
The financial services industry is a highly regulated industry with multiple stakeholders, including customers, investors, and regulators, all of whom have high expectations for its safety, soundness, and reliability. As a result, risk management has become a crucial part of the financial services industry, and with the increasing amount of data available, the use of advanced analytics has become essential to navigate risk management challenges. In this blog, we will explore how advanced analytics can help navigate risk management challenges in the financial services industry.
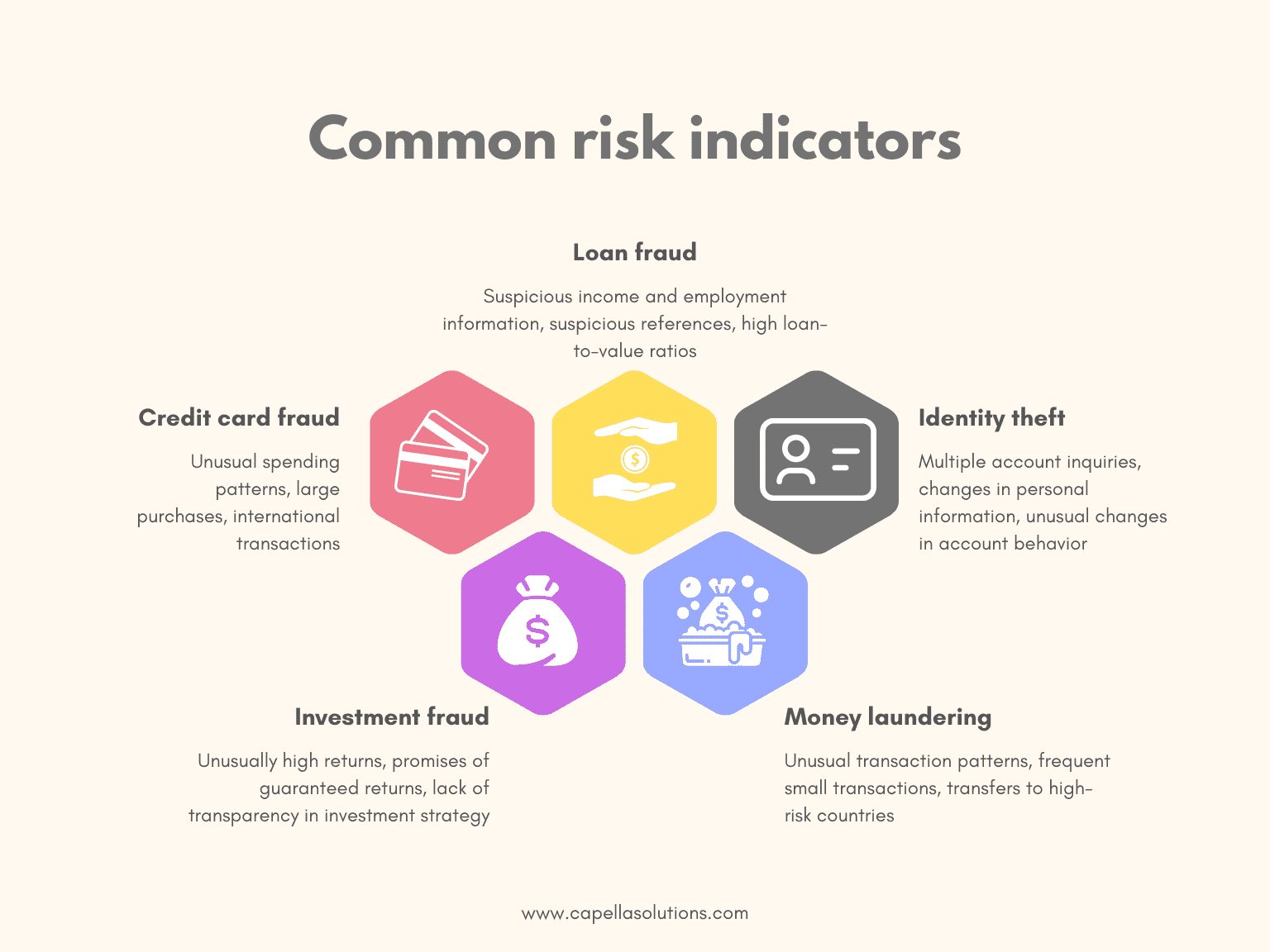
The Evolution of Risk Management in the Financial Services Industry
In the past, the risk management function in the financial services industry was primarily focused on compliance and control. However, after the 2008 financial crisis, regulators and stakeholders demanded a more comprehensive and integrated approach to risk management. As a result, the focus has shifted towards proactive risk management, which includes identifying, measuring, and managing risks across the entire organization.

The financial services industry faces a wide range of risks, including credit, market, operational, liquidity, and reputational risks. These risks can be difficult to manage, especially in a fast-paced, globalized business environment. Fortunately, advanced analytics can help navigate these challenges.
The Role of Advanced Analytics in Risk Management

Advanced analytics refers to the use of statistical and computational techniques to extract insights from data. With the large amount of data generated in the financial services industry, advanced analytics can help identify risks, measure their impact, and mitigate them before they become problems.
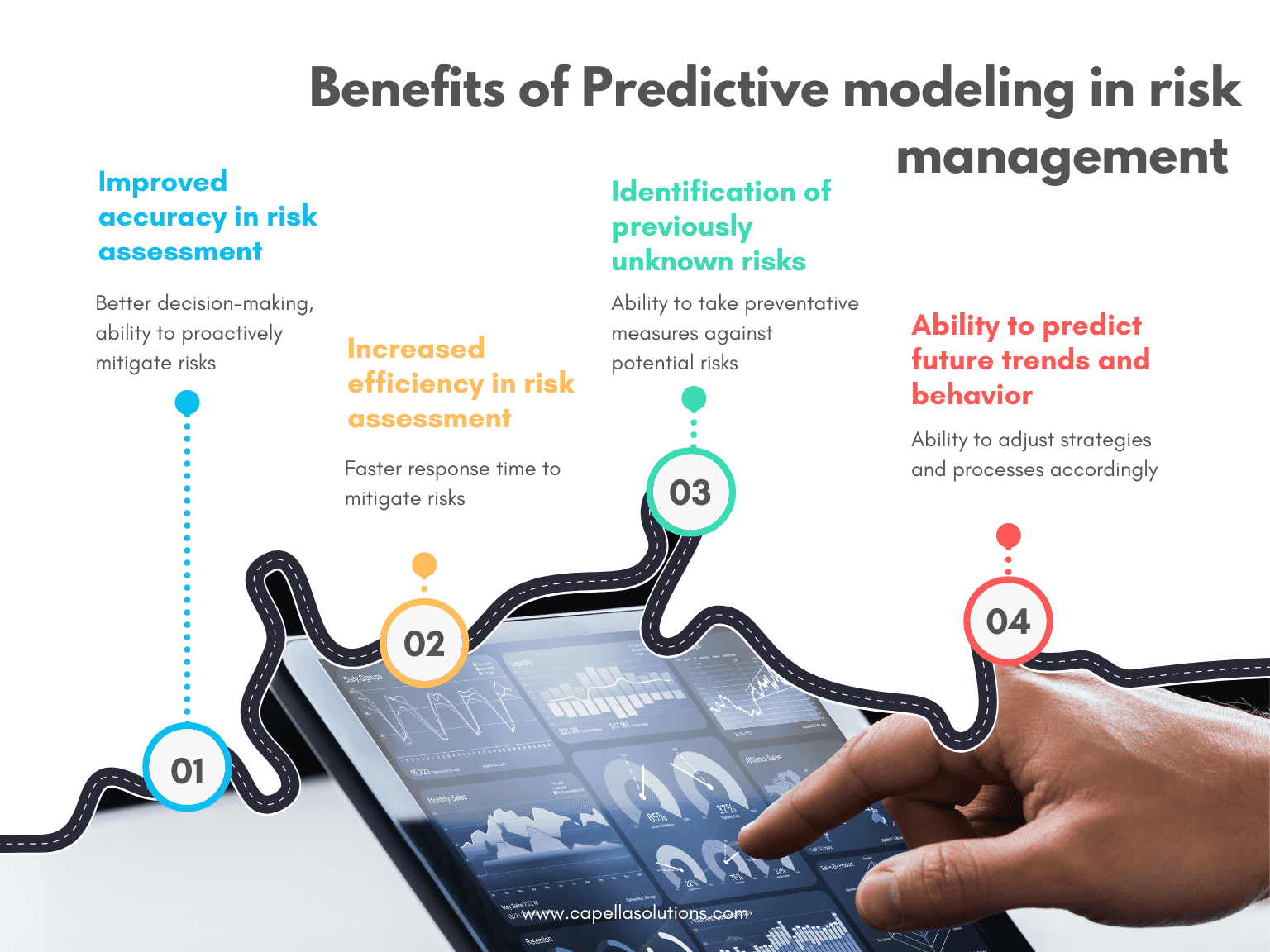
Here are some ways that advanced analytics can help navigate risk management challenges in the financial services industry:
Predictive Modeling
Predictive modeling uses statistical techniques to analyze historical data and identify patterns and relationships that can be used to predict future outcomes. In the financial services industry, predictive modeling can be used to:
- Identify potential defaults in loan portfolios
- Predict market movements and identify opportunities and risks
- Identify potential fraud and financial crime

Predictive modeling can be used to build more accurate risk models, which can help financial institutions manage their risks more effectively.
Machine Learning
Machine learning is a subset of artificial intelligence that uses algorithms to identify patterns in data. Machine learning can be used to:
- Identify unusual patterns in financial transactions
- Predict the likelihood of a customer defaulting on a loan
- Analyze social media sentiment to identify potential reputational risks
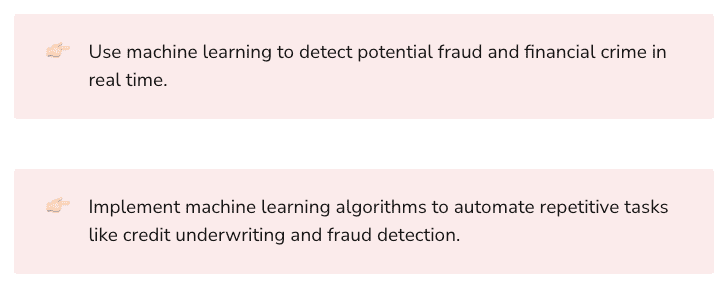
Machine learning can help financial institutions to quickly identify and mitigate risks before they become significant problems.
Natural Language Processing
Natural language processing (NLP) is a subset of artificial intelligence that allows computers to analyze human language. In the financial services industry, NLP can be used to:
- Analyze news articles and social media to identify potential market-moving events
- Identify potential fraud and financial crime
- Identify potential reputational risks
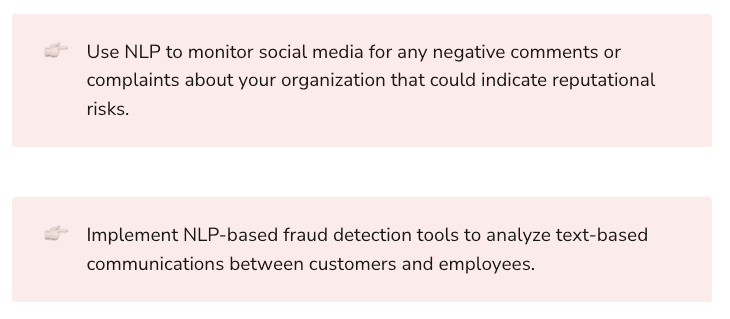
NLP can help financial institutions to quickly identify and mitigate risks that may have been missed by traditional methods.
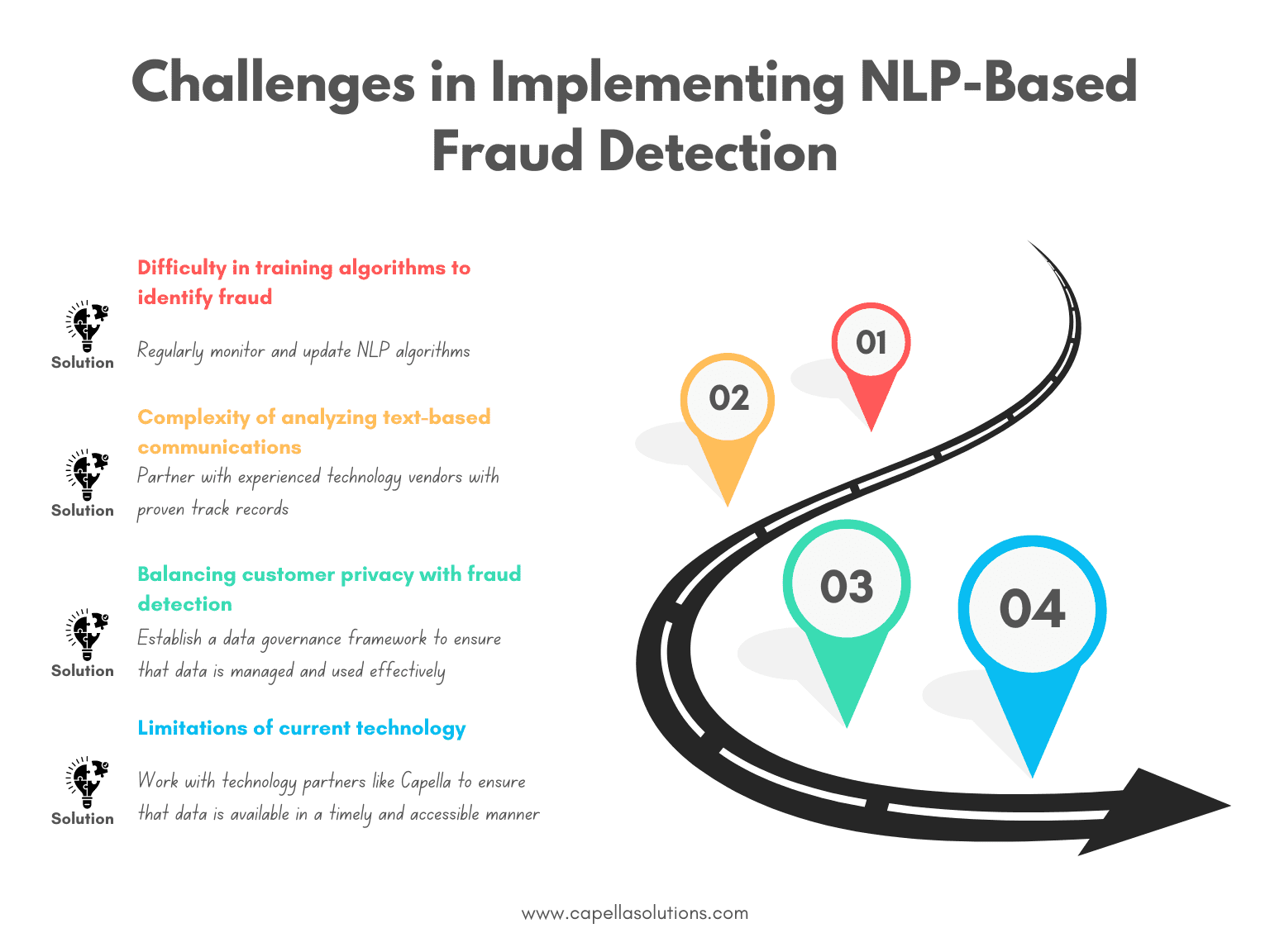
Network Analysis
Network analysis is a technique that analyzes the relationships between entities. In the financial services industry, network analysis can be used to:
- Identify potential money laundering and terrorist financing activities
- Identify connections between companies and individuals that may indicate potential financial crimes
- Identify potential reputational risks

Network analysis can help financial institutions to quickly identify and mitigate risks that may have been missed by traditional methods.
Advanced analytics can be a powerful tool for navigating risk management challenges in the financial services industry.
By leveraging predictive modeling, machine learning, and natural language processing, financial institutions can quickly identify and mitigate risks before they become significant problems.
However, organizations must be aware of the challenges associated with implementing advanced analytics for risk management, including data quality and availability and integration and implementation.
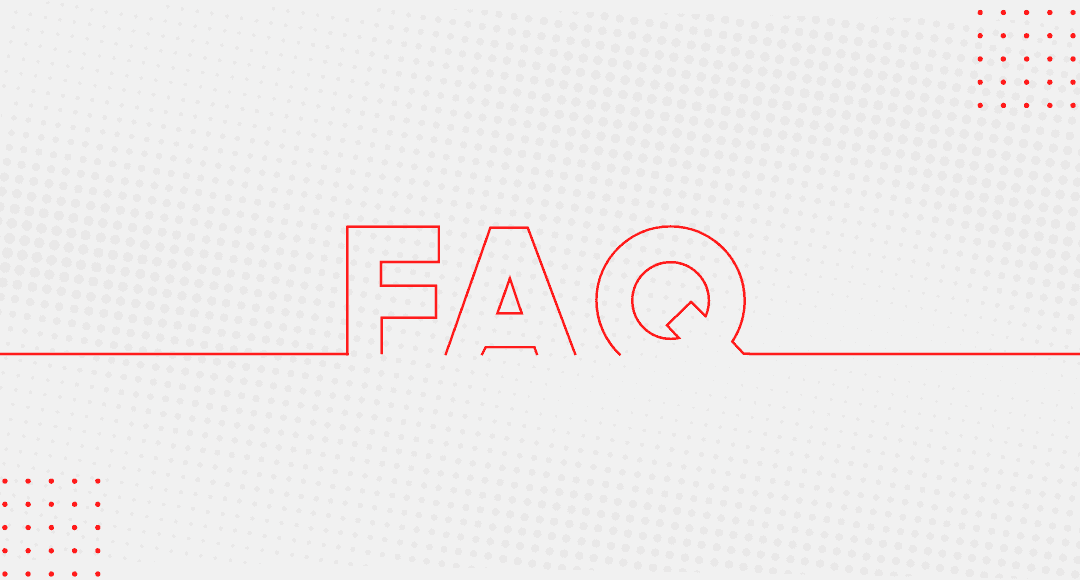
1. What is risk management in the financial services industry?
Risk management is the process of identifying, assessing, and prioritizing risks, followed by implementing strategies to minimize, monitor, and control the impact of potential risks. In the financial services industry, risk management is crucial for preventing financial losses due to a range of potential risks, including credit risk, market risk, operational risk, and regulatory risk.
2. What are the challenges of risk management in the financial services industry?
The financial services industry faces numerous challenges in risk management, including the complexity of financial products and services, rapidly evolving technologies, increased regulatory scrutiny, and growing cyber threats. Additionally, the sheer volume of data generated by financial transactions and market movements makes it difficult to quickly and accurately identify potential risks.
3. How can advanced analytics help in risk management?
Advanced analytics, such as machine learning and predictive modeling, can help financial institutions effectively manage risk by providing them with the tools to quickly and accurately analyze large volumes of data. These tools can help identify patterns and anomalies, forecast potential risks, and create predictive models to anticipate and mitigate potential losses.
4. What types of data can be used for risk management in financial services?
Financial institutions can use a variety of data sources for risk management, including market data, credit data, operational data, and customer data. These data sources can be used to identify potential risks, assess the likelihood of those risks, and develop strategies to mitigate the impact of those risks.
5. What is predictive modeling, and how can it be used in risk management?
Predictive modeling is a type of advanced analytics that uses statistical algorithms and machine learning techniques to analyze historical data and identify patterns that can be used to predict future outcomes. In risk management, predictive modeling can be used to forecast potential risks, assess the impact of those risks, and develop strategies to mitigate losses.
6. What are the benefits of using advanced analytics for risk management?
The benefits of using advanced analytics for risk management include improved accuracy and speed in identifying potential risks, the ability to create predictive models to anticipate and mitigate risks, and the ability to leverage large volumes of data to make more informed decisions. Additionally, advanced analytics can help financial institutions stay compliant with regulatory requirements and improve overall operational efficiency.
7. How can financial institutions implement advanced analytics for risk management?
Financial institutions can implement advanced analytics for risk management by first identifying the types of data they need to collect and analyze. Once the data sources have been identified, institutions can invest in the necessary technology and tools to process and analyze the data, including machine learning algorithms and predictive modeling software. Finally, institutions can train their staff to effectively use these tools to make informed decisions about risk management.
8. What are some common challenges in implementing advanced analytics for risk management?
Some common challenges in implementing advanced analytics for risk management include selecting the appropriate technology and tools for the specific needs of the institution, ensuring the quality and accuracy of data, managing the large volumes of data generated by financial transactions, and training staff to effectively use these tools to make informed decisions.
9. How can financial institutions stay up-to-date with advances in risk management technology?
Financial institutions can stay up-to-date with advances in risk management technology by regularly attending industry conferences and training sessions, engaging with industry experts, and networking with other financial professionals. Additionally, institutions can leverage research and development initiatives to stay ahead of the curve in terms of risk management technology.
10. How can financial institutions balance risk management with business objectives?
Financial institutions can balance risk management with business objectives by developing a risk management strategy that aligns with the overall goals of the institution. This strategy should take into account the specific risk profile of the institution and the risk appetite of stakeholders.
Financial institutions should regularly review and update their risk management strategies to ensure they are in line with the changing risk landscape and business objectives. It is essential to strike a balance between risk management and business objectives to ensure long-term sustainability and profitability.
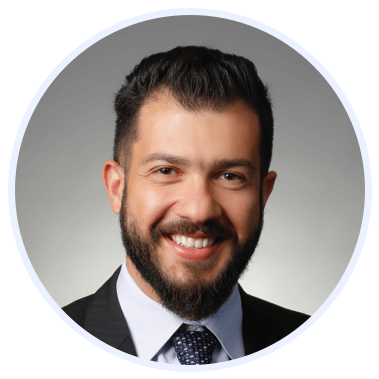
Rasheed Rabata
Is a solution and ROI-driven CTO, consultant, and system integrator with experience in deploying data integrations, Data Hubs, Master Data Management, Data Quality, and Data Warehousing solutions. He has a passion for solving complex data problems. His career experience showcases his drive to deliver software and timely solutions for business needs.