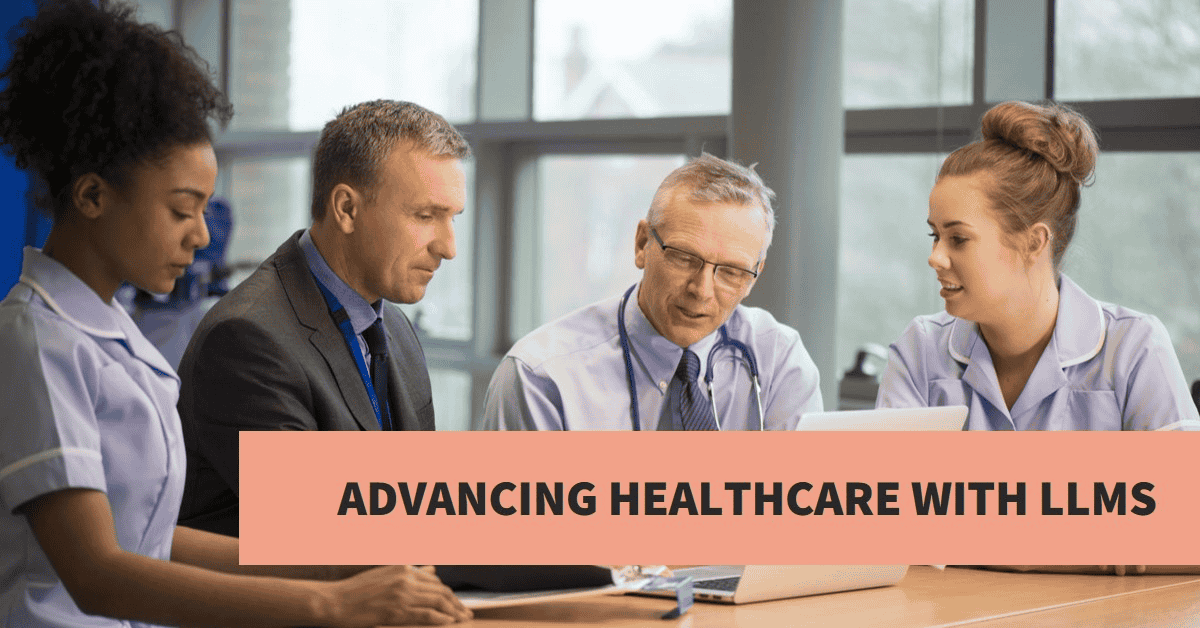
As the healthcare industry continues to evolve, the integration of advanced technologies like Large Language Models (LLMs) is becoming increasingly crucial. LLMs, a subset of artificial intelligence (AI), have the potential to revolutionize medical diagnosis and treatment by tapping into vast amounts of data and natural language processing capabilities. In this blog post, we will explore the opportunities and challenges associated with the application of LLMs in healthcare, drawing from my experience as a former CTO and technology leader.

Understanding LLMs and Their Potential in Healthcare

LLMs are AI models trained on massive datasets of text, enabling them to understand, generate, and manipulate human language with remarkable accuracy. In the context of healthcare, LLMs can be applied to various tasks, such as:
- Analyzing patient symptoms and medical history
- Predicting disease onset and progression
- Recommending personalized treatment plans
- Enhancing patient-provider communication
By processing and interpreting vast amounts of medical literature, clinical notes, and patient data, LLMs can assist healthcare professionals in making more informed decisions and improving patient outcomes.

Real-World Applications and Case Studies

Several healthcare organizations and technology companies have already begun exploring the potential of LLMs in medical diagnosis and treatment. For example:
Symptom Analysis: A leading healthcare provider partnered with an AI company to develop an LLM-powered chatbot that helps patients describe their symptoms and provides initial triage recommendations. The chatbot has shown promising results in reducing unnecessary emergency room visits and directing patients to appropriate care.
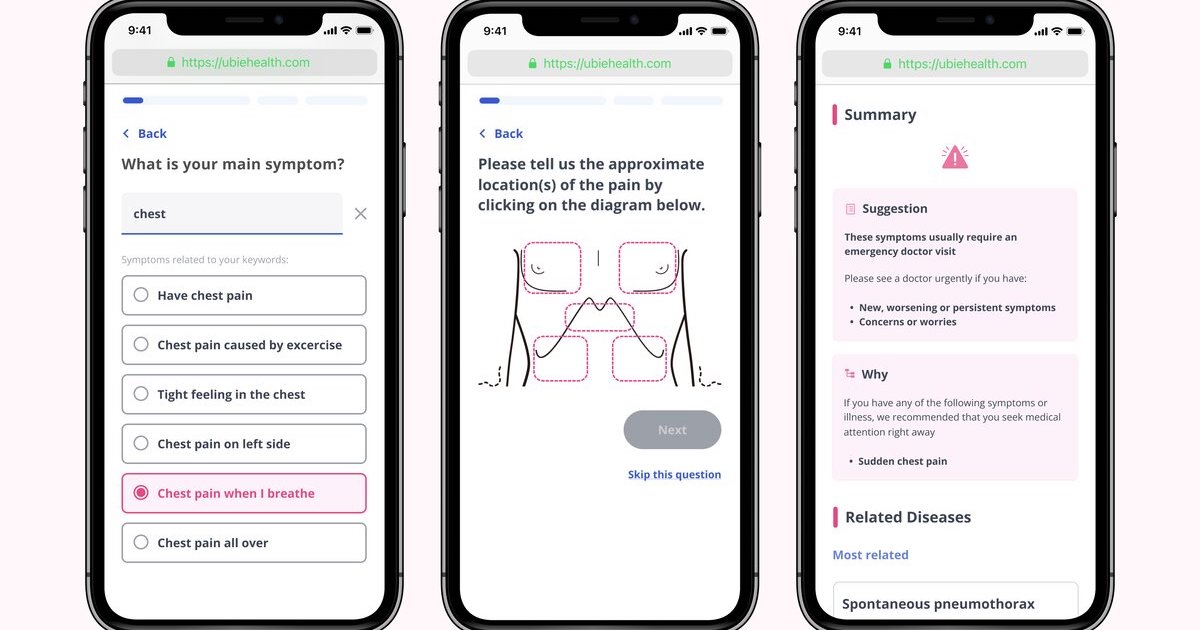
Disease Prediction: Researchers at a renowned medical university developed an LLM that predicts the likelihood of a patient developing certain chronic diseases based on their electronic health records (EHRs). The model has demonstrated high accuracy in identifying patients at risk of diabetes, heart disease, and other conditions, enabling early intervention and preventive care.
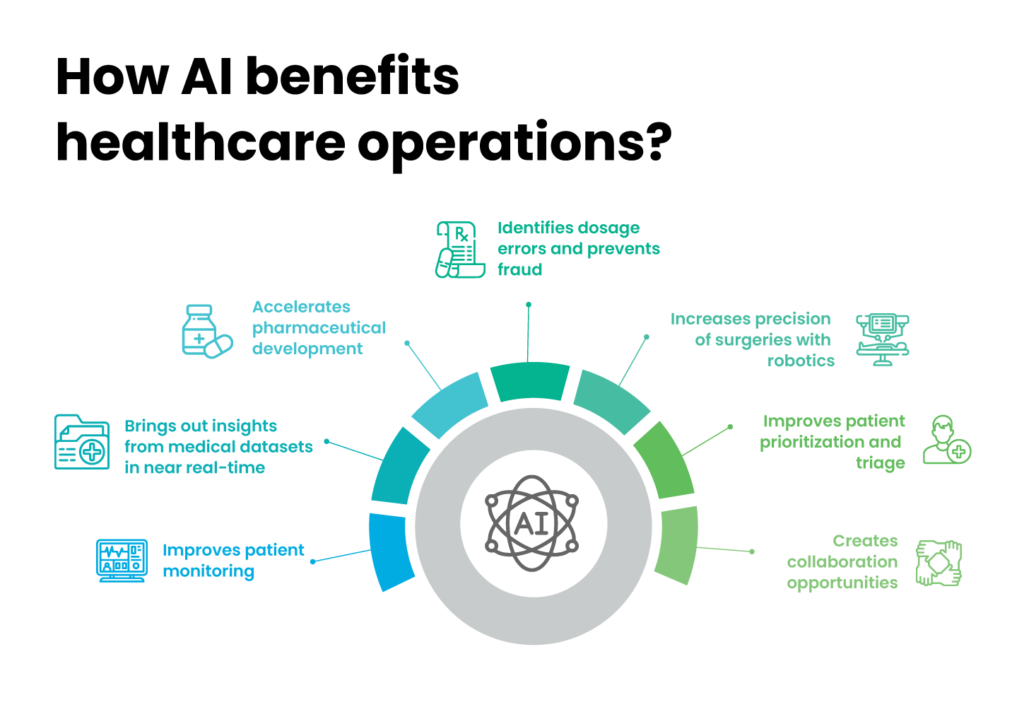
Benefits and Challenges
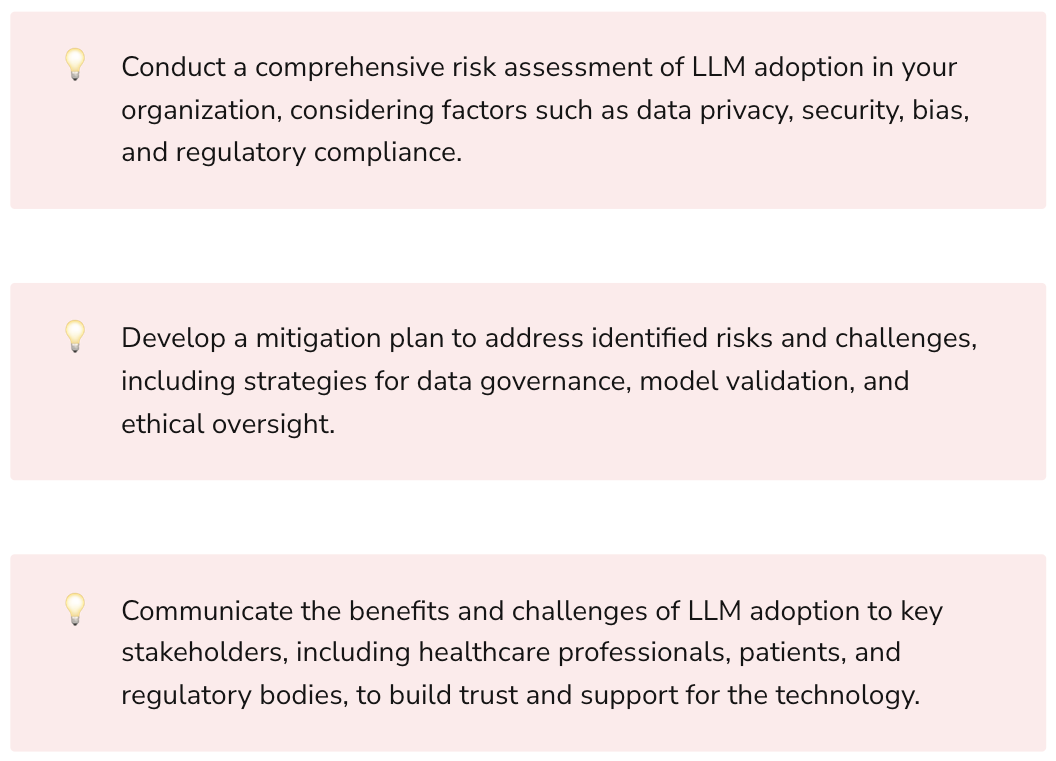
The integration of LLMs in healthcare offers several potential benefits, including:
- Improved diagnostic accuracy and efficiency
- Personalized treatment recommendations
- Enhanced patient engagement and communication
- Increased accessibility of medical expertise in underserved areas
However, the adoption of LLMs in healthcare also faces significant challenges, such as:
- Ensuring data privacy and security compliance (e.g., HIPAA, GDPR)
- Addressing potential biases and disparities in training data
- Validating model performance and clinical efficacy
- Integrating LLMs into existing healthcare workflows and systems

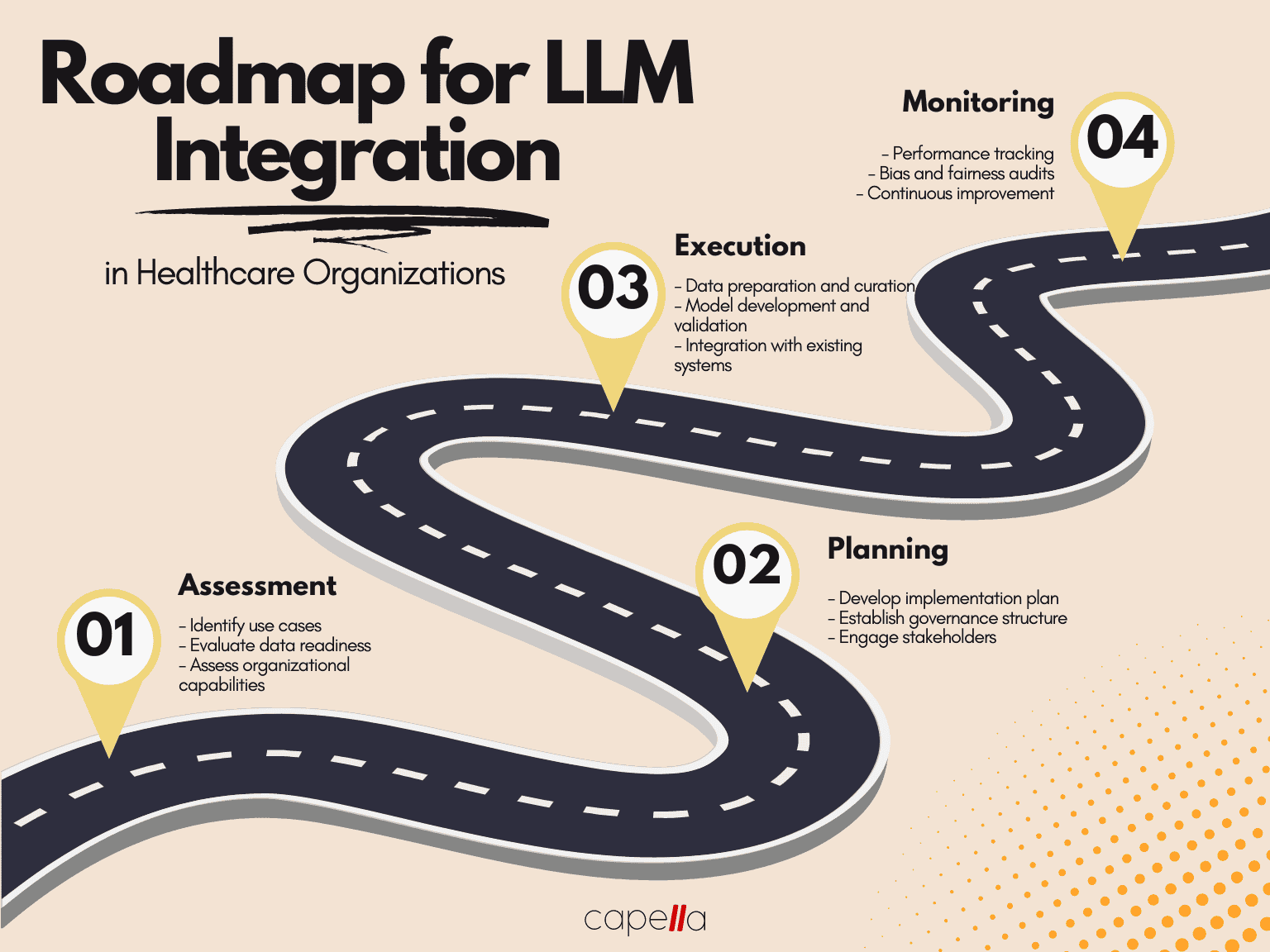
Strategies for Effective Integration
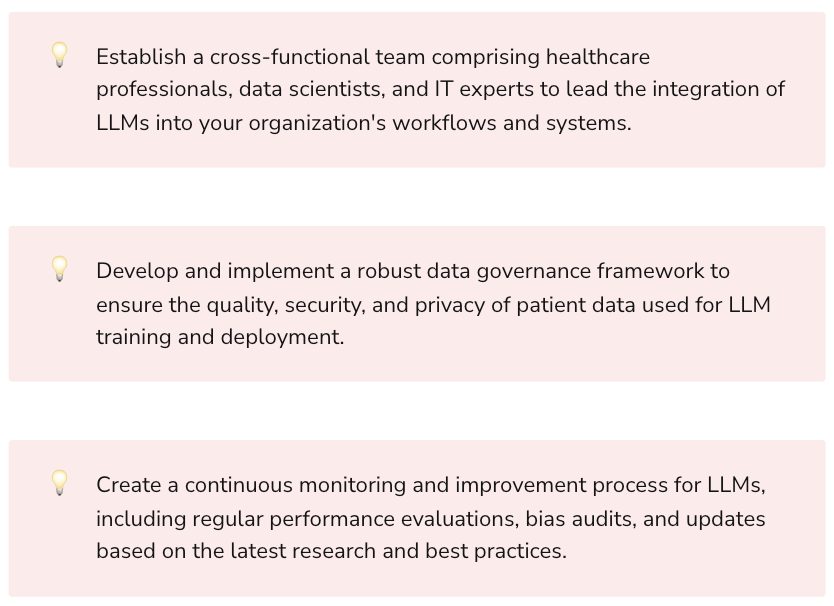
To effectively integrate LLMs into healthcare systems and workflows, organizations should consider the following strategies:
Data Preparation: Ensure that the training data for LLMs is high-quality, diverse, and representative of the target patient population. Implement robust data governance and privacy protection measures.
Model Validation: Conduct rigorous testing and validation of LLMs to assess their performance, fairness, and clinical efficacy. Engage domain experts and healthcare professionals in the validation process.
Human-AI Collaboration: Foster collaboration between LLMs and human healthcare professionals, using the strengths of both. LLMs can provide data-driven insights and recommendations, while human experts can provide contextual understanding and final decision-making.
Ethical Considerations: Develop and adhere to ethical guidelines for the use of LLMs in healthcare, ensuring transparency, accountability, and patient consent. Regularly audit and monitor LLMs for potential biases and unintended consequences.
Regulatory Compliance: Work closely with regulatory bodies to ensure that the deployment of LLMs in healthcare complies with relevant laws, regulations, and industry standards.

The Importance of Domain Expertise and Collaboration
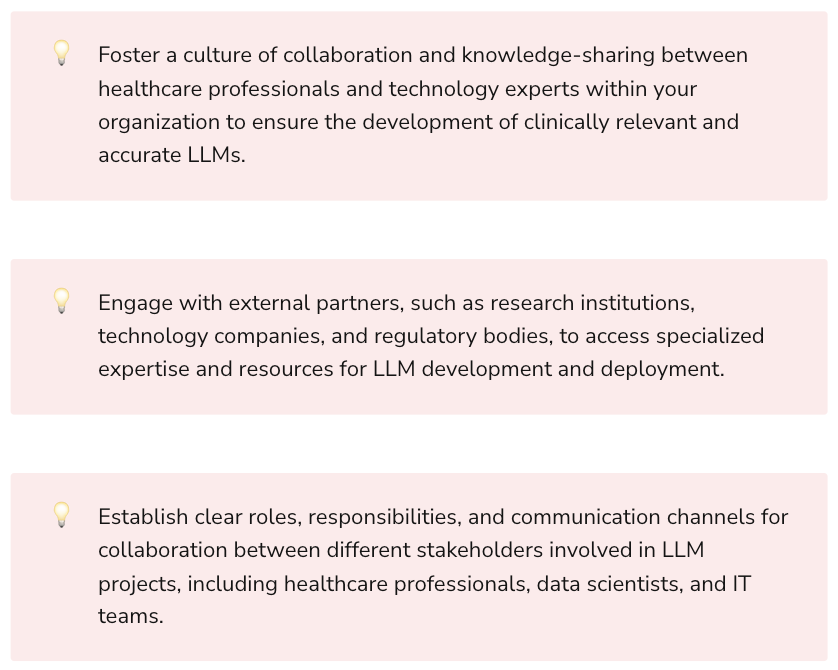
The successful application of LLMs in healthcare requires a collaborative effort between healthcare providers, technology companies, and regulatory bodies. Domain expertise is crucial in developing and deploying LLMs that are clinically relevant, accurate, and safe.

Healthcare professionals, including physicians, nurses, and researchers, should be actively involved in the design, development, and validation of LLMs. Their deep understanding of medical knowledge, clinical workflows, and patient needs can help ensure that LLMs are aligned with real-world healthcare requirements.
Technology companies, on the other hand, bring expertise in AI, data management, and scalable infrastructure. They can provide the technical foundation and innovation necessary to build and deploy robust LLM solutions in healthcare.
Regulatory bodies play a critical role in establishing guidelines, standards, and oversight mechanisms for the use of LLMs in healthcare. They help ensure that patient safety, privacy, and ethical considerations are prioritized throughout the development and deployment process.
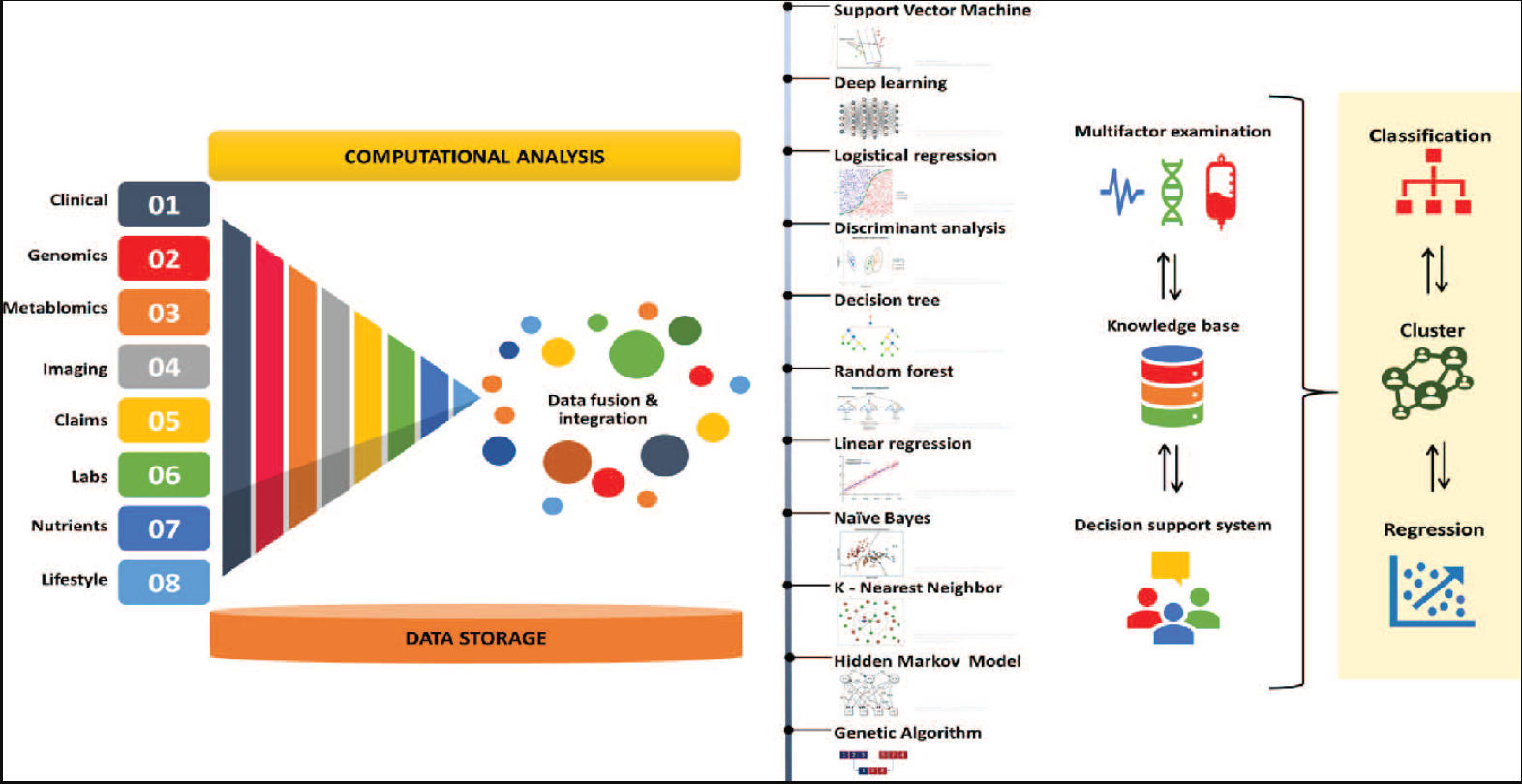
Future Developments and Research Directions

As the field of LLMs in healthcare continues to evolve, several exciting future developments and research directions emerge:
Personalized Medicine: LLMs can be employed to analyze patient-specific data, such as genomic information, lifestyle factors, and medical history, to develop highly personalized treatment plans and interventions.
Drug Discovery: LLMs can accelerate the drug discovery process by analyzing vast amounts of biomedical literature, identifying potential drug targets, and predicting drug-drug interactions.

Clinical Decision Support: Integration of LLMs into clinical decision support systems can provide healthcare professionals with real-time, evidence-based recommendations, improving the quality and consistency of care.
Mental Health Support: LLMs can be used to develop conversational agents that provide mental health support, such as cognitive-behavioral therapy, and help individuals manage stress, anxiety, and depression.
Conclusion
The application of LLMs in healthcare presents a transformative opportunity to improve medical diagnosis, treatment, and patient outcomes. LLMs can help healthcare professionals improve the quality and accessibility of care by using natural language processing and vast medical datasets.
However, the adoption of LLMs in healthcare also comes with significant challenges, including data privacy, bias, regulatory compliance, and integration with existing systems. Overcoming these challenges requires a collaborative effort between healthcare providers, technology companies, and regulatory bodies.
As we move forward, it is essential to prioritize ethical considerations, clinical validation, and human-AI collaboration in the development and deployment of LLMs in healthcare. By doing so, we can harness the full potential of this transformative technology while ensuring patient safety and well-being.
The future of healthcare is exciting, and LLMs will undoubtedly play a significant role in shaping it. As leaders in the healthcare and technology industries, it is our responsibility to navigate this landscape responsibly and collaboratively, always keeping the best interests of patients at the forefront.
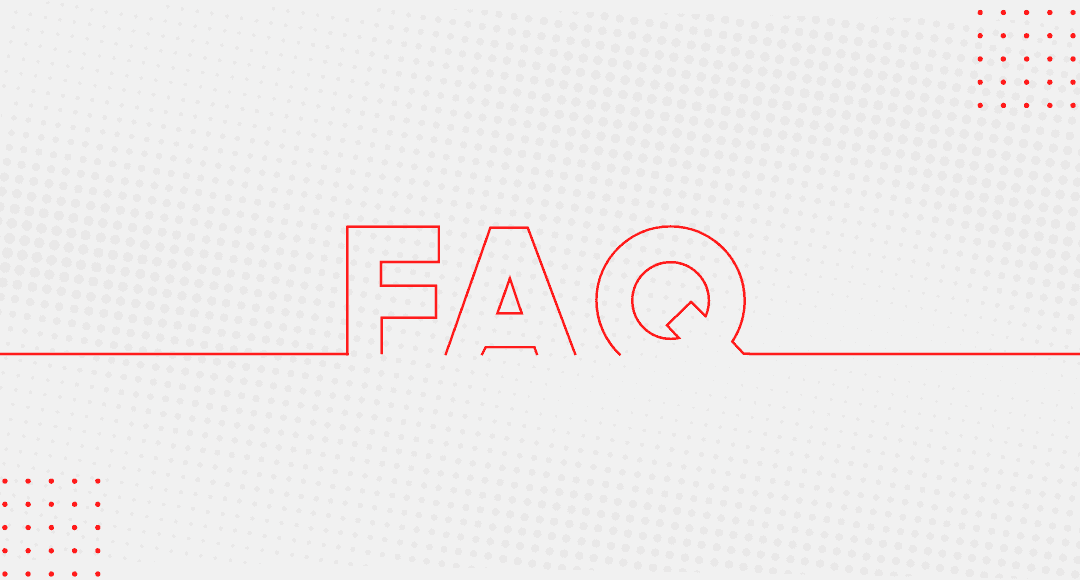
1. What are Large Language Models (LLMs) and how do they differ from other AI models?
Large Language Models (LLMs) are a type of AI model that is trained on vast amounts of text data to understand, generate, and manipulate human language. Unlike other AI models that focus on specific tasks or domains, LLMs can be applied to a wide range of language-related tasks and can generate human-like responses to prompts.
2. How can LLMs be used in healthcare, specifically for medical diagnosis and treatment?
LLMs can be used in healthcare for various applications, such as analyzing patient symptoms, predicting disease progression, recommending personalized treatment plans, and enhancing patient-provider communication. By processing and interpreting large amounts of medical data, LLMs can assist healthcare professionals in making more accurate and efficient diagnoses and treatment decisions.
3. Are there any real-world examples of LLMs being used in healthcare?
Yes, several healthcare organizations and technology companies have already begun exploring the use of LLMs in medical diagnosis and treatment. For example, some have developed LLM-powered chatbots for symptom analysis and triage, while others have used LLMs to predict the likelihood of patients developing certain chronic diseases based on their electronic health records (EHRs).
4. What are the main benefits of using LLMs in healthcare?
The main benefits of using LLMs in healthcare include improved diagnostic accuracy, increased efficiency, personalized treatment recommendations, enhanced patient engagement and communication, and increased accessibility of medical expertise in underserved areas.
5. What are the challenges and limitations of using LLMs in healthcare?
Some of the key challenges and limitations of using LLMs in healthcare include ensuring data privacy and security compliance, addressing potential biases and disparities in training data, validating model performance and clinical efficacy, and integrating LLMs into existing healthcare workflows and systems.
6. How can healthcare organizations effectively integrate LLMs into their existing systems and workflows?
To effectively integrate LLMs into their existing systems and workflows, healthcare organizations should focus on data preparation, model validation, human-AI collaboration, ethical considerations, and regulatory compliance. This involves ensuring high-quality and diverse training data, conducting rigorous testing and validation, fostering collaboration between LLMs and human experts, adhering to ethical guidelines, and working closely with regulatory bodies.
7. Why is domain expertise important in developing and deploying LLMs in healthcare?
Domain expertise is crucial in developing and deploying LLMs in healthcare because it ensures that the models are clinically relevant, accurate, and safe. Healthcare professionals, such as physicians and nurses, can provide valuable insights into medical knowledge, clinical workflows, and patient needs, which can help align LLMs with real-world healthcare requirements.
8. What role do technology companies and regulatory bodies play in the adoption of LLMs in healthcare?
Technology companies provide the technical foundation and innovation necessary to build and deploy robust LLM solutions in healthcare, while regulatory bodies establish guidelines, standards, and oversight mechanisms to ensure patient safety, privacy, and ethical considerations are prioritized throughout the development and deployment process.
9. What are some potential future developments and research directions for LLMs in healthcare?
Some potential future developments and research directions for LLMs in healthcare include personalized medicine, drug discovery, clinical decision support, and mental health support. LLMs can be utilized to analyze patient-specific data, accelerate drug discovery, provide evidence-based recommendations to healthcare professionals, and develop conversational agents for mental health support.
10. How can healthcare organizations and professionals prepare for the increasing adoption of LLMs in the industry?
Healthcare organizations and professionals can prepare for the increasing adoption of LLMs by staying informed about the latest developments and best practices, investing in building in-house expertise and capabilities, collaborating with technology partners and research institutions, and actively participating in shaping the ethical and regulatory frameworks governing the use of LLMs in healthcare.
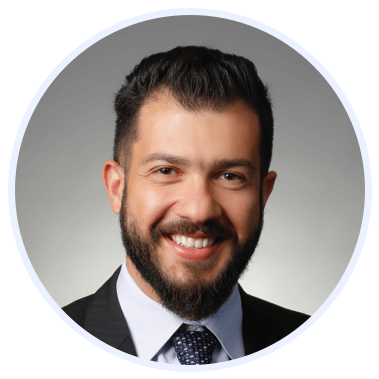
Rasheed Rabata
Is a solution and ROI-driven CTO, consultant, and system integrator with experience in deploying data integrations, Data Hubs, Master Data Management, Data Quality, and Data Warehousing solutions. He has a passion for solving complex data problems. His career experience showcases his drive to deliver software and timely solutions for business needs.