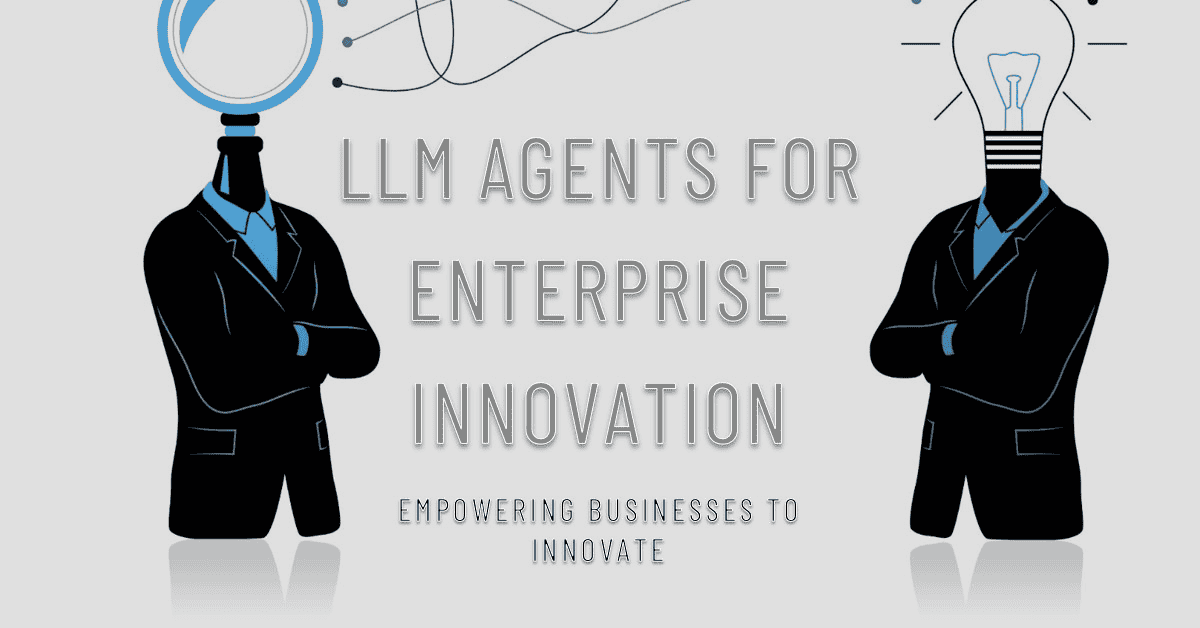
I remember the first time I saw a computer fill an entire room. It was 1985. I was fresh out of college, wide-eyed and ready to change the world. That behemoth of a machine, with its blinking lights and whirring tapes, was a marvel of its time. But it was just the beginning.
Fast forward to today. The phone in your pocket is thousands of times more powerful than that room-sized computer. And now, we're on the cusp of another revolution. It's called LLM agents.
Large Language Model agents. They're not just fancy chatbots or glorified search engines. They're the next big leap in enterprise technology. And they're about to change everything.
I've spent decades in the trenches of enterprise tech. From mainframes to the cloud, I've seen it all. But this? This is different. LLM agents are poised to revolutionize how businesses operate, innovate, and compete in ways we're only beginning to grasp.
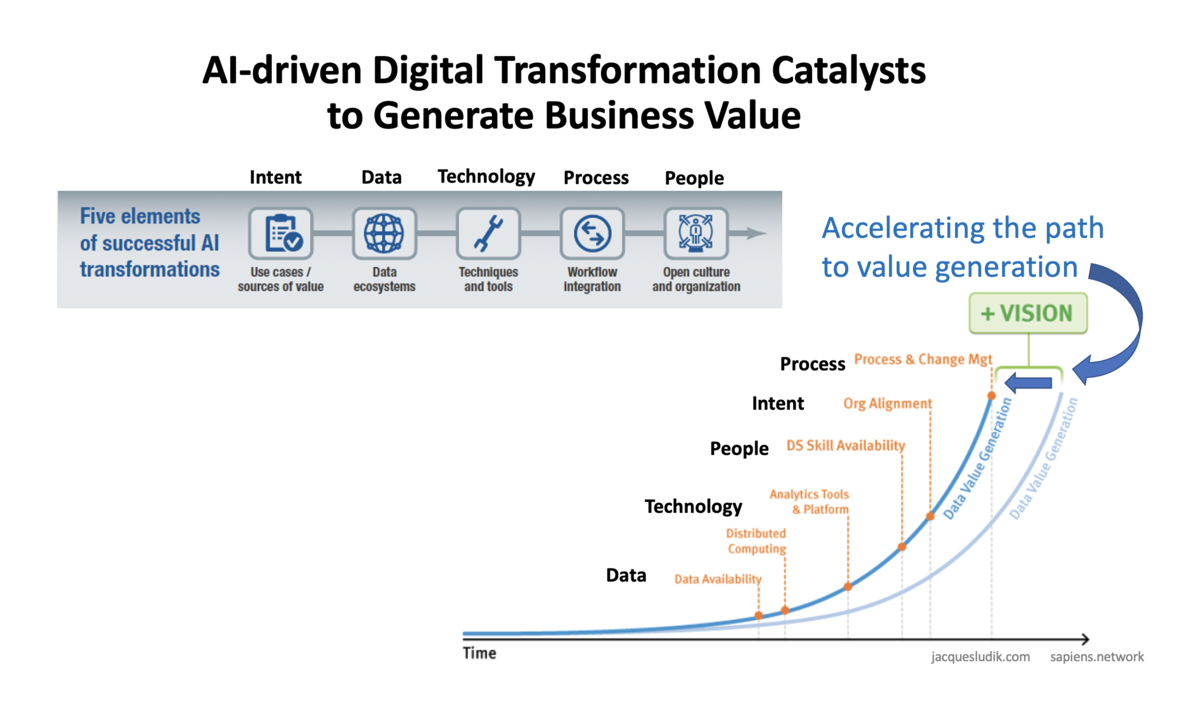
Imagine an AI assistant that doesn't just answer questions, but actively interfaces with your systems. It analyzes data, automates workflows, and generates insights that drive business decisions. All in real-time. All at a scale that would make that room-sized computer from 1985 look like an abacus.
But here's the kicker: This isn't science fiction. It's happening now. Today. In businesses across the globe. And if you're not paying attention, you're already falling behind.
Understanding LLM Agents

At their core, LLM agents are AI-powered systems built on large language models – sophisticated neural networks trained on vast amounts of text data. These agents can understand and generate human-like text, reason about complex problems, and even perform tasks that traditionally required human intelligence.
But here's where it gets interesting for enterprises: LLM agents aren't just glorified chatbots. They're intelligent assistants capable of interfacing with your existing systems, analyzing data, automating workflows, and generating insights that can drive business decisions.
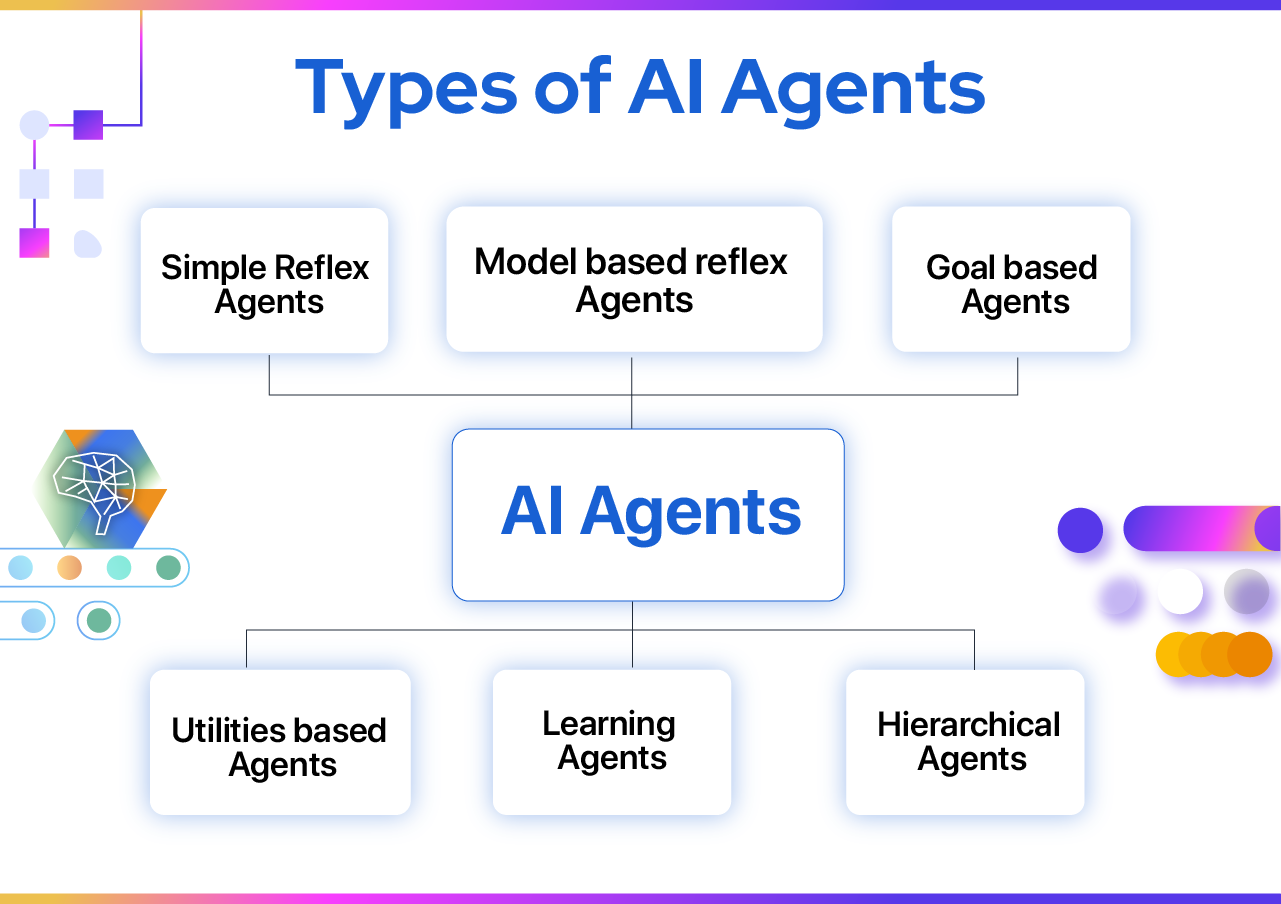
Consider this scenario:
In this example, our LLM agent isn't just passively responding to queries. It's actively pulling data from multiple systems, analyzing it using advanced language understanding, and pushing actionable insights back into the enterprise ecosystem. This level of integration and intelligence opens up possibilities that were previously unimaginable.
The Innovation Catalyst
So, how exactly do LLM agents drive innovation within enterprises? Let's break it down:
1. Accelerating Research and Development

In industries where R&D is the lifeblood of innovation – pharmaceuticals, technology, engineering – LLM agents are game-changers. They can sift through vast amounts of scientific literature, patents, and research papers at superhuman speeds, identifying patterns and connections that human researchers might miss.
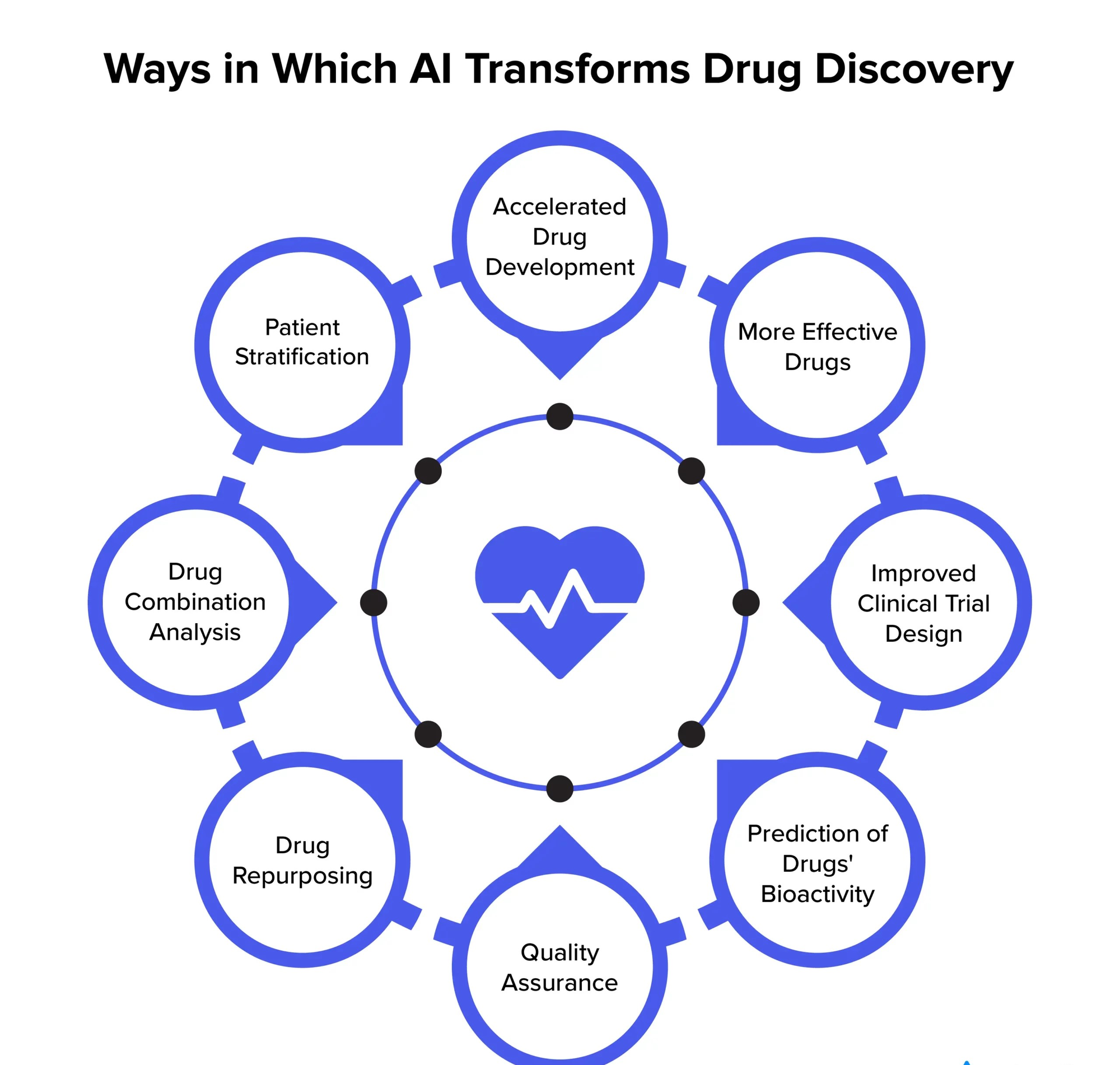
For instance, a pharmaceutical company I worked with implemented an LLM agent to assist in drug discovery. The agent was able to:
- Analyze millions of research papers and clinical trial results
- Identify potential drug candidates based on molecular structures
- Predict possible side effects and drug interactions
The result? A 30% reduction in early-stage drug discovery timelines and a significant increase in promising leads.
2. Enhancing Customer Experience

In today's hyper-competitive market, customer experience can make or break a business. LLM agents are revolutionizing how companies interact with and understand their customers.

Take the case of a major telecom provider that implemented an LLM-powered customer service agent:
This agent doesn't just provide scripted responses. It understands context, considers the customer's history, current network status, and formulates personalized, helpful responses. The result was a 40% reduction in call handling time and a 25% increase in customer satisfaction scores.
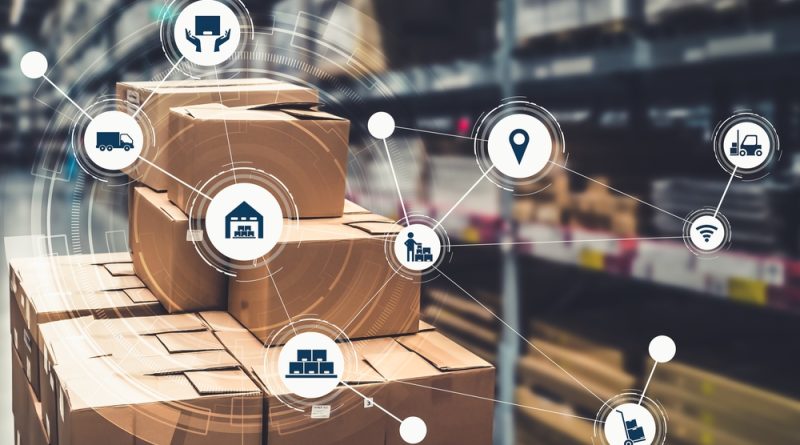
3. Streamlining Operations
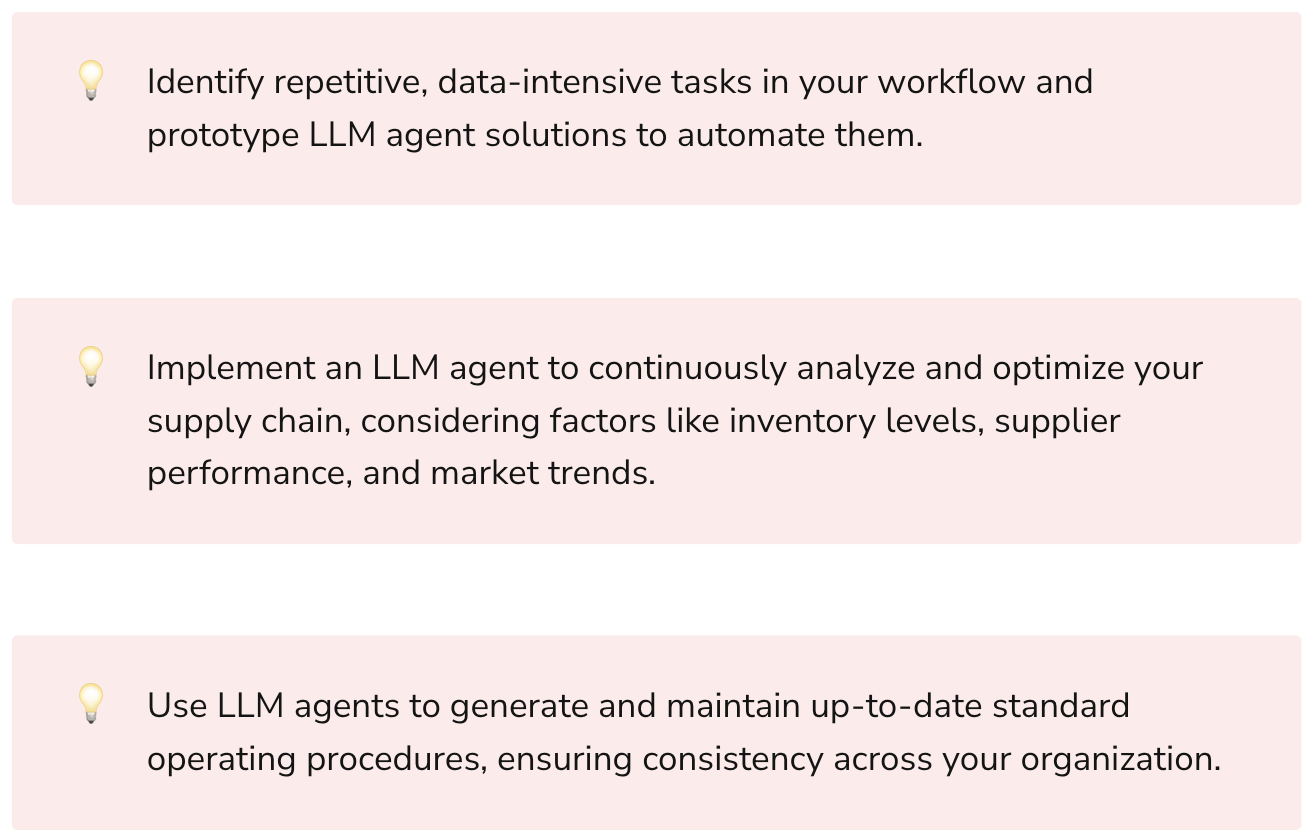
One of the most impactful applications of LLM agents is in streamlining complex business operations. These agents can automate and optimize processes that traditionally required significant human intervention.
For example, a global manufacturing company implemented an LLM agent to optimize its supply chain:
This agent analyzes complex data from multiple sources, considers various factors like inventory levels, supplier performance, and shipping routes, and generates optimized inventory strategies. The company reported a 15% reduction in inventory costs and a 20% improvement in order fulfillment rates.
Overcoming Implementation Challenges
While the potential of LLM agents is enormous, implementing them in enterprise environments comes with its own set of challenges. Here's how forward-thinking companies are addressing these:
1. Data Privacy and Security

LLM agents often require access to sensitive business data to function effectively. This raises legitimate concerns about data privacy and security.
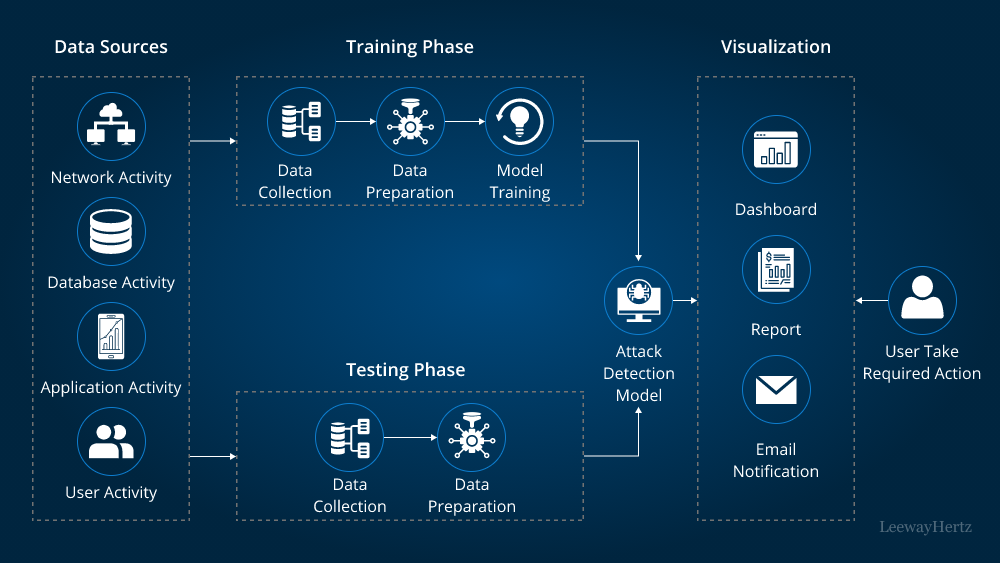
Solution: Implement a robust data governance framework. Use federated learning techniques where the LLM agent can learn from data without directly accessing it. Encrypt all data exchanges and implement strict access controls.
2. Integration with Legacy Systems

Many enterprises rely on legacy systems that weren't designed with AI integration in mind.
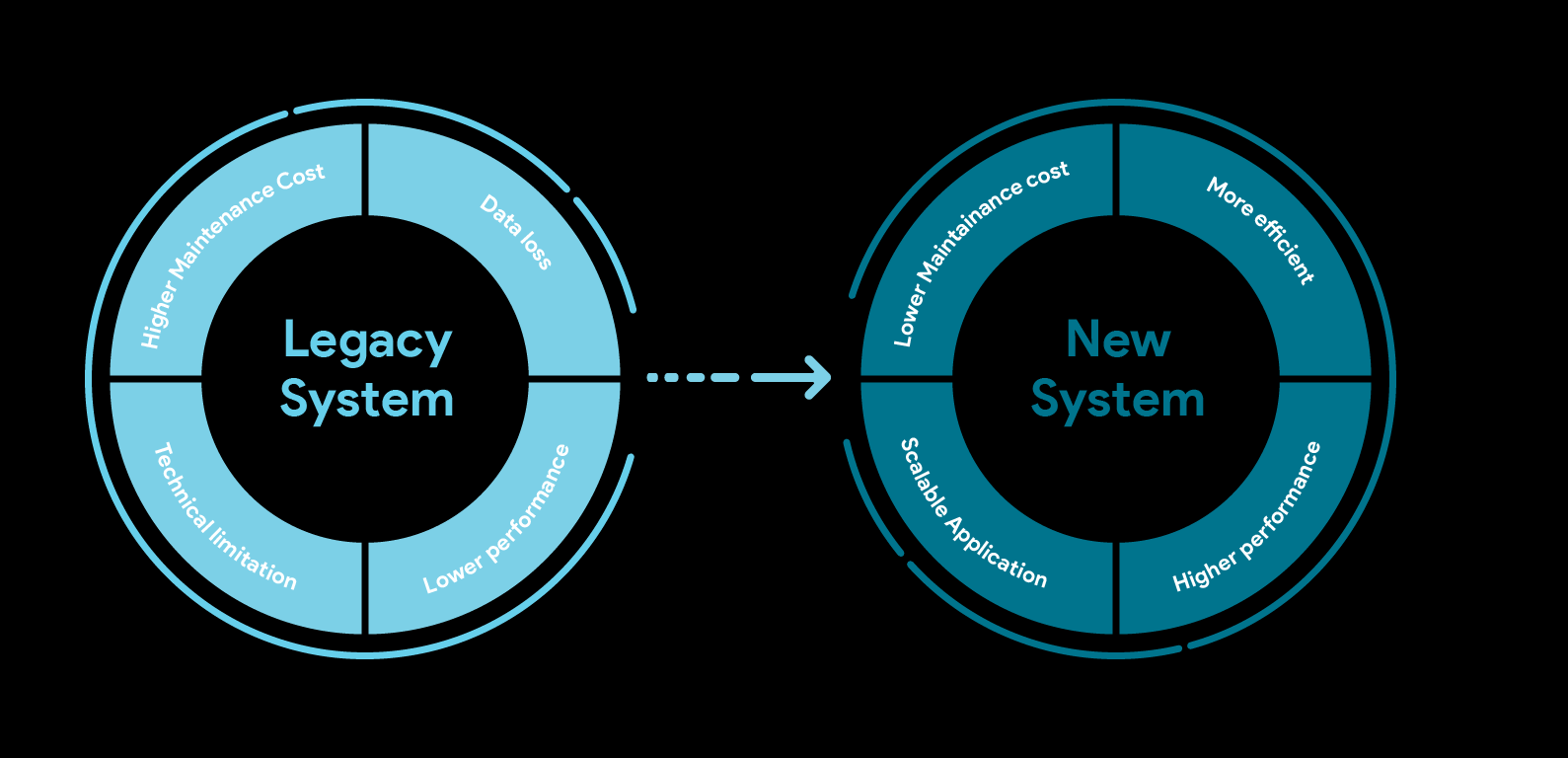
Solution: Develop middleware layers that can act as bridges between LLM agents and legacy systems. Gradually modernize core systems to be more AI-friendly. Consider using API-first approaches for new system developments.
3. Ethical Considerations

LLM agents can sometimes produce biased or inappropriate outputs, which can be particularly problematic in business contexts.
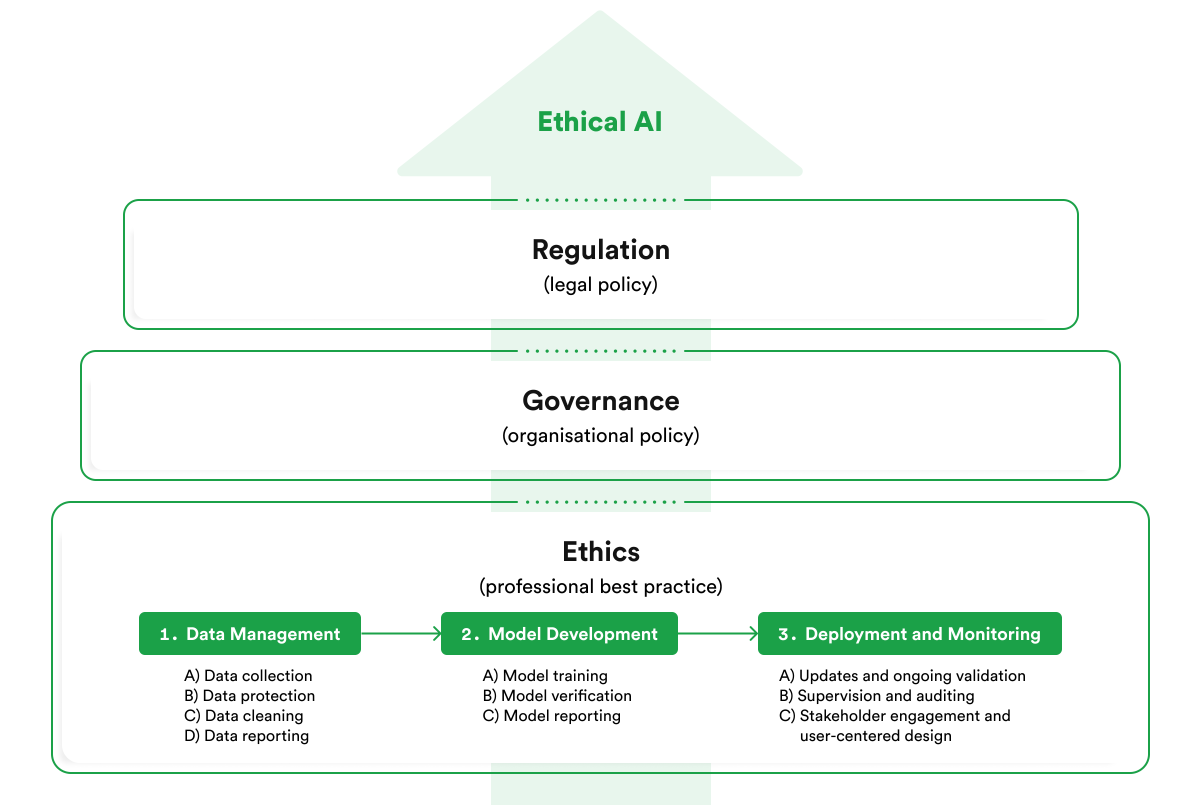
Solution: Implement rigorous testing and validation processes. Use techniques like constitutional AI to embed ethical guidelines directly into the LLM's training. Always maintain human oversight for critical decisions.
The Future of Enterprise Innovation
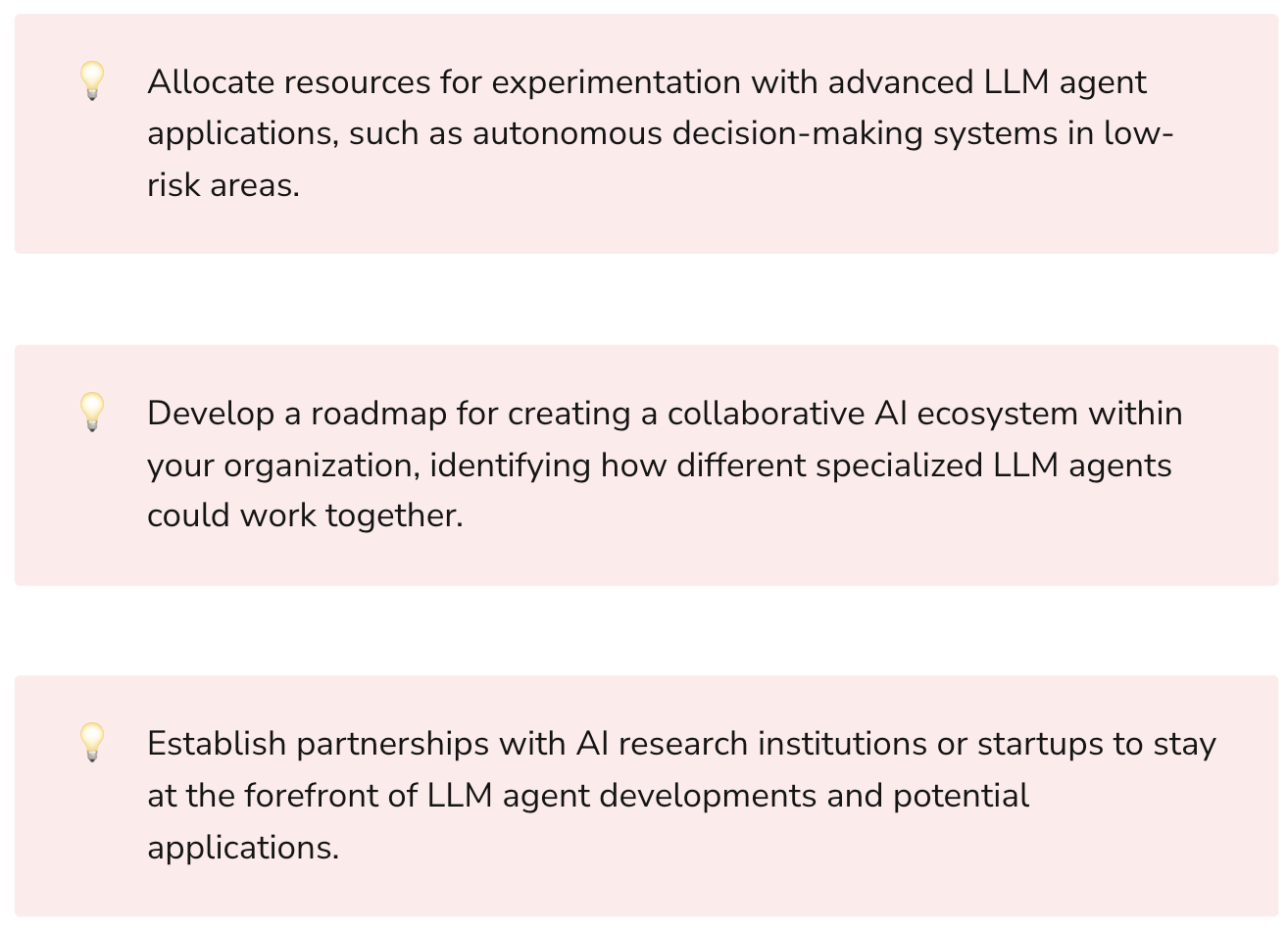
As we look to the future, the potential applications of LLM agents in driving enterprise innovation seem limitless. Here are some exciting possibilities on the horizon:
1. Autonomous Decision-Making Systems
Imagine LLM agents that can not only analyze data and provide recommendations but also make and implement decisions autonomously within predefined parameters.
This autonomous marketing agent could continuously optimize marketing spend across various platforms based on real-time performance data, dramatically improving ROI.
2. Predictive Innovation
LLM agents could analyze market trends, consumer behavior, and technological advancements to predict future innovation opportunities, giving companies a significant competitive edge.
3. Collaborative AI Ecosystems
Instead of isolated agents, we might see ecosystems of specialized LLM agents collaborating to solve complex business problems, mimicking human team dynamics but at an unprecedented scale and speed.
Final Thoughts
The integration of LLM agents into enterprise operations isn't just about adopting new technology – it's about fundamentally reimagining how businesses operate, innovate, and compete in the digital age.
For senior executives and decision-makers, the message is clear: LLM agents are not a far-off future technology. They're here now, and they're already transforming industries. The companies that will thrive in the coming years will be those that embrace this technology, experiment boldly, and reimagine their operations with LLM agents at the core.
The potential for innovation is immense, but so too is the risk of being left behind. As we stand on the brink of this new era, the question isn't whether LLM agents will transform your industry – it's whether you'll be leading that transformation or scrambling to catch up.
The future of enterprise innovation is here, powered by LLM agents. Are you ready to lead the charge?
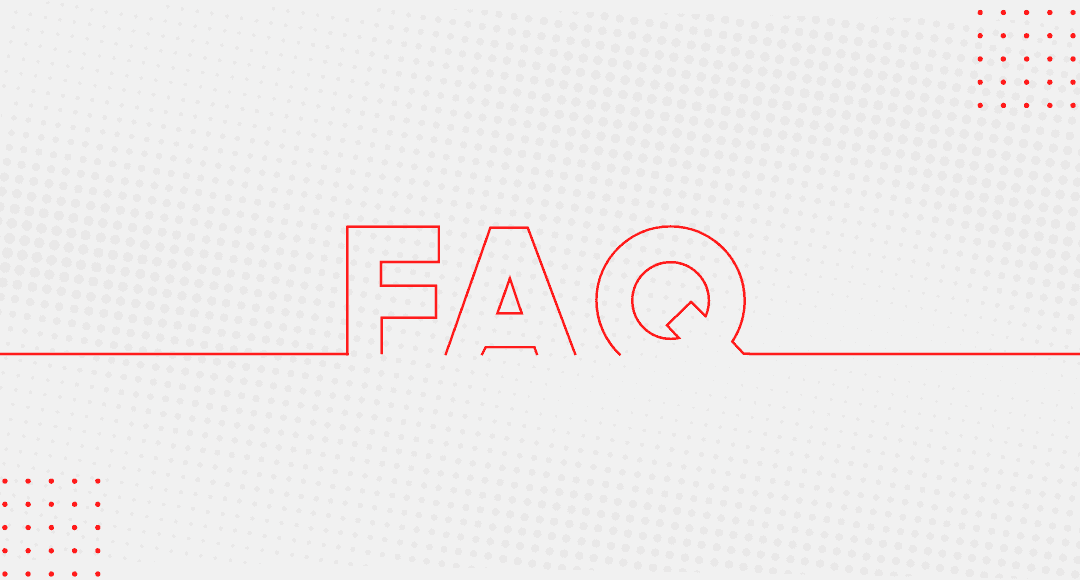
1. What exactly are LLM agents?
LLM agents are AI-powered systems built on large language models. They can understand and generate human-like text, reason about complex problems, and perform tasks that traditionally required human intelligence.
2. How do LLM agents differ from traditional chatbots?
Unlike traditional chatbots, LLM agents can understand context, learn from interactions, and perform complex tasks. They can interface with multiple systems, analyze data, and provide nuanced insights rather than just predefined responses.
3. What industries can benefit most from LLM agents?
While LLM agents have applications across all industries, they're particularly transformative in R&D-intensive fields like pharmaceuticals, technology companies with complex customer support needs, and industries with intricate supply chains like manufacturing and retail.
4. How can LLM agents drive innovation in enterprises?
LLM agents drive innovation by accelerating research, enhancing customer experiences, streamlining operations, and enabling predictive decision-making. They can analyze vast amounts of data, identify patterns, and generate insights at a scale and speed impossible for humans.
5. What are the main challenges in implementing LLM agents?
Key challenges include data privacy and security concerns, integration with legacy systems, ethical considerations around AI decision-making, and the need for specialized skills to develop and manage these systems effectively.
6. How can companies ensure data privacy when using LLM agents?
Companies should implement robust data governance frameworks, use federated learning techniques where possible, encrypt all data exchanges, and implement strict access controls. Regular audits and compliance checks are also crucial.
7. What skills do organizations need to successfully implement LLM agents?
Organizations need a mix of technical skills (like machine learning, data science, and software engineering) and domain expertise. Additionally, skills in AI ethics, prompt engineering, and change management are becoming increasingly important.
8. How can LLM agents improve customer experience?
LLM agents can provide personalized, 24/7 customer support, analyze customer feedback across channels to generate insights, and even predict customer needs before they arise. They can also help create tailored marketing content at scale.
9. What's the future of LLM agents in enterprise settings?
The future likely involves more autonomous decision-making systems, predictive innovation capabilities, and collaborative AI ecosystems where multiple specialized agents work together to solve complex business problems.
10. How should companies get started with LLM agents?
Companies should start by identifying specific use cases where LLM agents could add value. Begin with a pilot project in a non-critical area, invest in upskilling key staff, and gradually build a roadmap for wider implementation. It's crucial to establish clear governance and ethical guidelines from the outset.
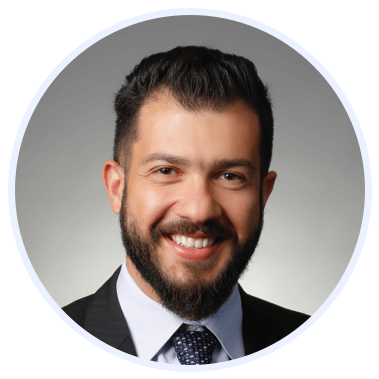
Rasheed Rabata
Is a solution and ROI-driven CTO, consultant, and system integrator with experience in deploying data integrations, Data Hubs, Master Data Management, Data Quality, and Data Warehousing solutions. He has a passion for solving complex data problems. His career experience showcases his drive to deliver software and timely solutions for business needs.