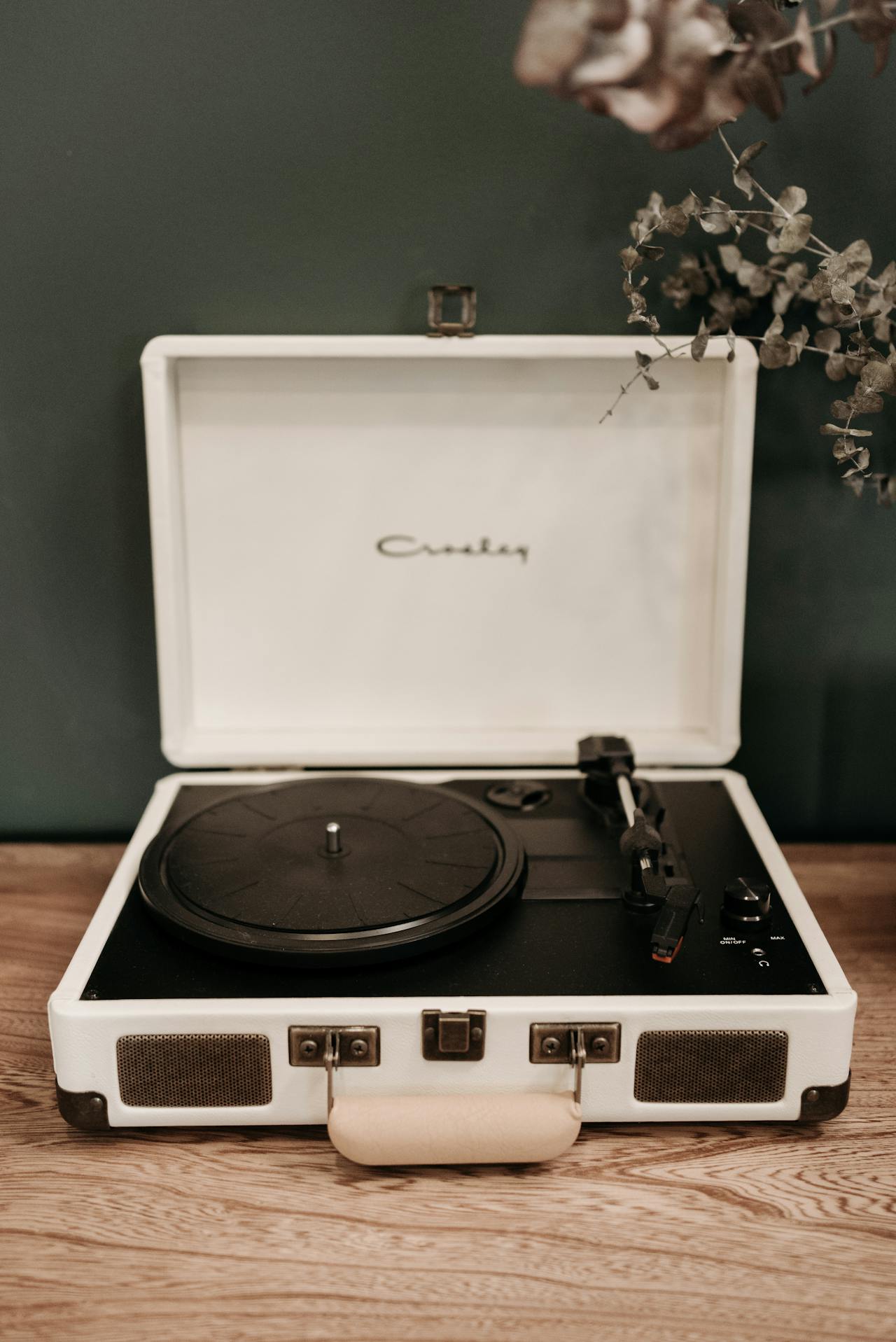
As a senior leader in any large organization, you’ve likely had one of those quiet moments of reflection. The kind where you think, “We’ve made it this far with what we have. Why fix what isn’t broken?” But here’s the thing: Your legacy systems aren’t just “not broken,” they’re probably holding you back in ways you can’t afford to ignore.
It’s hard to admit, but the systems you’ve relied on for years—sometimes decades—are no longer the gold standard. They were designed in an era that is now a distant memory. And while they may seem stable, they’re quietly draining your bottom line. That’s because the true cost of maintaining legacy systems goes beyond their upfront price tag.
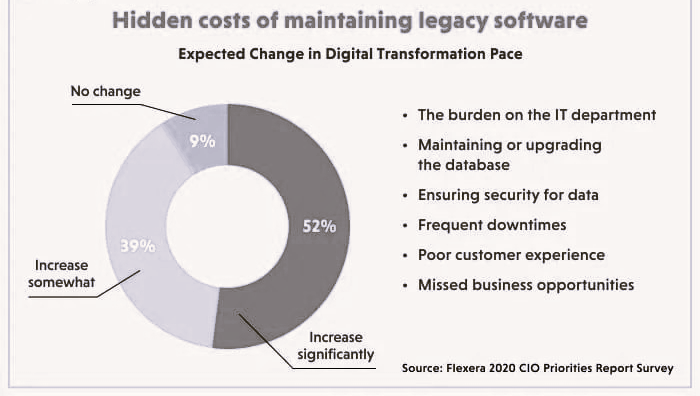
The Growing Financial Drain of Legacy Systems

Let’s start with the facts. According to a 2023 report from Gartner, companies spend an average of 70% to 80% of their IT budgets on maintaining legacy systems. That’s a staggering amount when you consider that the average company’s IT spend only grows by 3% year-over-year. So, if a significant chunk of that budget is locked into outdated infrastructure, what’s left for innovation? What’s left for AI-driven transformation?
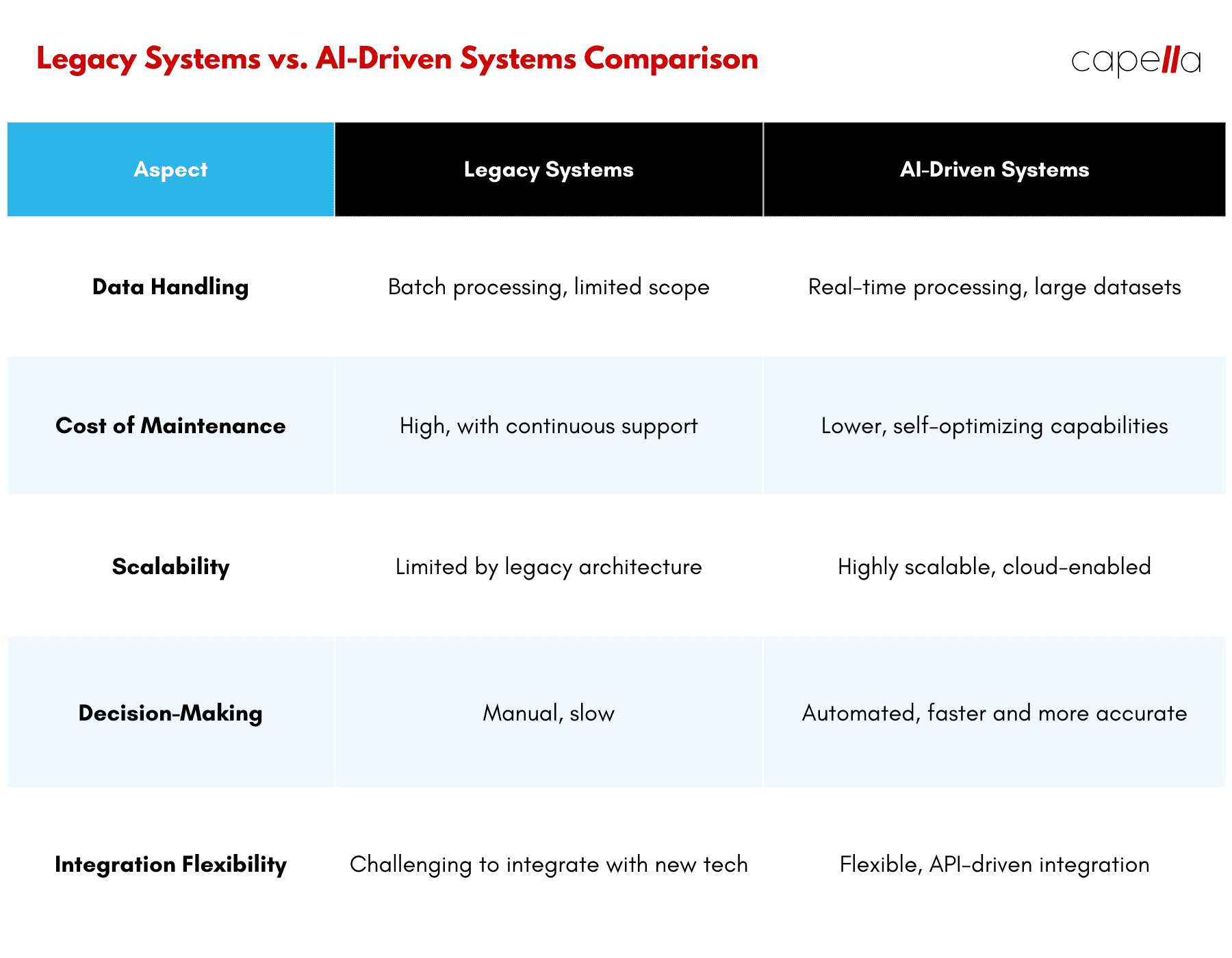
Legacy systems, whether they’re ERP solutions, on-prem databases, or custom-built tools from another era, often fail to scale. They can’t handle the volume and complexity of modern data, and worse, they lack the flexibility to integrate with newer, more agile technologies. These bottlenecks lead to slow decision-making, reduced productivity, and ultimately, lost profits.
In fact, a McKinsey & Company report revealed that companies still relying heavily on legacy systems face a 20% lower productivity rate compared to organizations that have adopted modern technologies. That's not just a statistic—it’s the type of inefficiency that directly affects your profitability.
But let’s break this down. What exactly does this mean for your organization? How does AI integration turn the tide? And more importantly, how do you get started?
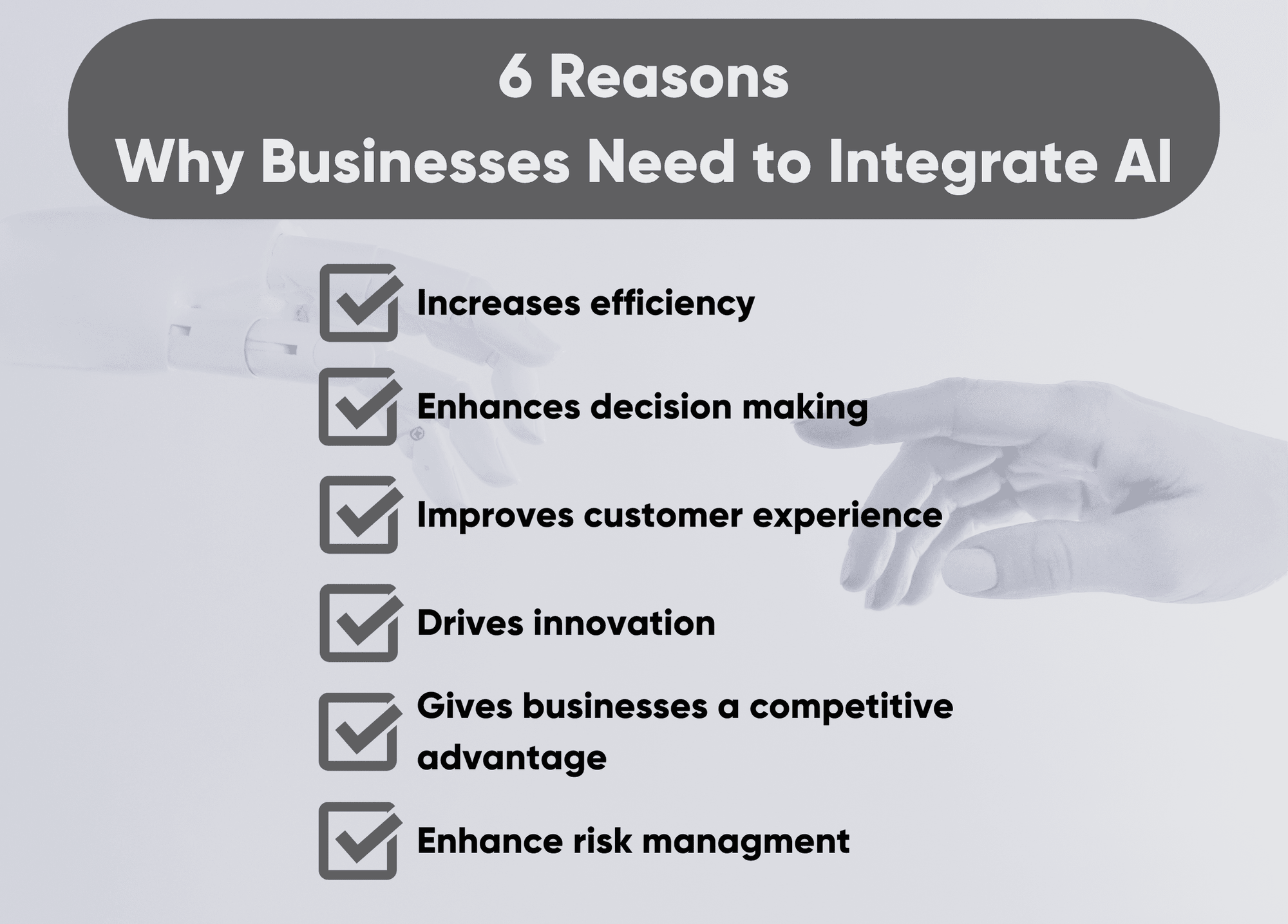
The Case for AI Integration
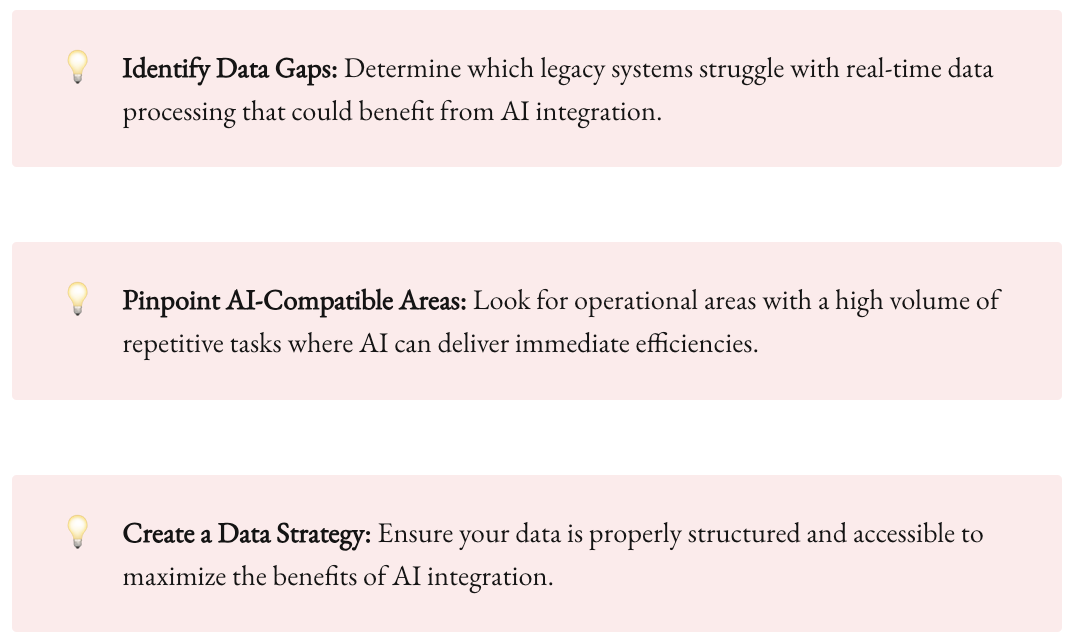
At its core, AI is about intelligence—processing vast amounts of data at speeds and with accuracy far beyond what any human or traditional system could accomplish. AI can help automate complex tasks, analyze data in real time, and provide insights that are tailored to the needs of your organization.

But here’s where it gets interesting: Legacy systems can’t handle AI. To put it bluntly, they weren’t built with AI in mind. Legacy systems struggle to integrate with modern AI technologies, creating silos where data can’t flow freely and insights can’t be leveraged efficiently.
Let’s take a practical example. Imagine you run a large manufacturing operation. You’ve invested heavily in legacy systems for inventory management, procurement, and production. These systems have served you well, but now they’re becoming increasingly cumbersome. Real-time demand forecasting is almost impossible, and when supply chain disruptions occur, it takes days to manually assess the impact.
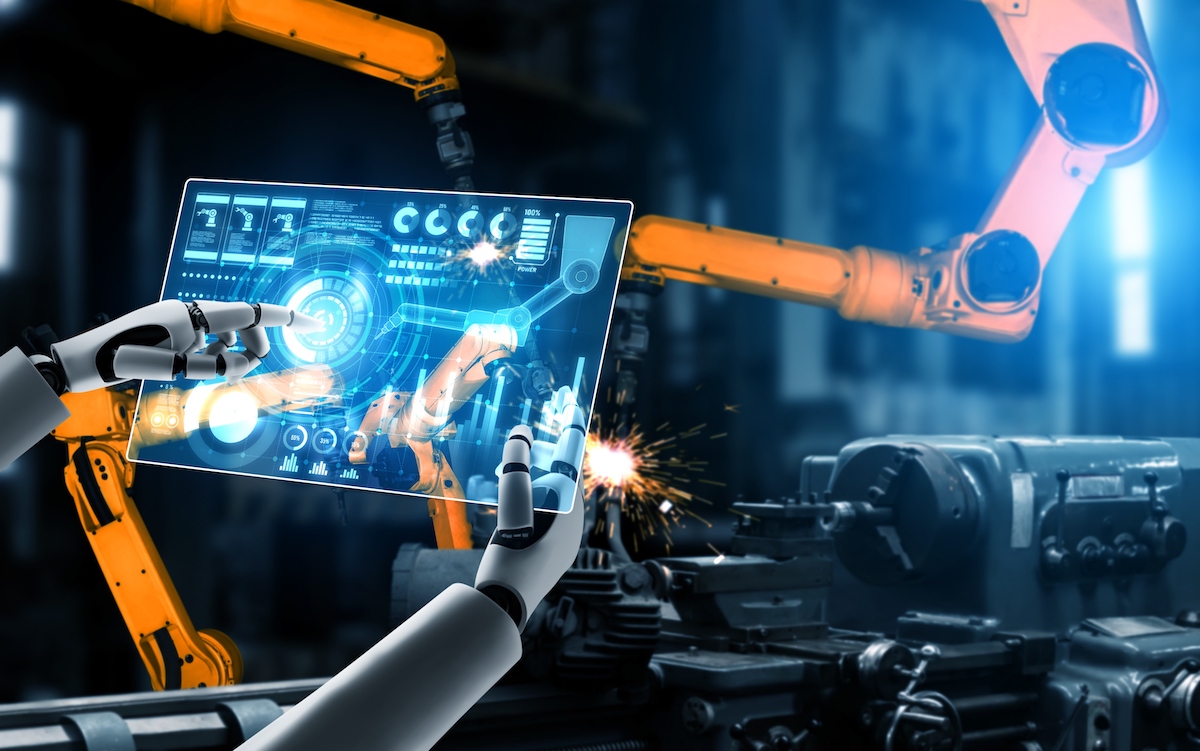
A Real-World Example
Now, imagine integrating an AI-driven predictive analytics platform into your workflow. The AI could instantly process and analyze historical demand data, global supply chain trends, and even social media sentiment to provide real-time forecasts that help you optimize inventory levels and reduce waste.
By feeding the AI with real-time data from your systems, the technology will identify patterns and trends that your legacy systems can’t. It can alert you to supply chain risks before they materialize, suggest optimal purchasing decisions, and even forecast sales performance with remarkable accuracy.
This is the power of AI. It transforms data from a burden into a business asset, driving efficiencies, improving decision-making, and ultimately increasing profits.
The True Cost of Sticking with Legacy Systems

If the potential of AI isn’t enough to get you thinking, consider this: The true cost of maintaining legacy systems often goes unnoticed.
It’s not just about money. It’s about opportunity. Every day you hold off on AI integration, you risk being left behind by competitors who are already using AI to improve customer experiences, streamline operations, and make data-driven decisions.
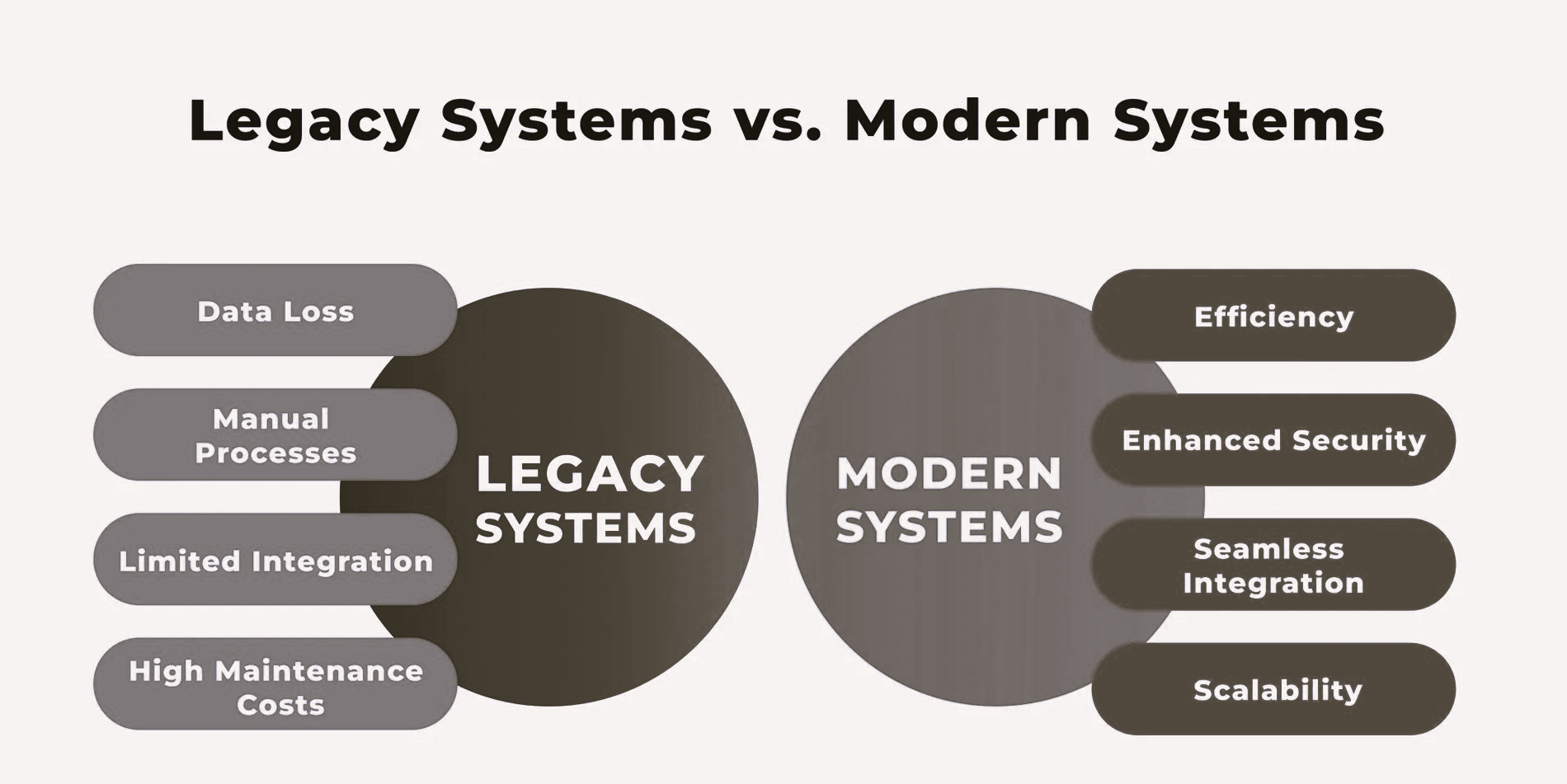
In fact, a Forrester Research survey found that 62% of firms that had integrated AI into their operations saw a 30% increase in customer satisfaction within the first year of implementation. Conversely, those stuck with legacy systems were more likely to face customer churn and slower response times.
Addressing the Risks of AI Integration
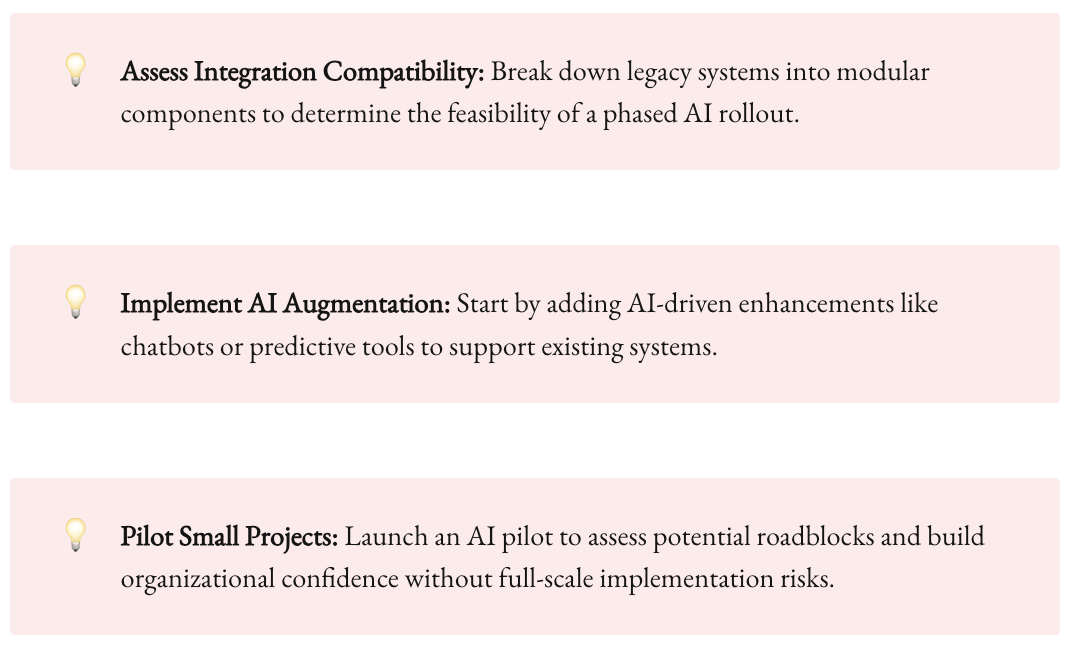
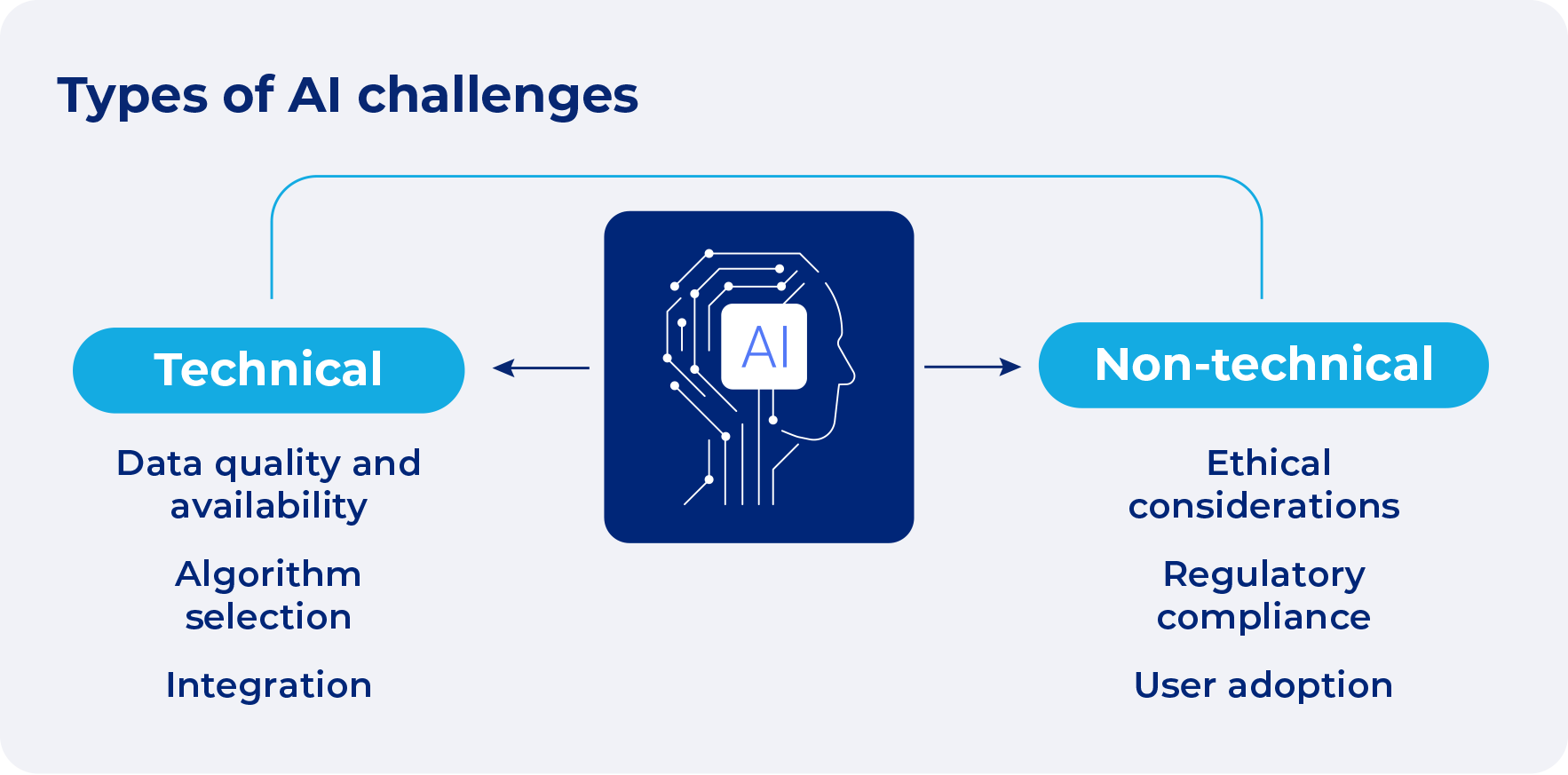
AI sounds great on paper, but the idea of integrating it into an existing legacy infrastructure can be daunting. The risks are real: data migration challenges, compatibility issues, and potential downtime during the transition.
But this is where strategy comes into play. AI integration isn’t about replacing everything at once. It’s about identifying the right opportunities for AI to enhance your current systems and workflows.
For example, consider breaking down your legacy systems into modules. Instead of overhauling everything, identify areas where AI can drive immediate results and start with small, focused experiments. These early wins can help build momentum and generate the buy-in you need for larger AI initiatives.
One such strategy is known as AI augmentation, where AI is used to complement your existing systems rather than replace them entirely. In this approach, AI takes on the heavy lifting of repetitive tasks—such as data entry or basic customer queries—while your legacy systems handle more complex processes that require human oversight.
Here’s a practical example:
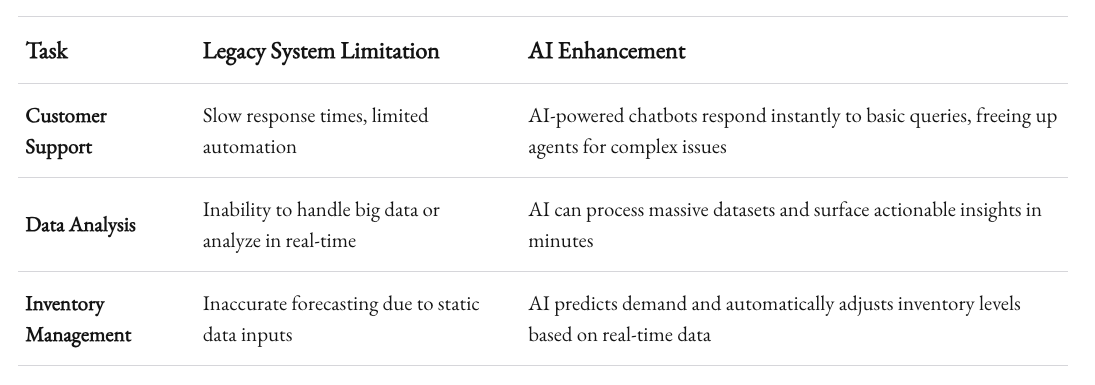

How to Get Started with AI Integration
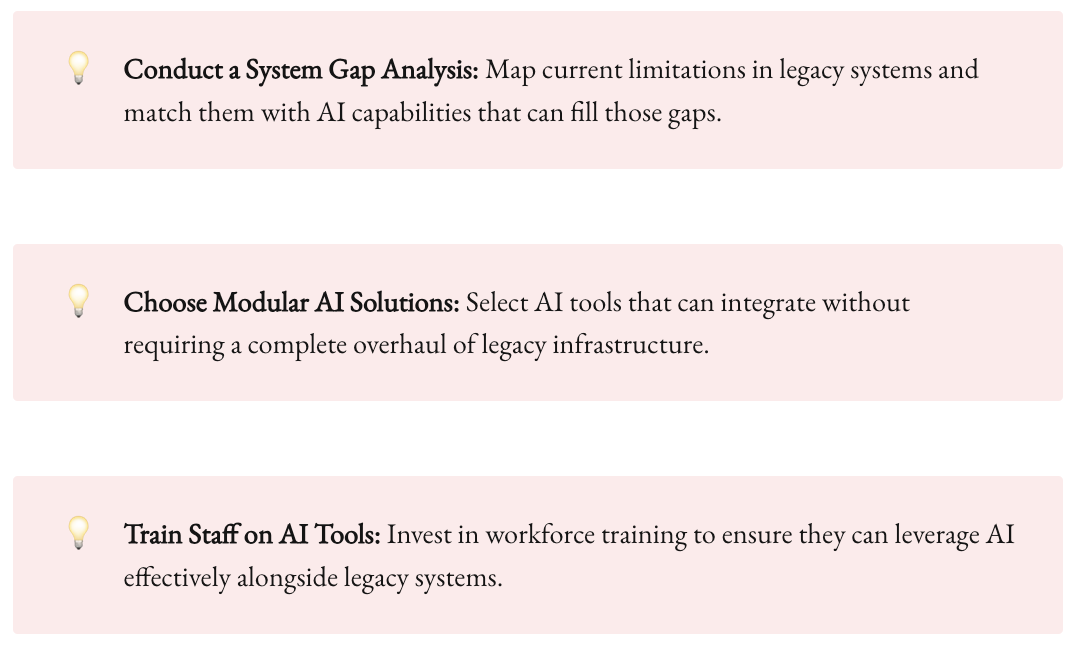
- Assess the Gaps in Your Current Systems
Start by evaluating your existing legacy systems and identifying areas where they fall short. Is there a delay in data processing? Is your customer support system struggling with volume? Pinpoint these bottlenecks and figure out where AI can step in to drive improvements. - Choose the Right AI Tools
You don’t need to reinvent the wheel here. There are plenty of AI platforms designed to integrate seamlessly with existing systems. Whether it’s predictive analytics, natural language processing (NLP) for chatbots, or AI-powered business intelligence (BI) tools, the right tools can augment your legacy infrastructure without requiring a complete overhaul. - Start Small, Scale Fast
Launch a pilot project. Start with a single department or process where AI can deliver quick wins. Once you’ve proven the value, expand the integration across other areas. This phased approach reduces risk and builds confidence in the technology. - Invest in Data Quality
AI is only as good as the data it learns from. If your legacy systems are holding onto fragmented or low-quality data, it’s time to clean house. Investing in data management tools to ensure accuracy and consistency will help your AI systems deliver actionable, reliable insights. - Build a Culture of Innovation
AI integration isn’t just about technology—it’s about people. Foster a culture that embraces change and innovation. Ensure your team is trained in new AI tools and workflows to maximize the technology’s potential.

The Clock is Ticking
Let’s be clear: Legacy systems are holding your organization back. They’re outdated, inefficient, and a drain on your profits. AI offers the solution, but only if you act now. The longer you wait, the greater the risk of being left behind.
Embracing AI is no longer a luxury—it’s a necessity. By strategically integrating AI into your existing infrastructure, you can enhance efficiency, increase profitability, and stay ahead of the competition.
So, what are you waiting for? The future of your business isn’t just around the corner—it’s already here. And it’s powered by AI.
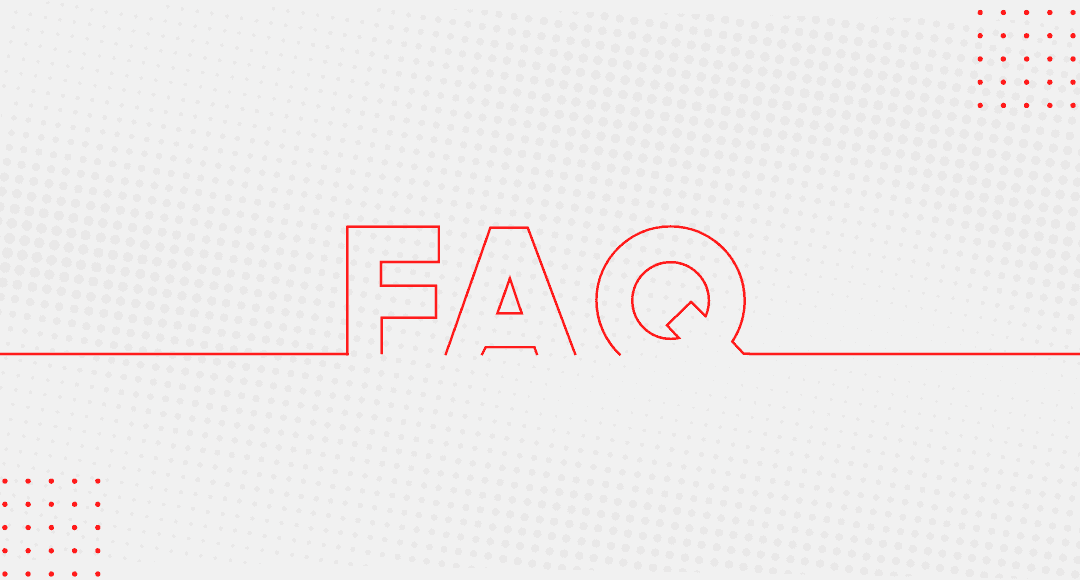
1. What exactly is a legacy system?
A legacy system is any technology, infrastructure, or software application that was designed and implemented many years ago, often decades. It’s often characterized by its lack of flexibility, inability to scale, and challenges integrating with newer systems. These systems, while functional, were built for a different era and are typically slower, harder to maintain, and unable to meet the demands of modern data processing.
2. Why are legacy systems so costly to maintain?
Legacy systems are costly because of the continuous need for patching, the scarcity of expertise, and the integration challenges they present. The cost also rises because these systems often need specific hardware and software to operate, both of which might be outdated and expensive to source. Further, legacy systems often require manual processes to perform what newer systems can automate, leading to lost productivity and increased labor costs.
3. How does AI help reduce operational costs associated with legacy systems?
AI reduces operational costs by automating manual tasks, enhancing decision-making through predictive analytics, and providing real-time insights that legacy systems can’t. It eliminates bottlenecks, reduces dependency on human intervention for repetitive processes, and allows data to be processed much more efficiently, which ultimately lowers labor and maintenance costs.
4. Is replacing a legacy system with AI always a complete overhaul?
No, AI integration does not have to be an all-or-nothing scenario. Companies can start with AI augmentation, where AI technologies enhance existing capabilities of legacy systems without a full replacement. For example, AI-driven chatbots can be added to customer support systems, or predictive analytics can be overlaid on existing supply chain data. This phased approach lowers risk and ensures smoother transitions.
5. What are the biggest risks associated with AI integration?
The biggest risks include compatibility issues with existing systems, the complexity of data migration, and potential downtime during the integration. There’s also the challenge of data quality; AI is only as effective as the data it processes. Legacy systems often have fragmented or poorly structured data, which can limit AI's effectiveness. To mitigate these risks, a well-planned phased integration is key, with a focus on enhancing data quality early on.
6. How can AI integration improve productivity?
AI improves productivity by automating time-consuming tasks, such as data entry, report generation, and routine customer service inquiries. By taking over these repetitive activities, AI frees up human workers to focus on more strategic and creative initiatives. It also enhances decision-making capabilities by providing real-time insights and predictive analytics, allowing teams to make informed decisions faster and with greater accuracy.
7. How can a company determine which AI tools are suitable for their legacy systems?
The key is to conduct a thorough system gap analysis. This involves assessing where your legacy systems fall short—whether in terms of speed, scalability, or accuracy. Once gaps are identified, companies can look for AI solutions that are compatible and modular, meaning they can integrate seamlessly without a full overhaul. Consulting AI vendors that offer integration services and seeking tools that support APIs for interoperability can help make the right choice.
8. Is the cost of AI integration justified compared to the maintenance of legacy systems?
While AI integration involves upfront investment, the long-term savings and benefits generally outweigh the costs associated with maintaining legacy systems. Legacy systems have high recurring costs, both direct and indirect, due to maintenance, human labor, and inefficiencies. AI integration not only lowers these maintenance costs but also drives revenue growth through enhanced customer experiences, operational efficiency, and improved decision-making capabilities.
9. How long does it typically take to integrate AI into legacy systems?
The timeline for AI integration can vary significantly depending on the complexity of the legacy system, the scope of the AI integration, and the readiness of the organization. A small pilot project might be implemented in 3-6 months, whereas a full-scale integration could take 12-18 months. Starting with small, scalable projects and gradually expanding can help reduce timelines and manage risks.
10. What skills are needed in a team to successfully integrate AI with legacy systems?
Successful integration requires a mix of skills. You need AI specialists and data scientists to develop and deploy AI models. Data engineers are crucial for managing and transforming data to ensure it's suitable for AI processing. Additionally, having IT professionals familiar with legacy systems helps to address compatibility issues, while change management experts ensure that teams are trained and that the transition is smooth. Collaboration between these roles ensures a well-rounded approach to AI integration.
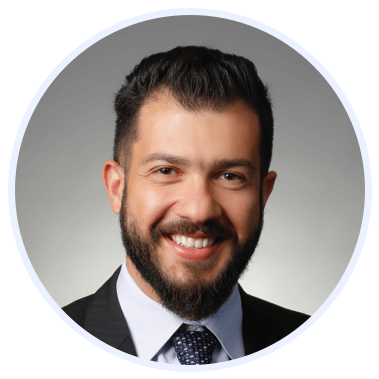
Rasheed Rabata
Is a solution and ROI-driven CTO, consultant, and system integrator with experience in deploying data integrations, Data Hubs, Master Data Management, Data Quality, and Data Warehousing solutions. He has a passion for solving complex data problems. His career experience showcases his drive to deliver software and timely solutions for business needs.