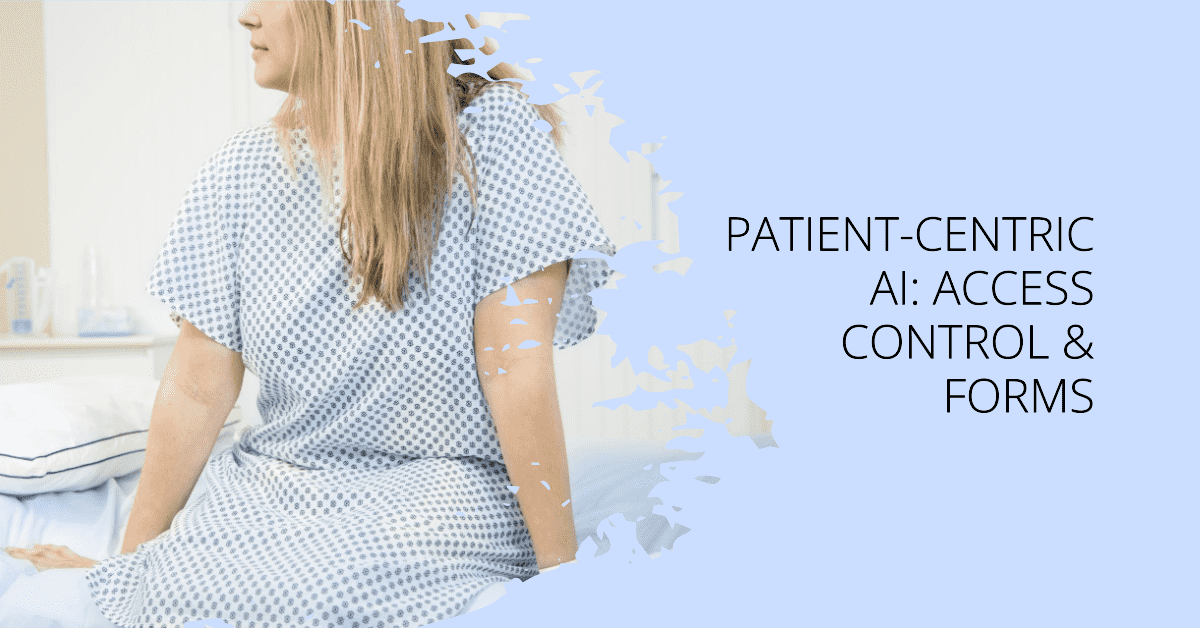
Healthcare organizations today are faced with a challenging balancing act - providing personalized, empathetic patient care while also ensuring compliance with strict data privacy regulations. This is where patient-centric AI comes in. AI-powered solutions allow providers to automate administrative tasks, freeing up more time for meaningful doctor-patient interactions. At the same time, AI enables granular access control and facilitates consent processes to protect patient data privacy.

In this blog post, we'll explore how healthcare organizations can leverage AI to put patients at the center of care while also securing sensitive health data.

The Promise and Peril of Healthcare Data

Electronic health records (EHRs) have become indispensable in modern medicine. EHRs allow providers to quickly access patient medical history, coordinate care, reduce mistakes due to illegible handwriting, and more. However, digitization of health data also introduces risks:
- Privacy violations: Medical records contain highly sensitive personal information. Unauthorized access can lead to identity theft, insurance fraud, and other issues.
- Compliance burdens: Strict regulations like HIPAA impose complex security and privacy requirements for working with healthcare data. Non-compliance results in heavy penalties.
- Administrative overload: Doctors spend over 50% of their day on EHR data entry instead of actual patient care.
Artificial intelligence can help address these challenges in a patient-centric manner. Let's look at two key applications: access control and consent management.
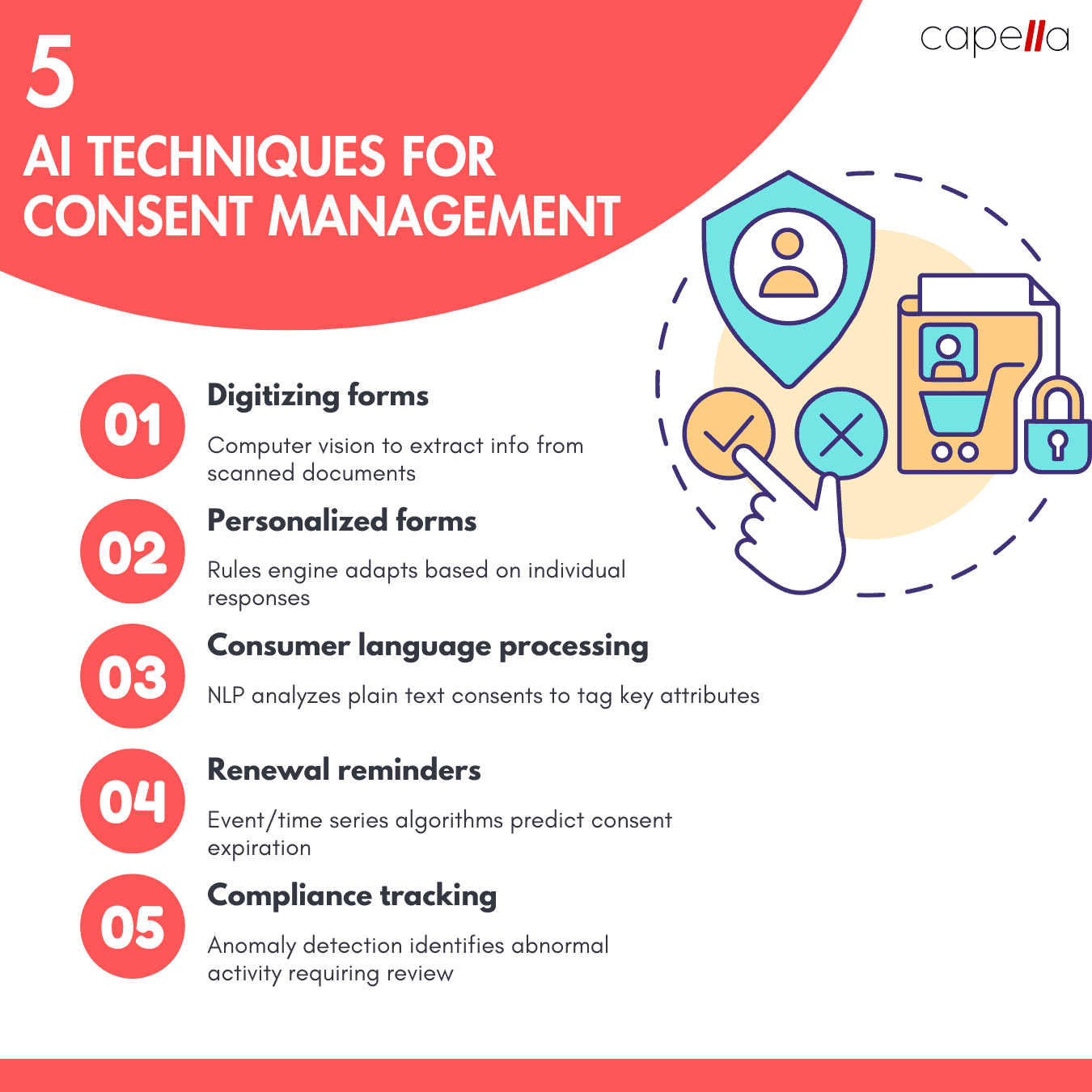
Secure and Granular Access Control with AI

Role-based access control (RBAC) is a popular technique to manage access to sensitive data. Users are assigned roles like "doctor", "nurse", "billing specialist" etc. Roles are then granted access to certain data or functions.
However, RBAC has limitations in healthcare:
- A "one-size-fits-all" access policy cannot capture nuances across departments, care settings, and individual provider preferences.
- Static roles don't reflect dynamic shifts in user responsibilities over time.
AI-powered user provisioning solutions offer more granular and intelligent access control:
- AI analyzes healthcare workflows, user behavior patterns, and compliance policies to create customized data access mappings.
- Instead of broad roles like "doctor", personas are defined at individual user or department level based on context.
- Access permissions are automated and continually optimized instead of manual role assignments.
Let's look at an example:

The AI has granted different data access to oncologists based on their department's specialty area, capturing finer nuances. The dietician's access is read-only as their job does not require modifying health records.
These personalized policies are automatically enforced when a user tries to access patient data via the EHR system or other apps. The AI tracks activity and continually refines access mapping as roles evolve.
Benefits of Intelligent Access Management:
- Improved security: Granular need-to-know access minimizes insider threat.
- Enhanced privacy: Only necessary data exposed based on user context.
- Better compliance: Adaptive controls proactively prevent unauthorized access.
- Higher productivity: No excessive privileges means less audit logs to comb through.
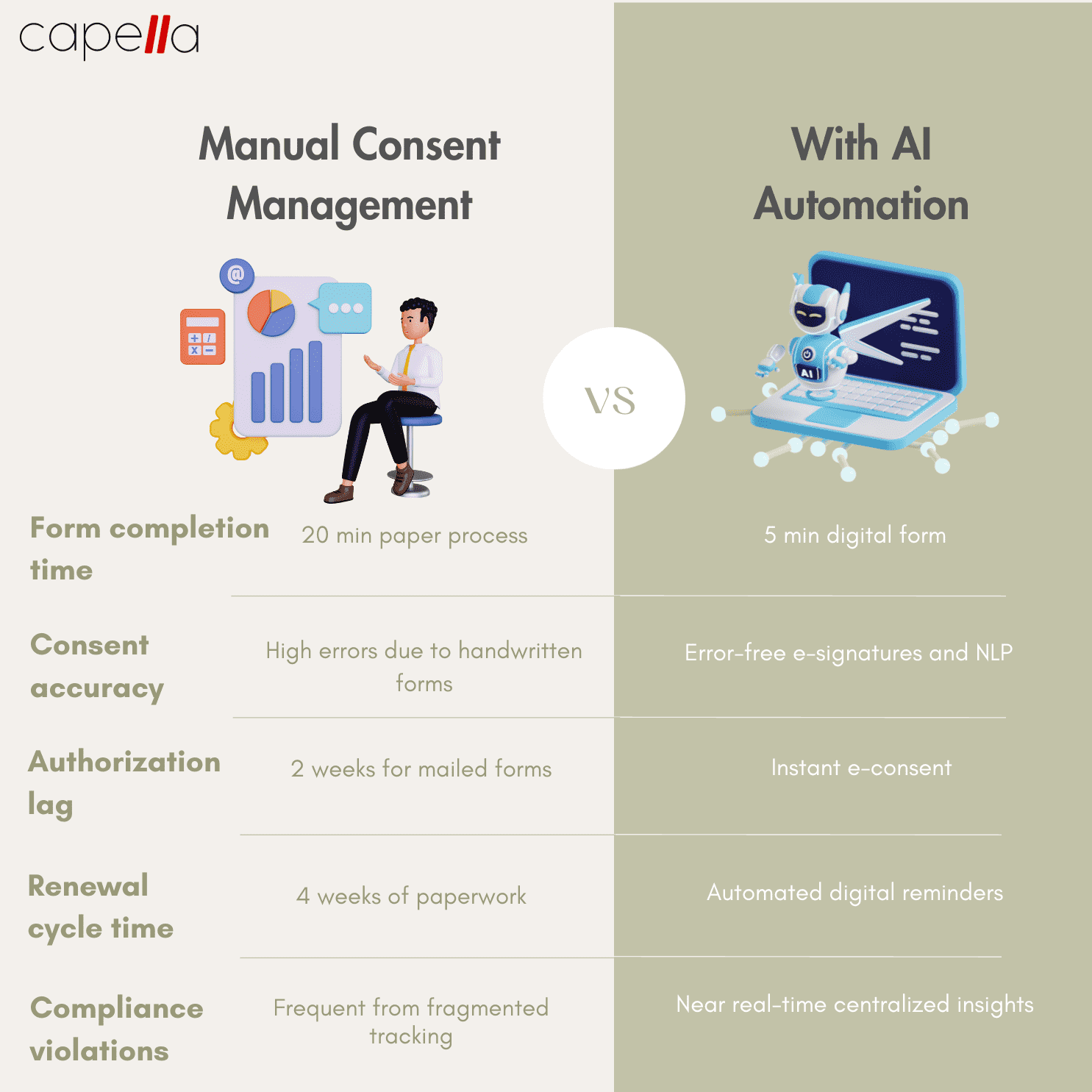
Smarter Consent Management and Forms with AI
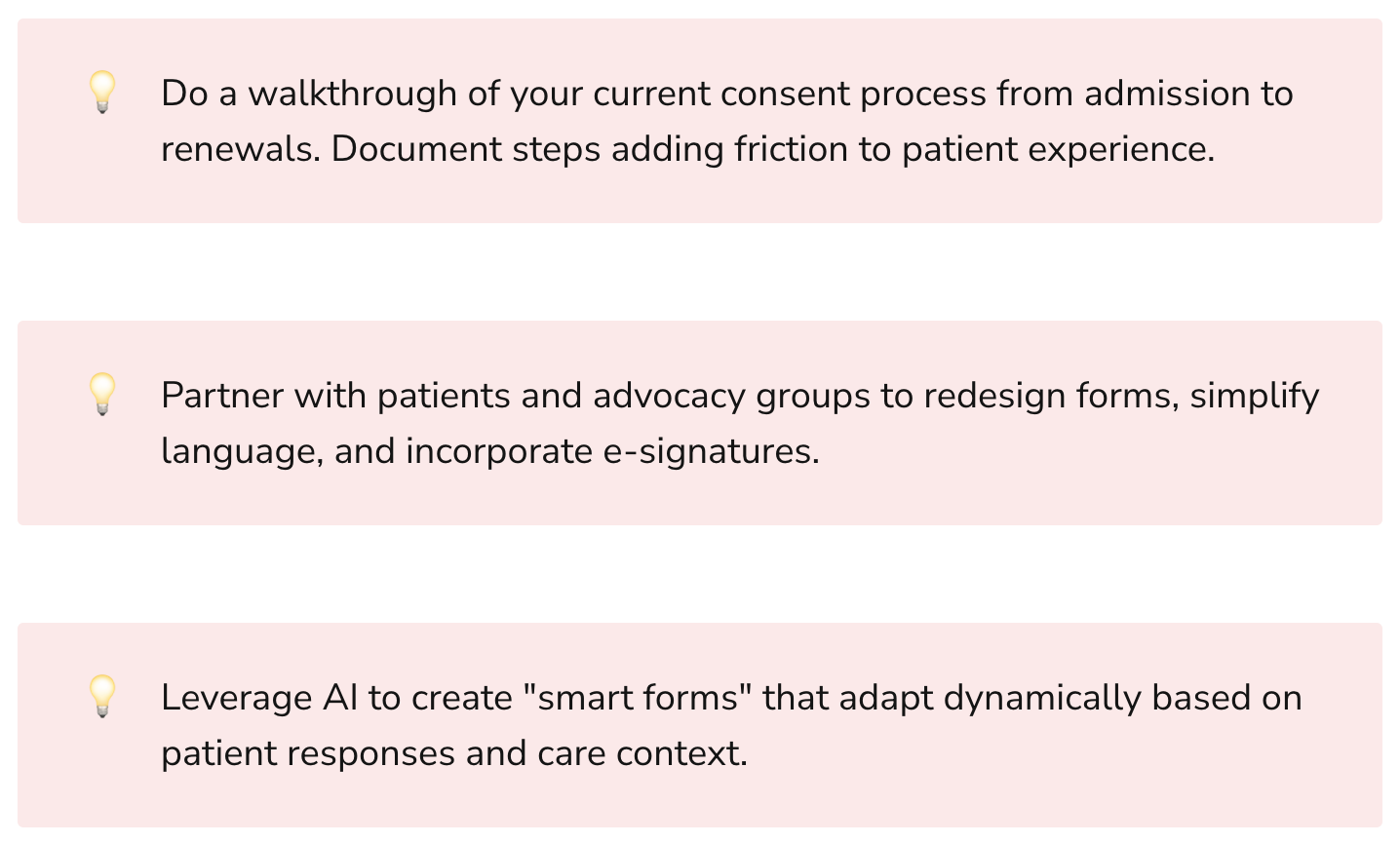
Patients provide consent in many contexts - signing general privacy terms upon hospital admission, giving permission for specific procedures, applying for financial assistance, and more.
Managing consent end-to-end while providing a positive patient experience presents challenges:
- Tedious paper forms: Printing, scanning, mailing, faxing and manual filing of forms is time-consuming.
- Fragmented systems: Consents are stored across EHR, billing, and other systems leading to blindspots.
- Lack of audit trails: Hard to track consent validity, renewals, withdrawals and amendments.
- Disengaged patients: Complex legalese and excessive paperwork hinders transparency.
Once again, AI and automation provides a patient-centric solution:
- Digital forms: Patients can easily fill consent, intake, and assessment forms on any device. eSignatures enable paperless workflows.
- Centralized management: All forms and consent data integrated across platforms via APIs.
- Process intelligence: Rules engine tracks cycles, sends renewal reminders, and monitors compliance.
- Natural Language Processing (NLP): AI reads free text consumer consents to auto-tag attributes like dates, care activities, information access, etc.
- Multilingual: Machine translation bridges language barriers by supporting patient-preferred languages.
Let's visualize how an automated solution enhances the consent procedure:
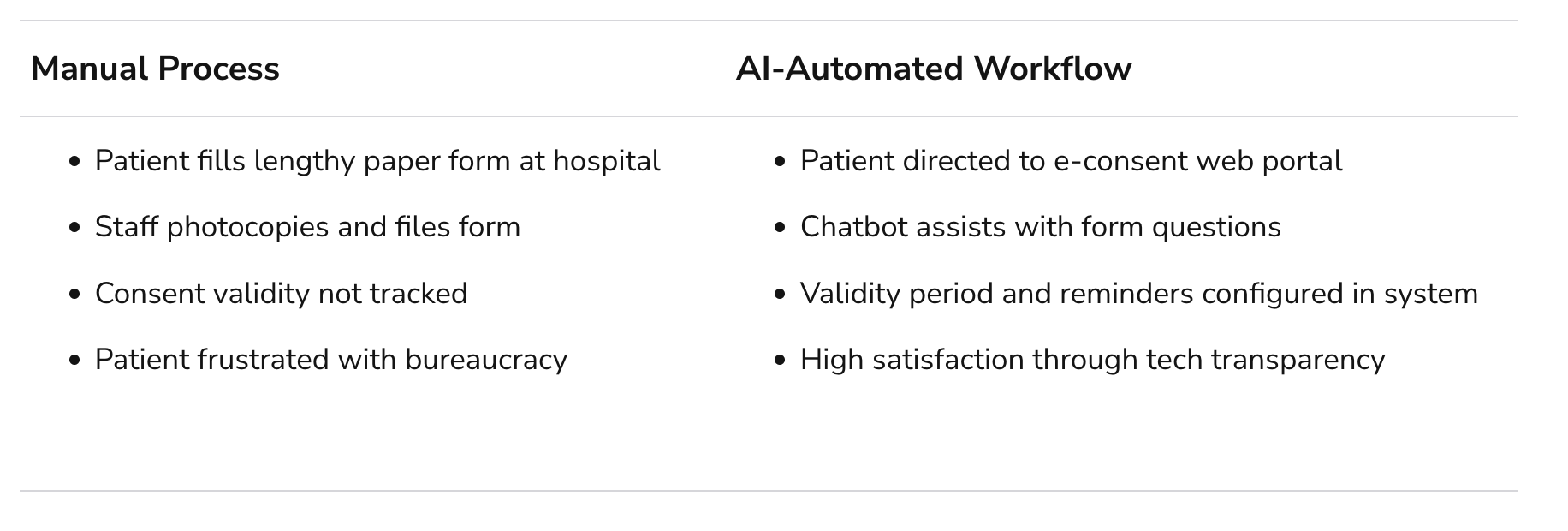
The AI-powered workflow provides a transparent, engaging experience while ensuring continuous compliance. Patients are empowered with the convenience of digital platforms rather than being blocked by paperwork.
Real World Examples
Let's look at a couple real world examples of AI enabling patient-centric yet secure health data management:
Careology - Consent Management Platform
Careology offers Avalon, an enterprise SaaS platform for consent, waiver and visit management. The system integrates with EHRs like Epic and Cerner to provide features like:
- DigitalAdaptive forms covering 150+ consent templates
- Configurable conditional logic adapts questions based on user responses
- Patient portal to complete forms remotely
- Supply patient-education materials to enhance comprehension
- Automate state-specific consent procedures and renewals
- Analytics dashboard to identify bottlenecks
By streamlining consent workflows, Careology allows healthcare staff to dedicate more time to patient experience while also achieving continuous compliance.
DataRobot - AI-driven User Access Controls
Leading enterprise AI vendor DataRobot applies ML algorithms to learn user behavior patterns and implement least privilege access. Instead of broad roles, DataRobot builds statistical models to analyze individual user data access tendencies. These models then enable dynamically adaptive access policies tailored to specific users.
DataRobot's AI-powered access governance greatly reduces security risks while optimizing productivity. Healthcare organizations can leverage the personalization and automation capabilities to manage consents and other patient data securely.
Key Takeaways
Some key points covered in this article:
- Electronic health records introduce data privacy and compliance challenges due to their sensitivity.
- Rigid role-based access control strategies fail to capture nuances in healthcare workflows.
- AI enables granular, contextual user access policies that automatically adapt to changes.
- Consent management is fraught with fragmented systems, renewal tracking issues, and poor patient experience.
- AI automation streamlines consent workflows by applying NLP, rules engines, mobility and other technologies.
- Leading healthcare IT vendors offer AI platforms purpose-built for user provisioning, consent management and privacy.
Patient-centricity is no longer just a buzzword - it is now a competitive necessity. With the help of AI, healthcare organizations can enable patient-focused experiences while also securing sensitive personal data. Consent and access control are two areas where AI can drive major improvements.
What other ways are you applying AI to balance empathetic care with rigorous data governance?
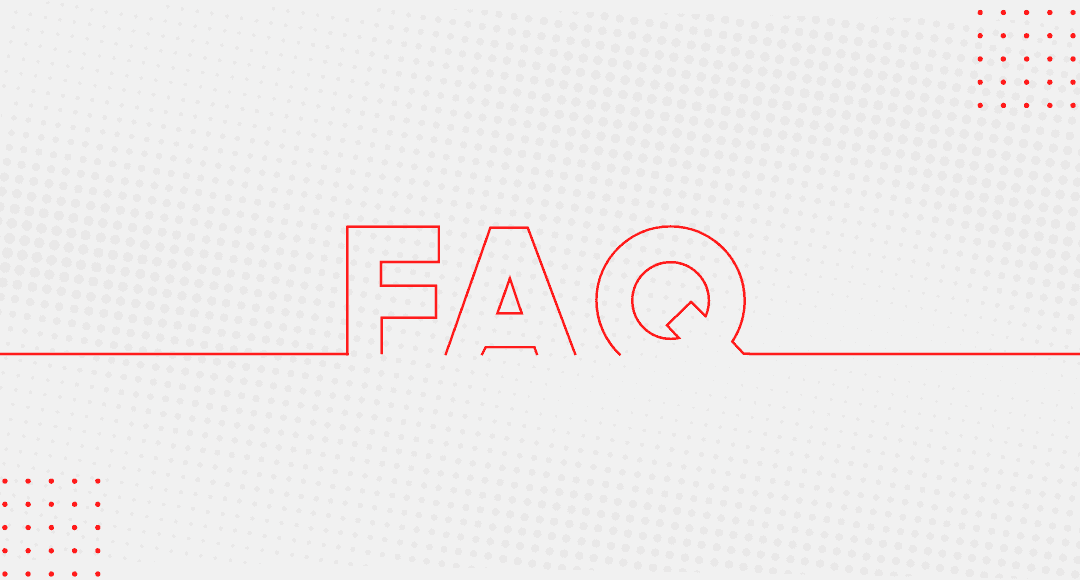
1. What are the risks of using broad role-based access control for EHR systems?
Role-based access control (RBAC) where users are assigned standard profiles like “doctor”, “nurse”, or “admin” is a common but flawed approach for securing sensitive patient health records. Some key risks include:
- Excessive permissions: Broad roles fail to account for specific needs and contexts, granting unnecessary access. For example, an oncologist may get full access to all patient records instead of just cancer patients.
- Policy gaps: Standard roles cannot address all the nuances and shifts in responsibilities across large healthcare organizations. This results in compliance gaps.
- Insider threats: With wide access, bad actors are harder to identify. Disgruntled employees can misuse permissions granted for their generic role.
- No customization: RBAC lacks personalization to tailor access at department or individual levels based on responsibilities.
- Administrative overload: Managing static roles with constantly changing access needs creates compliance reporting and auditing overhead.
In summary, the fundamental limitations of RBAC make it an outdated access control approach for modern healthcare institutions dealing with vast amounts of sensitive patient data.
2. How can AI help create smarter access control policies for EHR systems?
AI and advanced analytics can create personalized, contextual access policies that automatically adapt to changes. Some techniques include:
- Access pattern analysis: AI models identify unique patterns in user data access to define finer-grained persona instead of broad roles. For instance, this can restrict oncologists to only relevant cancer patient records.
- Behavior anomaly detection: Continuously monitor access logs to detect unusual activity based on past behavioral baselines. This flags insider risks early.
- Usage context detection: Analyze workflows, care settings, user department, and other variables to modularize access privileges based on situational needs.
- Predictive intelligence: Just-in-time recommendations to grant, revoke or elevate access based on predicted user responsibilities. This addresses changing access needs.
- Automated provisioning: Use the AI-driven insights to automatically configure tailored data access permissions to each user.
In summary, advanced analytics transforms access governance into a dynamic, personalized privilege model rather than a static permissions structure. This significantly improves security and compliance.
3. What are some key regulations around patient consent management in healthcare?
Some major regulations governing patient consent include:
- HIPAA: Requires healthcare entities to obtain patient consent for disclosing protected health information (PHI) for uses beyond standard treatment, payment and operations. HIPAA consent is complex with elements like expiration, right to revoke etc.
- Common Rule: Mandates consent documentation procedures for participation in clinical research studies and trials. Consent must list risks, benefits, procedures, and more.
- GINA: Prohibits collection of genetic information without explicit consent. This includes family history, genetic tests, services received etc.
- State laws: Individual state regulations add additional consent requirements such as special procedures for HIV status, mental health, substance abuse etc.
Staying compliant across this patchwork of regulations is challenging. Automating consent flows can help significantly.
4. What techniques can AI use to digitize and optimize consent workflows?
AI can drive several aspects of automated consent workflows:
- Natural language processing (NLP): Analyze unstructured text in scanned legacy consent documents or free text entries in forms to extract key attributes and clauses. This structures consent data.
- Computer vision (CV): Intelligently parse consent documents and driver license/ID cards to auto-fill digital forms. This removes manual effort.
- Rules engines: Dynamically adapt forms based on patient answers and care context using preset conditional logic, reducing irrelevant questions.
- Predictive analytics: Forecast consent renewal timelines and prompt providers to send renewal reminders as expiration nears. This prevents lapses.
- Blockchain: Immutably log consent activity on distributed ledger for easy end-to-end audit trails across systems.
- Multilingual NLP: Translate forms and analyze non-English free text consent entries to remove language barriers.
AI delivers significant efficiency, accuracy and compliance gains throughout the consent management lifecycle.
5. How can intelligent chatbots improve patient experience with consent workflows?
Chatbots can enhance patient experience by:
- Providing an intuitive, conversational interface to complete consent procedures instead of combing through complex documents.
- Dynamically answering common consent-related questions or concerns using NLP without human escalation.
- Simplifying legal/medical jargon into easily understandable language based on the patient’s background.
- Proactively initiating renewals or amendments by engaging patients through their preferred communication channels.
- Offering multi-language support to serve diverse populations.
- Analyzing sentiment of patient conversations to identify areas of friction in existing consent flows.
Overall, chatbots create positive, personalized consent interactions at scale across patient demographics to improve comprehension and compliance.
6. What techniques can improve comprehension of consent forms for patients?
Some best practices to enhance consent form comprehension include:
- Simplifying language using familiar terms, avoiding complex grammar, and readability optimization. AI writing assistants can help with this.
- Structured formats like bulleted lists, graphics, and visual callouts for key sections rather than dense blocks of text.
- Testing forms with sample users and incorporating feedback into the design in an iterative manner.
- Segmenting consent documents into discrete sections for each care activity rather than a single comprehensive form.
- Translating forms into diverse languages based on geographic patient demographics. AI powered multi-lingual NLP can enable this at scale.
- Providing online education materials such as videos and FAQs to reinforce understanding of consent procedures.
- Analyzing consent form responses to identify problem areas of low comprehension so they can be reworked. AI analytics dashboards can provide such insights.
The goal should be simplifying consents into an engaging, educational experience for patients instead of a legal necessity. AI and UX best practices can enable this transformation.
7. How can healthcare organizations measure and report on consent management metrics?
Healthcare organizations can measure consent management performance by tracking metrics like:
- Consent completion rate: Percentage of patients completing consent forms out of total admissions or visits. Higher is better.
- Consent approval lag: Average time between initiation and finalization of consents. Lower is better.
- Consent renewal rate: Percentage of expiring consents that are renewed on time. Higher is better.
- Consent form abandonment: Percentage of patients starting but failing to complete consent forms. Lower is better.
- Consent velocity: Average number of consents finished per day/week. Higher indicates greater efficiency.
- Consent form comprehension: Patient scores on quizzes or surveys measuring understanding of consent terms. Higher is better.
AI analytics dashboards can automatically generate insights into these metrics by digesting consent data across fragmented systems. This enables data-driven consent workflow optimization.
8. What are some leading technologies and vendors for patient consent management?
Some leading consent management platforms include:
- Careology: End-to-end enterprise SaaS solution for consent workflows and analytics. Integrates with EHRs.
- n-of-one: Specializes in supporting personalized and translational medicine research consents.
- eConsent: Digital consent tool focusing on clinical trials and life sciences. Supports eSignatures.
- DocuSign: Popular SaaS platform provides eSignature workflows across industries including healthcare consents.
- intelliCorpus: NLP platform analyzes unstructured consent documents to extract key elements and clauses.
Healthcare CIOs have a growing array of AI-based consent management options to evaluate based on their budget, use cases and technology stack.
9. What are some key principles for a patient-centric approach to access control and consent workflows?
Some guiding principles for patient-centric data governance include:
- Transparency: Clearly communicate how health data is accessed and used to patients in simple terms.
- Control: Provide easy mechanisms for consent approvals, amendments, revocation, and renewals.
- Security: Minimize data exposure using contextual access controls instead of broad access.
- Compliance: Continuously monitor and optimize consent and access workflows to adhere to regulations.
- Experience: Leverage AI, mobility, multi-lingual capabilities to simplify engagement for diverse patients.
- Outcomes: Link consents and data usage to personalized care recommendations that improves patient satisfaction.
- Culture: Instill values of empathy, education and empowerment rather than risk-aversion in data governance processes.
A patient-centric philosophy needs to permeate access control and consent mechanisms rather than just treating them as obligatory checkboxes.
10. How can healthcare providers get started with transforming access and consent processes using AI?
Some tips to get started:
- Conduct assessment of current access policies and consent workflows to identify pain points and bottlenecks. Engage both patients and providers.
- Start with a limited pilot of new AI-based capabilities for a small group to demonstrate benefits. For example, digitizing consent forms for a specific department.
- Evaluate various technology solutions but focus on capabilities that will drive measurable improvements in patient experience or provider productivity.
- Phase the rollout to continuously gather feedback from users on what’s working and what’s not to guide iterative enhancements.
- Develop metrics framework aligned to objectives like reduced consent abandonment rates or faster access request approvals. But focus on a few vital metrics rather than an exhaustive list.
- Foster cultural shift through training and education on why new patient-centric processes are beneficial even if there is initial lack of comfort with change.
With the right strategic roadmap centered around patient needs, AI can transform data access and consent experiences to be seamless as well as secure.
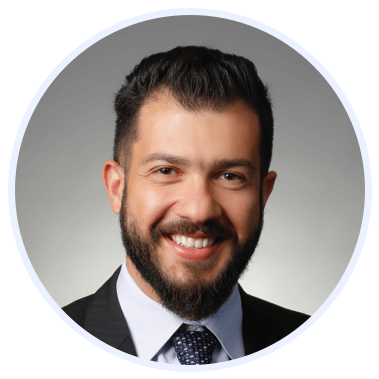
Rasheed Rabata
Is a solution and ROI-driven CTO, consultant, and system integrator with experience in deploying data integrations, Data Hubs, Master Data Management, Data Quality, and Data Warehousing solutions. He has a passion for solving complex data problems. His career experience showcases his drive to deliver software and timely solutions for business needs.