
Operational Data Hubs (ODH) are increasingly becoming a crucial part of modern organizations' data management strategies. Often compared to a Data Lake, an ODH is a centralized platform that collects, stores, and processes data from various sources in real-time. It provides a single point of access for various applications and analytics tools to access and analyze data, enabling organizations to make data-driven decisions and improve operational efficiency.
In this blog, we'll take a deep dive into ODHs, their key features, benefits, and how they differ from Data Lakes. We'll also discuss some best practices for implementing an ODH and provide examples of organizations that have successfully leveraged ODHs to drive business value.

What is an Operational Data Hub?
An ODH is a central platform that collects, stores, and processes data from various sources in real-time. It provides a single point of access for various applications and analytics tools to access and analyze data. Unlike Data Lakes, which are often used for storing large amounts of data in raw form, ODHs are designed to provide immediate access to data for operational purposes.
ODHs are often implemented using a distributed architecture, where data is collected from various sources, processed, and then stored in a central repository. This architecture enables organizations to scale their data management capabilities as the data volumes increase. ODHs also provide built-in security and governance features, ensuring that data is accessed only by authorized users and applications.

Key Features of an Operational Data Hub
There are several key features that differentiate an ODH from other data management platforms. Some of the most notable features include:
- Real-time data processing: ODHs are designed to process data in real-time, enabling organizations to access data as soon as it is generated. This enables organizations to make data-driven decisions and improve operational efficiency.
- Centralized data repository: ODHs provide a central repository for storing data from various sources. This enables organizations to access data from a single location, making it easier to analyze and gain insights.
- Distributed architecture: ODHs are often implemented using a distributed architecture, enabling organizations to scale their data management capabilities as data volumes increase.
- Built-in security and governance: ODHs provide built-in security and governance features, ensuring that data is accessed only by authorized users and applications. This helps organizations comply with data privacy regulations and maintain data integrity.
Benefits of Implementing an Operational Data Hub
There are several benefits of implementing an ODH, including:
- Improved operational efficiency: By providing real-time access to data, ODHs enable organizations to make data-driven decisions and improve operational efficiency. This can help organizations reduce costs, improve customer service, and increase productivity.
- Enhanced data accessibility: ODHs provide a single point of access for data from various sources, making it easier for organizations to access and analyze data. This can help organizations gain insights and make better decisions.
- Increased data scalability: ODHs are designed to handle large volumes of data, enabling organizations to scale their data management capabilities as data volumes increase.
- Improved data security and governance: ODHs provide built-in security and governance features, ensuring that data is accessed only by authorized users and applications. This helps organizations maintain data integrity and comply with data privacy regulations.
How Does an ODH Differ from a Data Lake?
ODHs are often compared to Data Lakes, as both are central platforms for storing and managing data. However, there are some key differences between the two:
- Data processing: ODHs are designed to process data in real-time, enabling organizations to access data as soon as it is generated. In contrast, Data Lakes are often used to store large amounts of data in raw form, without any processing.
- Data accessibility: ODHs provide immediate access to data for operational purposes, enabling organizations to make data-driven decisions and improve operational efficiency. Data Lakes, on the other hand, are often used for batch processing and analytics, providing access to data for analytical purposes.
- Architecture: ODHs are often implemented using a distributed architecture, enabling organizations to scale their data management capabilities as data volumes increase. Data Lakes, on the other hand, are typically implemented using a centralized architecture.
- Governance: ODHs provide built-in security and governance features, ensuring that data is accessed only by authorized users and applications. Data Lakes, on the other hand, do not have such built-in features and require additional governance tools to ensure data integrity and compliance with data privacy regulations.
Best Practices for Implementing an ODH
Implementing an ODH requires careful planning and execution. Here are some best practices for implementing an ODH:
- Define your data management strategy: Before implementing an ODH, it's important to define your data management strategy. This should include defining your data sources, data governance policies, and the data analytics and applications that will access the ODH.
- Choose the right technology: There are several technology options for implementing an ODH, including Hadoop, Apache Spark, and cloud-based solutions. It's important to choose the technology that best fits your organization's needs and requirements.
- Ensure data quality: Data quality is crucial for the success of an ODH. It's important to ensure that the data collected from various sources is accurate, consistent, and relevant. This can be achieved through data cleansing, transformation, and validation processes.
- Implement security and governance: ODHs provide built-in security and governance features, but it's important to implement additional measures to ensure data integrity and compliance with data privacy regulations. This can include implementing access controls, data encryption, and auditing mechanisms.
Examples of Organizations Leveraging ODHs
There are several organizations that have successfully implemented ODHs and are leveraging them to drive business value. Some examples include:
- Walmart: Walmart implemented an ODH to collect and process data from various sources in real-time. This enabled the company to improve its inventory management and supply chain operations, resulting in increased efficiency and cost savings.
- JPMorgan Chase: JPMorgan Chase implemented an ODH to collect and process data from various sources, including customer transactions, market data, and internal systems. This enabled the company to improve its risk management and compliance processes, ensuring that data is accessed only by authorized users and applications.
- Netflix: Netflix implemented an ODH to collect and process data from various sources, including customer data, streaming data, and internal systems. This enabled the company to improve its recommendation algorithms and provide personalized content recommendations to its customers.
Operational Data Hubs are increasingly becoming a crucial part of modern organizations' data management strategies. With real-time data processing, centralized data repository, and built-in security and governance features, ODHs provide a single point of access for data from various sources, enabling organizations to make data-driven decisions and improve operational efficiency.
While there are some similarities between ODHs and Data Lakes, there are also some key differences in terms of data processing, accessibility, architecture, and governance. Organizations looking to implement an ODH should carefully plan and execute their implementation, following best practices such as defining their data management strategy, choosing the right technology, ensuring data quality, and implementing security and governance measures.
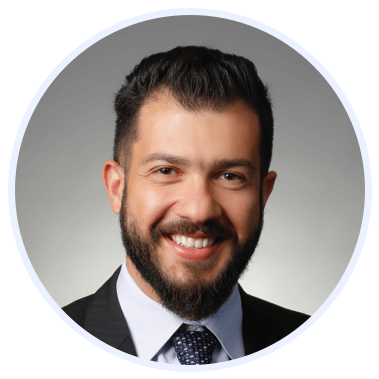
Rasheed Rabata
Is a solution and ROI-driven CTO, consultant, and system integrator with experience in deploying data integrations, Data Hubs, Master Data Management, Data Quality, and Data Warehousing solutions. He has a passion for solving complex data problems. His career experience showcases his drive to deliver software and timely solutions for business needs.