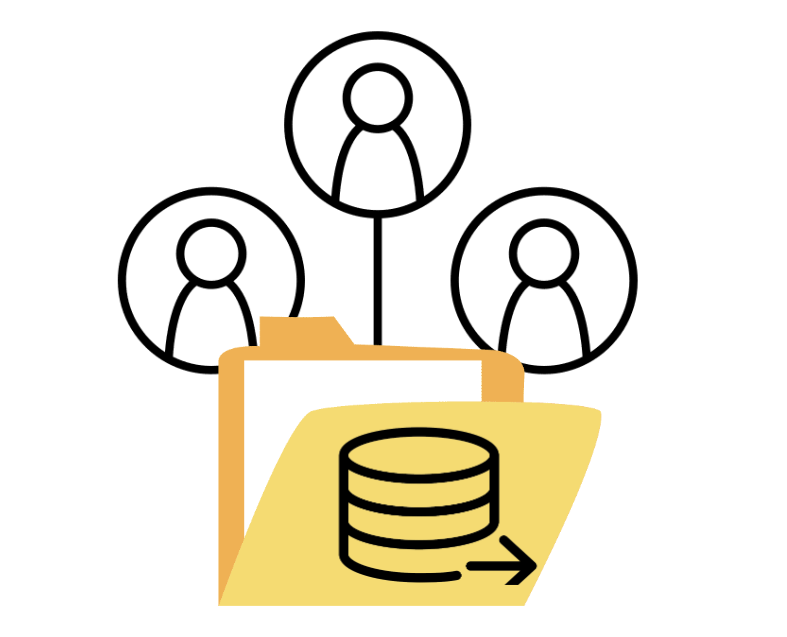
6. Identify And Break Down Data Silos
One of the most common causes of poor data quality is data silos. Data silos occur when different departments or users have access to different sets of data. This can lead to duplication of data, inconsistency, and errors.
To avoid this, you should make sure all users have access to the data they need. You can do this by creating a central repository for all your data or by using a data management platform.
By identifying and breaking down silos, you can improve data quality and ensure all users are working with the same data.
7. Establish A Data Quality Improvement Plan
Another way to improve your data quality is to establish a data quality improvement plan. This is a plan that outlines the steps you will take to improve your data quality.
It should include a review of your current data quality, a set of data quality standards, and a plan for how you will achieve these standards.
By establishing a data quality improvement plan, you can make sure your data quality improvement efforts are focused and effective.

8. Develop A Process To Regularly Assess And Maintain Data Quality
Once you have established a data quality improvement plan, you need to develop a process to regularly assess and maintain your data quality. This means conducting data audits regularly and using data cleansing tools to automate the process.
It also means monitoring and reporting data quality issues so you can identify and address them quickly.
By regularly assessing and maintaining your data quality, you can ensure that your data is accurate and up-to-date.
9. Implement Tools In Your Process
There are several tools you can use to improve your data quality. These tools include data cleansing tools, data quality assessment tools, and data quality improvement tools.
- Data cleansing tools help you identify and correct data errors. Examples of these tools are Talend Data Quality and Informatica Data Quality.
- Data quality assessment tools help you assess your data quality. These tools include Data Quality Review Toolkit and Data Quality Scorecard.
- Data quality improvement tools help you improve your data quality. The most popular tool is Data Quality Management Framework.
These tools can help you automate the process of data quality improvement and make it easier to identify and correct errors. By using these tools, you can improve your data quality and make sure your data is accurate and up-to-date.
10. Appoint A Data Expert
One of the best ways to improve your data quality is to appoint a data expert. This person will be responsible for overseeing all aspects of your data quality. They will be responsible for developing data quality standards, conducting data audits, and managing data cleansing efforts.
By appointing a data expert, you can ensure that your data quality improvement efforts are focused and effective.
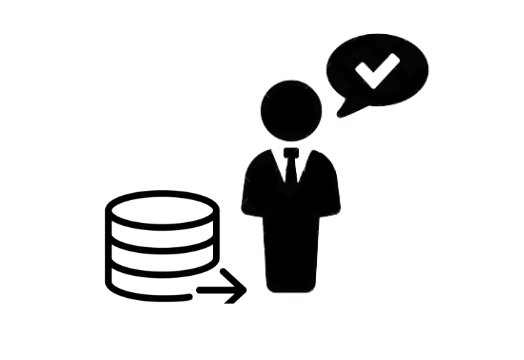
Grow Your Business With Good Data Quality
Good data quality is essential for businesses of all sizes. It helps you make better decisions, enhance your customer experience, improve your operations, increase your revenue, and grow your business!
If you need help improving your data quality, contact Capella Solutions today. We have the experience and expertise to help you establish a data quality improvement plan tailored to your organization's specific needs.
Contact us today to learn more about how we can help you improve your data quality!

1. What is data quality and why should organizations care about it?
Data quality refers to how accurate, complete, consistent, and timely data is. High quality data is essential for organizations to operate efficiently and make smart decisions. Poor data quality leads to errors, unreliability, lack of insights, poor customer experiences, and compliance issues. Every organization should make continuous improvements to data quality a priority in order to reduce costs, risks and enable fact-based strategic planning.
2. What are some common indicators of poor data quality?
Common signs of poor data quality include high error rates in reports, missing or outdated information, duplicate or contradictory records, violations of integrity constraints, failed validation checks, and anomalies that don't align with expectations. Customer complaints about incorrect data may also indicate quality issues. Lack of trust in data and excessive manual verification are also signals that quality needs improvement.
3. How can organizations assess and measure the quality of their data?
Data quality should be measured across dimensions like accuracy, completeness, consistency, conformity, and timeliness. Statistical profiling examines data for anomalies and patterns indicative of quality problems. Data auditing compares datasets to identify errors and inconsistencies. Quality metrics should be defined and tracked over time to quantify improvements. Assessing data quality is crucial for identifying which areas need attention.
4. What are some best practices for improving data quality?
Strategies for improving data quality include clarifying business rules for entering valid data, implementing strong validation checks on data entry, consolidating data in master databases with a unified schema, establishing persistent unique identifiers for records, profiling new data before integration, providing staff training on data disciplines, appointing data stewards, documenting all systems of record, and continually monitoring quality metrics.
5. How can organizations prevent the accumulation of bad data on an ongoing basis?
Preventing future accumulation of bad data requires fixing root causes, putting guards in place to catch errors early, and establishing accountability. This includes training personnel, automating validation checks, masking or quarantining records that don’t meet quality standards, tracking edit histories on records, monitoring incoming data in real-time, and profiling batches of new data for anomalies.
6. What role can data governance play in improving data quality?
Data governance establishes policies, guidelines, processes, and decision rights for managing data. This helps set organization-wide standards for data quality. Governance policies can require documentation, change control approval, master data management, persistent identifiers, access controls, and compliance audits. Data stewards oversee critical subject areas. Governance provides an institutional foundation to build and sustain high data quality.
7. What types of tools and technologies are available for improving data quality?
Tools for improving data quality include profiling tools to analyze datasets and detect anomalies, parsing and standardization tools to fix formatting inconsistencies, data matching to deduplicate records, monitoring and notification when issues arise, and comprehensive data quality suites. Specialized tools handle domains like addresses and contact info. Machine learning techniques can automate quality checks. Cloud-based tools reduce infrastructure requirements.
8. How can master data management help organizations improve data quality?
Master data management (MDM) consolidates data from different systems into unified "golden records" that provide authoritative, trusted information. MDM applies consistent data rules and quality checks which improves completeness, accuracy, and consistency. Having unified definitions and codified business rules enhances quality. MDM enables tracking data lineage and profiling datasets before integration into master records.
9. What role should data professionals play in improving data quality?
Data professionals like data analysts, engineers, and stewards have key responsibilities around improving data quality. Data analysts profile and audit data to assess quality issues. Data engineers develop ETL pipelines that apply validation rules and corrections. Data stewards define governance policies and standards. Data custodians act as subject matter experts on critical data. All data professionals have a role to play in continuous data quality improvement.
10. Why should data quality be viewed as an ongoing discipline rather than a one-time project?
Data quality can't be tackled through a single project with an end date. New data is always emerging. Business priorities shift. Technology changes. Data decay inevitably sets in over time. To maintain high quality, organizations need documented standards, automated monitoring, executive commitment, andmost importantlya culture that values qualityas an operational discipline that requires ongoing vigilance, measuring, and improvement. Data quality is a journey, not a destination.
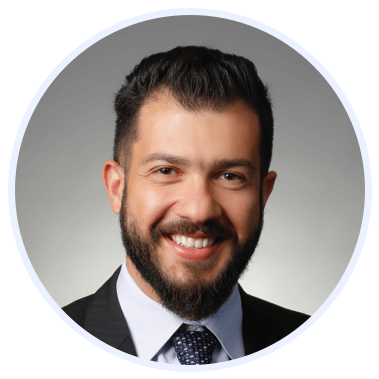
Rasheed Rabata
Is a solution and ROI-driven CTO, consultant, and system integrator with experience in deploying data integrations, Data Hubs, Master Data Management, Data Quality, and Data Warehousing solutions. He has a passion for solving complex data problems. His career experience showcases his drive to deliver software and timely solutions for business needs.