
I remember watching a veteran machinist at one of our client facilities show a new hire the subtle art of calibrating a complex CNC machine. The expertise wasn't in the manual – it was in his head, gained from decades of experience. As he demonstrated the precise sequence of adjustments, I couldn't help but think about how many similar knowledge-sharing moments happen across factory floors every day. And how many of these valuable insights are lost when experienced workers retire or move on.
After spending years helping manufacturing companies optimize their operations, I've seen firsthand how tribal knowledge becomes a critical bottleneck. The real cost isn't just in the immediate inefficiencies – it's in the cumulative loss of expertise that could have powered innovation and growth for years to come.

The Hidden Cost of Lost Knowledge
Manufacturing operations thrive on expertise that's often unwritten. The maintenance technician who knows exactly how to troubleshoot a temperamental machine. The quality inspector who can spot defects through subtle signs others might miss. The production supervisor who's memorized the optimal settings for different material batches. This knowledge, accumulated over years of hands-on experience, represents an invaluable asset that most companies inadvertently let slip away.
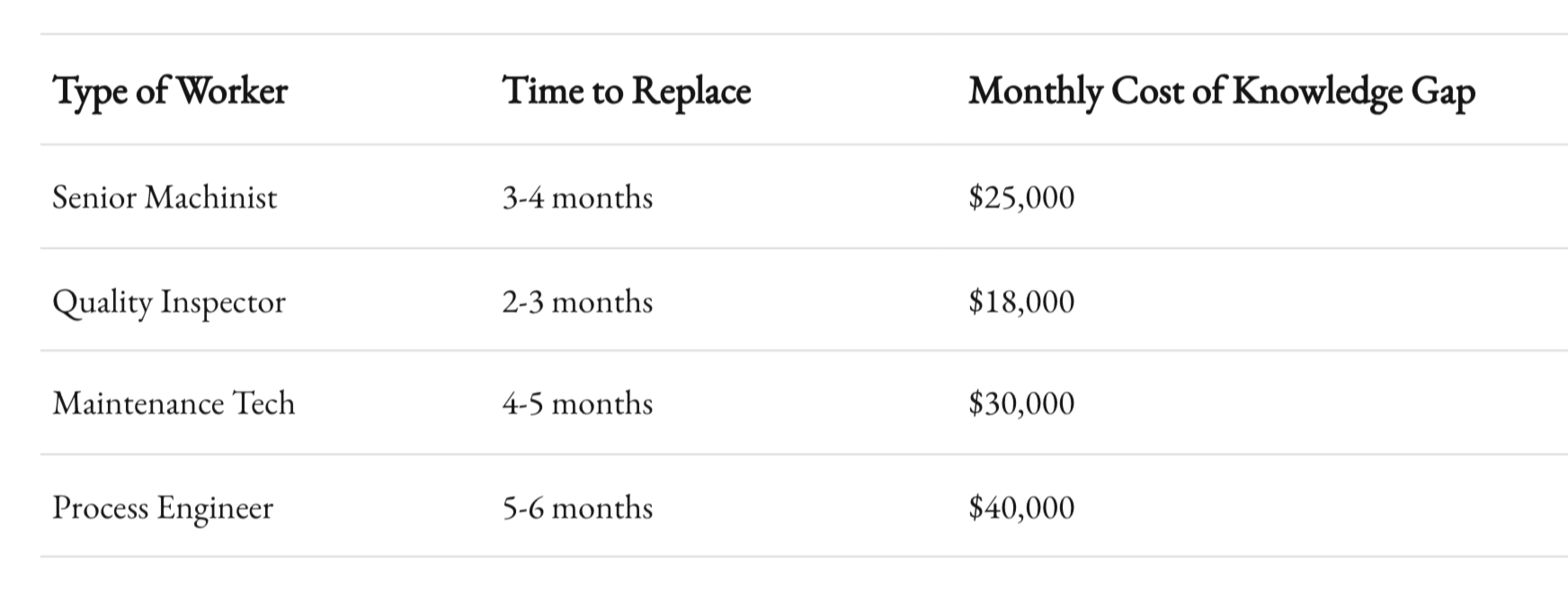
The financial impact is staggering. In my work with mid-sized manufacturers, I've seen production delays stretch into days when key knowledge holders are unavailable. New hires take months longer to reach full productivity when they can't access the insights of their experienced colleagues. Quality issues crop up as best practices get lost in translation between shifts.
But it doesn't have to be this way. Modern AI technologies have evolved to capture, preserve, and share operational knowledge in ways that feel natural and intuitive.
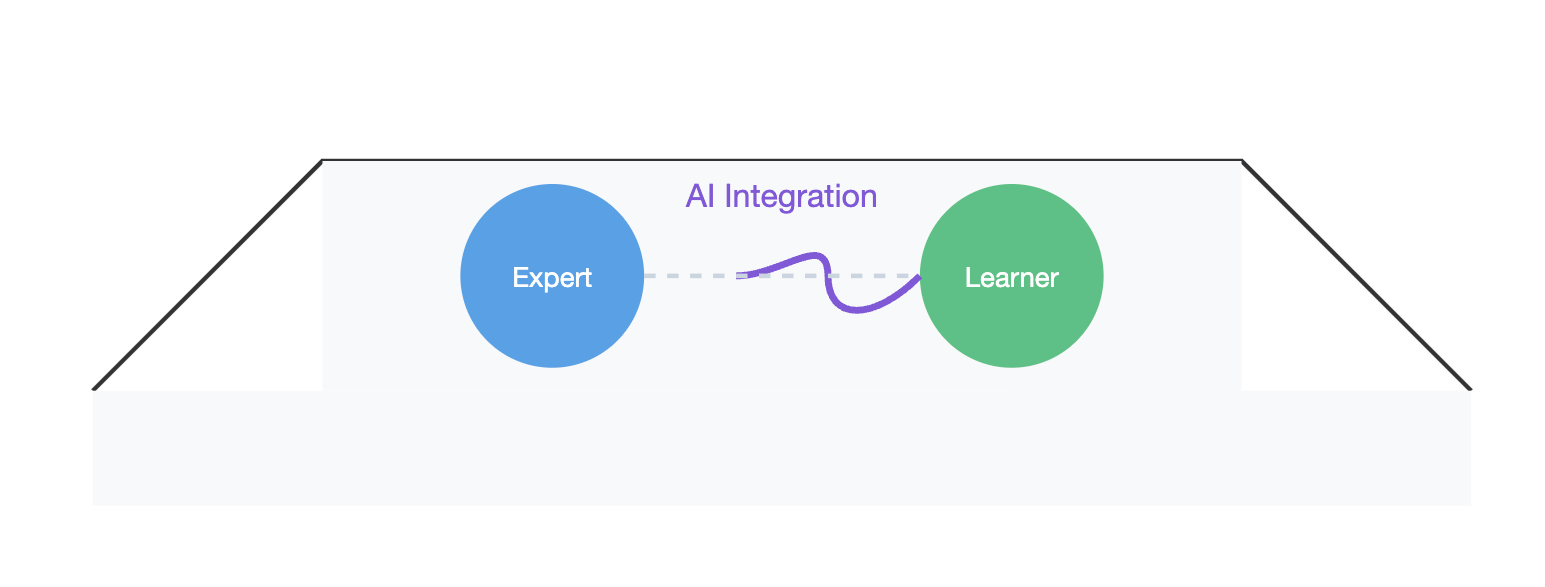
Beyond Traditional Documentation
Traditional approaches to knowledge management – thick binders of standard operating procedures, shared network drives full of PDFs, or basic wikis – fall short in manufacturing environments. They're static, hard to update, and rarely capture the nuanced know-how that makes operations run smoothly.

I've seen companies invest heavily in documentation initiatives, only to watch their carefully crafted manuals gather dust. The reality is that busy operators and technicians don't have time to dig through pages of text when they need an answer. They need relevant information, instantly accessible, in a format that matches how they actually work.
This is where AI-driven knowledge sharing systems shine. They can understand natural language questions, pull relevant insights from various sources, and present solutions in clear, actionable terms. When that new machinist needs to recall the calibration steps, they can simply ask the system as if they were asking their mentor.
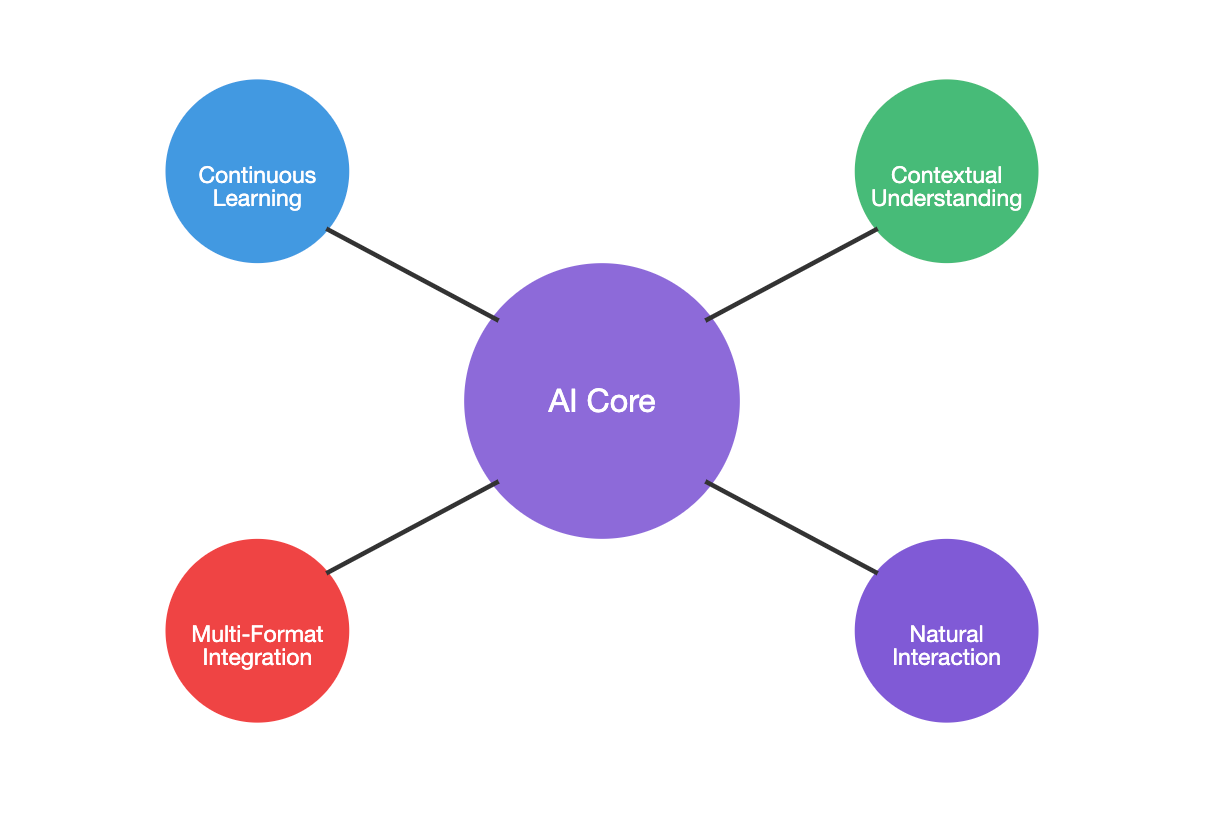
The AI Knowledge Engine at Work
The most effective knowledge-sharing solutions I've implemented combine several key capabilities:
Continuous Learning
The system doesn't just store static information – it learns from every interaction. When experienced workers resolve issues or discover better methods, the AI captures these insights and adds them to its knowledge base. This creates a living repository that grows smarter over time.
Contextual Understanding
Modern language models can understand the context behind questions. If an operator asks about adjusting feed rates, the system knows to consider the specific machine type, material being processed, and current production parameters. This contextual awareness ensures relevant, practical answers.
Multi-Format Integration
Manufacturing knowledge comes in many forms – text documents, photos, videos, sensor data, and verbal explanations. Advanced AI can synthesize information across these formats, making all forms of expertise searchable and accessible.
Natural Interaction
The best systems feel like talking to a knowledgeable colleague. Workers can ask questions in plain language, get clarification if needed, and receive answers that match their level of expertise.
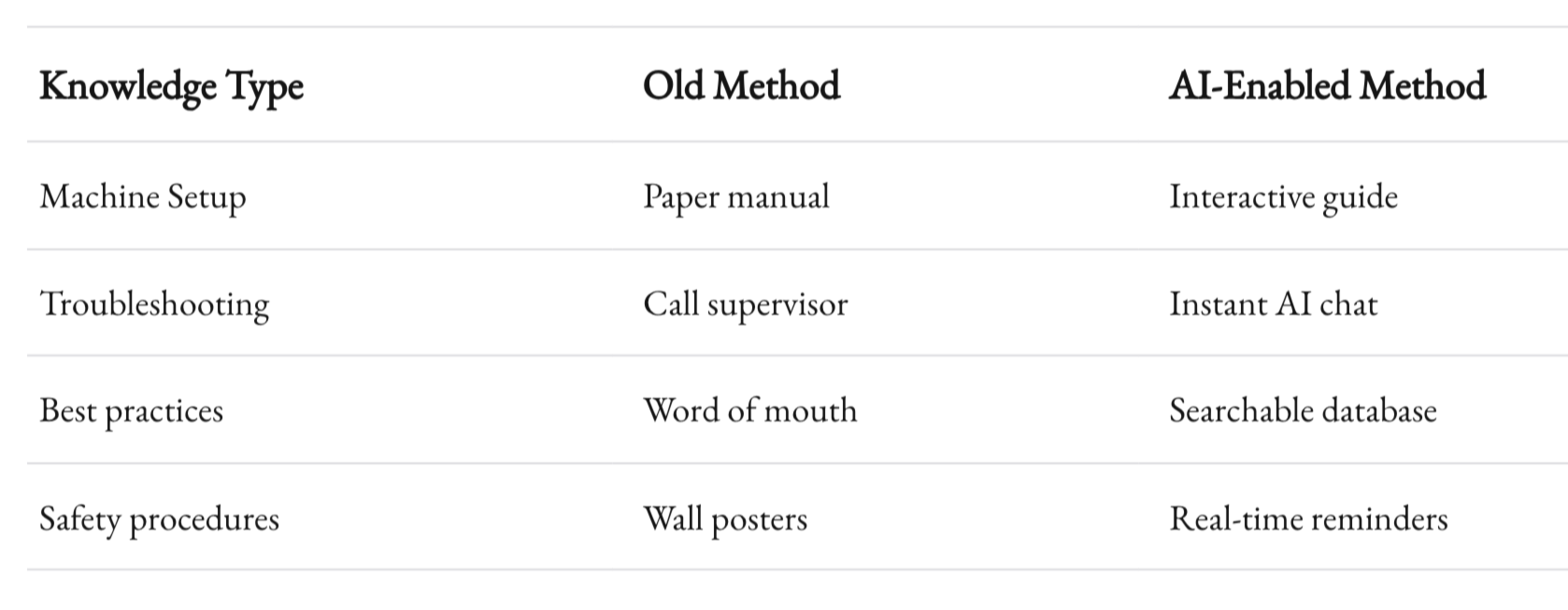
Real Impact on Operations
Let me share a recent example from one of our implementations. A medium-sized automotive parts manufacturer was struggling with knowledge transfer between shifts. Their most experienced operators worked the day shift, leaving night shift workers without ready access to their expertise.

We implemented an AI-driven knowledge sharing system that:
- Captured detailed process knowledge from senior operators
- Made this expertise available 24/7 through a simple chat interface
- Automatically documented successful problem resolutions
- Translated complex technical information into clear, actionable steps
The results were transformative. Within three months:
- Training time for new operators decreased by 40%
- Quality issues during night shifts dropped by 35%
- Time spent searching for solutions decreased by 60%
But the most telling impact came from an unexpected source. The system began identifying patterns in how different operators handled similar challenges, leading to standardized best practices that improved efficiency across all shifts.
Making It Work in Your Operation
Successfully implementing AI-driven knowledge sharing requires more than just deploying technology. Based on my experience guiding dozens of manufacturers through this transition, here are the critical success factors:
Start with High-Impact Areas
Begin where knowledge gaps cause the most pain. This might be complex maintenance procedures, quality troubleshooting, or setup processes for difficult products. Early wins in these areas build momentum for broader adoption.
Engage Your Experts
Your experienced workers are key to success. Involve them early, show them how the system preserves and amplifies their expertise, and make them champions of the initiative. Their buy-in will encourage others to embrace the new approach.
Build Trust Through Transparency
Workers need to understand how the AI makes recommendations. Be clear about where the knowledge comes from and maintain human oversight for critical decisions. This builds confidence in the system's suggestions.
Integrate with Existing Workflows
The easier it is to use the system, the more it will be used. Integrate knowledge sharing into existing processes and tools rather than adding extra steps to workers' routines.
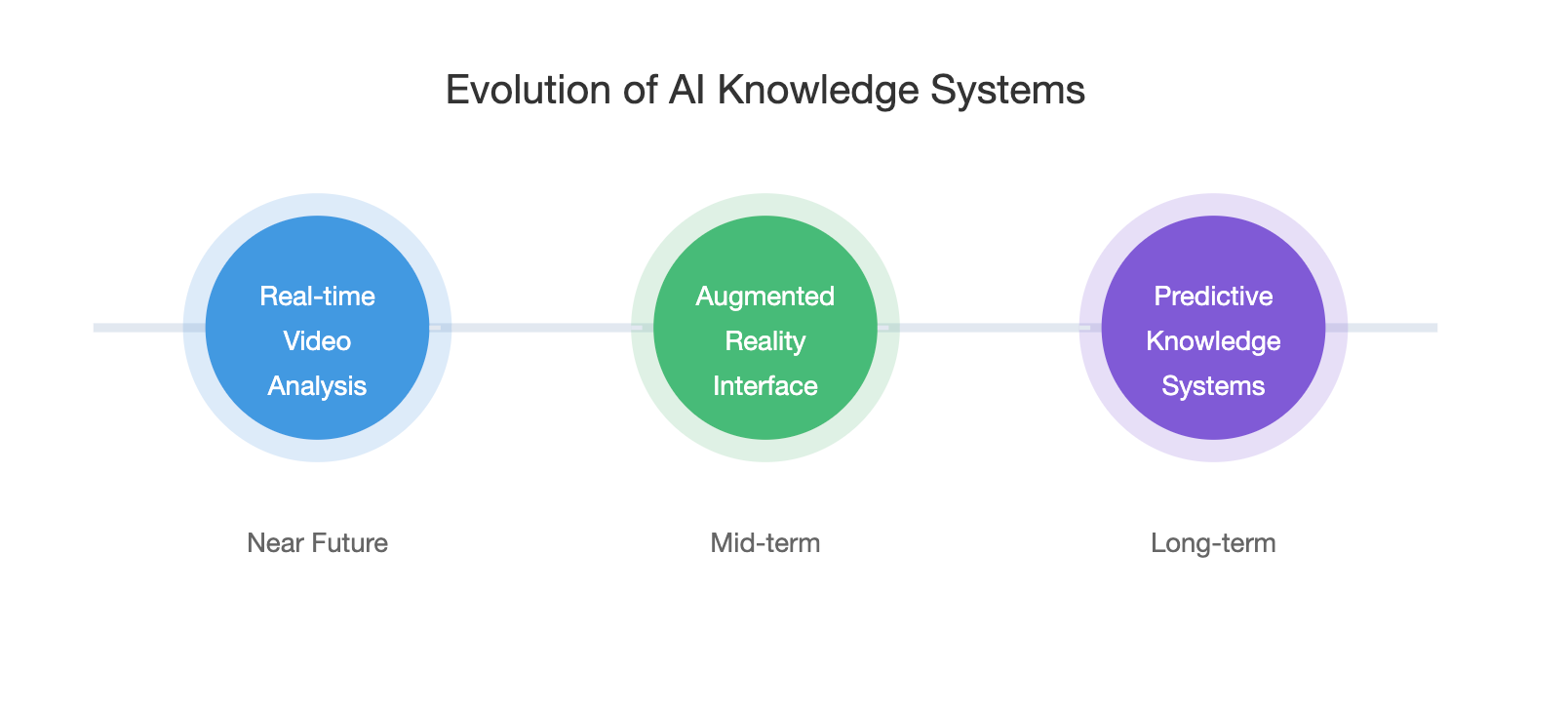
Looking Ahead
The future of manufacturing knowledge sharing is both exciting and practical. As AI technology continues to evolve, we're seeing new capabilities emerge:
- Real-time video analysis that can watch experienced workers and automatically document their techniques
- Augmented reality interfaces that overlay relevant knowledge onto physical equipment
- Predictive systems that anticipate knowledge needs based on current operations
But the core principle remains unchanged: valuable operational knowledge should be preserved, shared, and built upon. AI simply makes this more natural and effective than ever before.

Taking the First Step
If you're considering implementing AI-driven knowledge sharing in your operation, start by assessing your current knowledge management challenges:
- Where do information bottlenecks occur most frequently?
- Which processes rely heavily on tribal knowledge?
- What expertise are you at risk of losing to retirement or turnover?
The answers will guide you toward the most valuable applications of this technology for your specific situation.

Remember, the goal isn't to replace human expertise – it's to amplify and preserve it, making your entire operation more resilient and capable. When done right, AI-driven knowledge sharing doesn't just solve immediate operational challenges; it creates a foundation for continuous improvement and innovation.
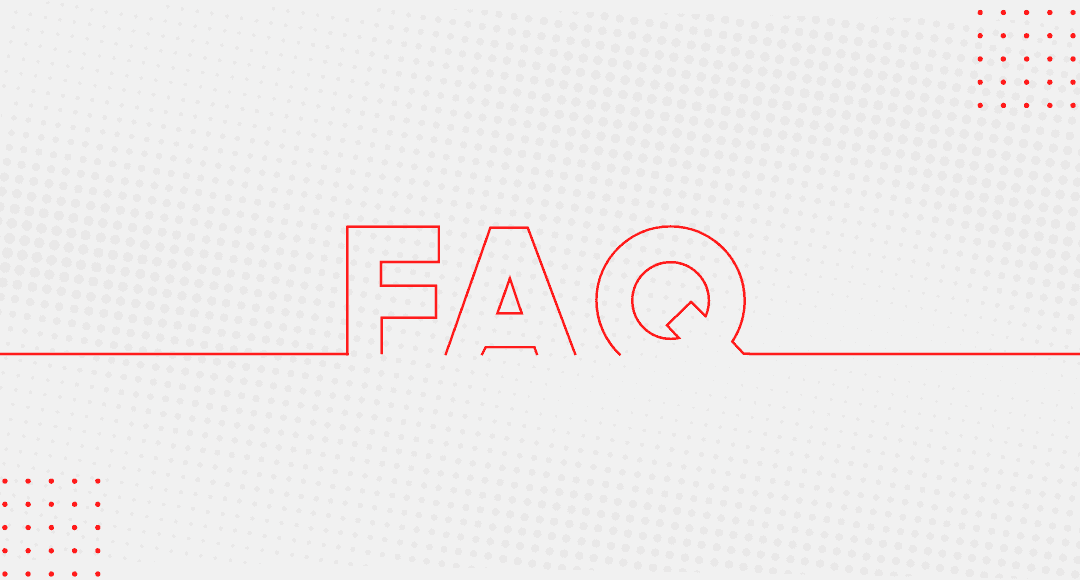
Q: How much does it typically cost to implement an AI knowledge sharing system in a mid-sized manufacturing facility?
The cost varies based on complexity and scale, but for a mid-sized facility with 100-200 workers, initial implementation typically ranges from $50,000 to $100,000. This includes software licensing, initial setup, and training. However, most facilities recover this investment within 6-8 months through reduced training costs, fewer errors, and improved productivity. One of my clients recently saved $180,000 in the first year just by reducing overtime needed for training and troubleshooting.
Q: We already have a document management system. Why do we need AI-powered knowledge sharing?
Traditional document management systems are great for storing information but poor at retrieving contextual knowledge. Think of it this way: if a machine operator needs to know why their usual settings aren't working with a new material, they don't want to read through 50 pages of documentation. They need immediate, relevant answers. AI systems can understand the context of questions, pull information from multiple sources, and provide specific, actionable answers in seconds.
Q: How do we ensure sensitive proprietary information stays secure?
Security is built into modern AI knowledge systems through multiple layers. You maintain full control over what information is ingested into the system, who can access it, and how it can be shared. We typically implement role-based access controls, encryption, and audit trails. The system can be hosted on-premises if required, ensuring your intellectual property never leaves your network.
Q: What happens if our internet connection goes down?
Most systems are designed to work offline with core functionality. Critical knowledge is cached locally, allowing workers to access essential information even without internet connectivity. When connection resumes, the system automatically syncs any new information or interactions that occurred during the offline period. One of our implementations in a rural facility maintains 95% functionality during internet outages.
Q: How do we get our experienced workers to share their knowledge with the system?
The key is making knowledge sharing feel natural and rewarding. Instead of asking workers to document everything they know, we use methods like recording short video explanations, voice notes, or having the AI system learn from their problem-solving conversations. We also implement recognition programs where workers get credit (and often rewards) when their shared knowledge helps others solve problems.
Q: How long does it take to see real results from implementation?
While some benefits are immediate, like faster access to information, the full impact typically becomes clear within 3-4 months. One automotive parts manufacturer saw a 40% reduction in training time within the first month, but the more substantial benefits – like a 35% reduction in quality issues and 25% improvement in first-time-right rates – emerged after three months of consistent use.
Q: What happens to the system's effectiveness when we introduce new processes or equipment?
The AI system is designed to learn and adapt continuously. When you introduce new processes or equipment, the system can be quickly updated with new documentation, and it learns from how workers interact with these changes. The more it's used with new processes, the more effective it becomes. We typically see the system adapt to major changes within 2-3 weeks of implementation.
Q: How does the system handle multiple languages in a diverse workforce?
Modern AI knowledge systems support multiple languages seamlessly. Workers can ask questions in their preferred language and receive answers in that same language, even if the original information was in a different language. The system maintains technical accuracy across translations, which is crucial for manufacturing environments. One of our implementations successfully operates in five different languages at a single facility.
Q: What metrics should we track to measure the system's effectiveness?
Focus on metrics that directly impact your operations: time spent finding information (should decrease by 70-80%), training time for new hires (typically reduces by 40-50%), error rates (expect 30-40% reduction), and time spent by experts handling routine questions (usually drops by 60%). Also track indirect metrics like employee satisfaction and knowledge engagement rates.
Q: What's the most common mistake companies make when implementing these systems?
The biggest mistake is treating it as purely a technology implementation rather than a change management project. Success depends on proper change management, clear communication, and sustained engagement from all levels. Companies that rush to implement without properly engaging their workforce often struggle with adoption, even if the technical implementation is perfect. Start with a clear communication plan and involve workers in the implementation process from day one.
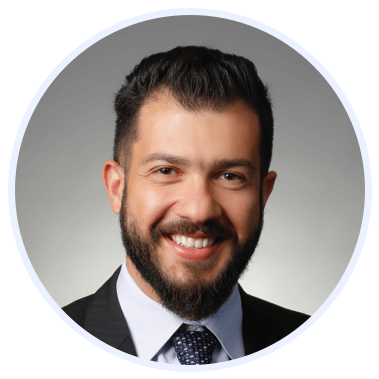
Rasheed Rabata
Is a solution and ROI-driven CTO, consultant, and system integrator with experience in deploying data integrations, Data Hubs, Master Data Management, Data Quality, and Data Warehousing solutions. He has a passion for solving complex data problems. His career experience showcases his drive to deliver software and timely solutions for business needs.