
Effective inventory management is crucial for companies striving to stay competitive. Executives and decision-makers in large enterprises are constantly seeking innovative solutions to optimize their inventory levels, reduce costs, and improve customer satisfaction. One powerful tool that has emerged in recent years is data analytics. By harnessing the power of data, companies can gain valuable insights into their inventory management processes and make data-driven decisions to enhance efficiency and profitability.

In this blog, we will explore a real-life example of a company that successfully implemented data analytics to transform its inventory management practices. By examining their journey, we will uncover the key benefits, challenges, and best practices associated with using data analytics for effective inventory management.

Case Study: Aliaxis Corporation
To illustrate the impact of data analytics on inventory management, let's delve into the case of Aliaxis Corporation, a global leader in manufacturing and distribution. Aliaxis had been facing significant challenges in managing their vast inventory, resulting in excessive carrying costs, stockouts, and missed sales opportunities. Seeking a solution, Aliaxis embarked on a data analytics initiative to gain deeper insights into their inventory operations.

The Data Analytics Solution
Aliaxis began by consolidating and integrating their data from various sources, including sales records, supplier information, production schedules, and customer feedback. This data formed the foundation for their inventory analytics platform. Leveraging advanced analytics techniques, Aliaxis implemented a series of algorithms and models to analyze their inventory data and generate actionable insights.

Key Benefits of Data Analytics for Aliaxis
The implementation of data analytics brought about a multitude of benefits for Aliaxis Corporation. Let's explore some of the key advantages they experienced:
Demand Forecasting: By analyzing historical sales data, market trends, and external factors, Aliaxis could accurately forecast demand for their products. This enabled them to optimize inventory levels and avoid both excess and insufficient stock.
Optimized Reordering: Leveraging data analytics, Aliaxis identified the optimal reorder points and order quantities for each product. This eliminated overstocking and reduced lead times, resulting in significant cost savings.
Supplier Performance Evaluation: Aliaxis used data analytics to evaluate the performance of their suppliers. By monitoring metrics such as delivery times, quality, and pricing, they could make informed decisions about their supplier relationships, ensuring reliability and efficiency in the supply chain.
Stockout Prevention: Through real-time monitoring of inventory levels and demand patterns, Aliaxis successfully mitigated the risk of stockouts. By proactively replenishing stock, they avoided lost sales opportunities and improved customer satisfaction.
Inventory Optimization: Aliaxis leveraged data analytics to identify slow-moving and obsolete inventory. By addressing these issues promptly, they freed up valuable warehouse space and reduced carrying costs.
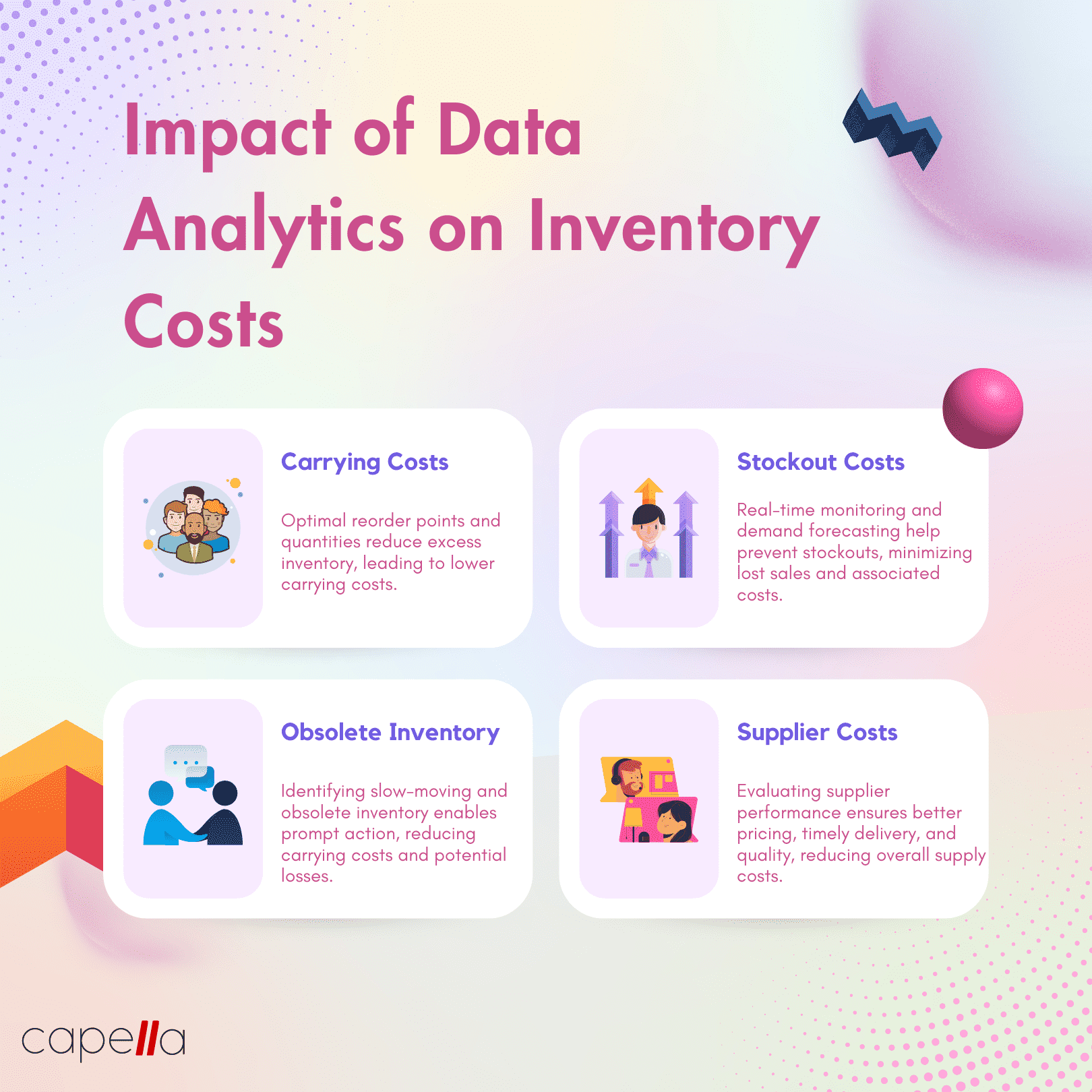
Overcoming Challenges
While the benefits of data analytics in inventory management are substantial, Aliaxis faced several challenges during their implementation. It is important to be aware of these potential obstacles and address them proactively. Some of the challenges encountered by Aliaxis and their corresponding solutions include:
Data Quality: Aliaxis initially faced challenges in ensuring data accuracy and completeness. They addressed this issue by investing in data cleansing and validation processes, as well as establishing data governance protocols.
Integration Complexity: Consolidating data from disparate sources proved to be a complex task for Aliaxis. To overcome this challenge, they employed data integration tools and technologies, ensuring seamless data flow across the organization.
Skill Set Requirements: Aliaxis had to upskill its workforce to utilize data analytics tools and interpret insights effectively. They invested in training programs and hired data analytics experts to bridge the skills gap.
Change Management: Implementing data analytics necessitated a shift in Aliaxis's organizational culture. They focused on change management strategies, fostering a data-driven mindset among employees and ensuring buy-in from all stakeholders.

Best Practices for Effective Data Analytics in Inventory Management
Based on Aliaxis's experience and industry best practices, here are some key recommendations for effectively using data analytics in inventory management:
Define Clear Objectives: Clearly define the goals and objectives of your data analytics initiative. Identify the key performance indicators (KPIs) you aim to improve and align your efforts accordingly.
Leverage Advanced Analytics Techniques: Utilize advanced analytics techniques such as forecasting models, optimization algorithms, and machine learning algorithms to extract meaningful insights from your inventory data.
Invest in Data Quality: Ensure the accuracy, completeness, and reliability of your data. Implement data cleansing and validation processes, and establish data governance protocols to maintain data integrity.
Integrate Data Sources: Consolidate data from various sources, including sales records, supplier information, and customer feedback. Invest in data integration tools and technologies to enable seamless data flow and comprehensive analysis.
Build a Skilled Team: Invest in training programs and recruit data analytics experts to build a team capable of effectively leveraging data analytics tools and interpreting insights.
Foster a Data-Driven Culture: Promote a data-driven mindset across the organization. Encourage employees to embrace data-driven decision-making and provide them with the necessary tools and resources to do so.
Continuously Monitor and Improve: Establish a feedback loop and continuously monitor the performance of your data analytics initiatives. Regularly evaluate the outcomes and refine your strategies to drive continuous improvement.
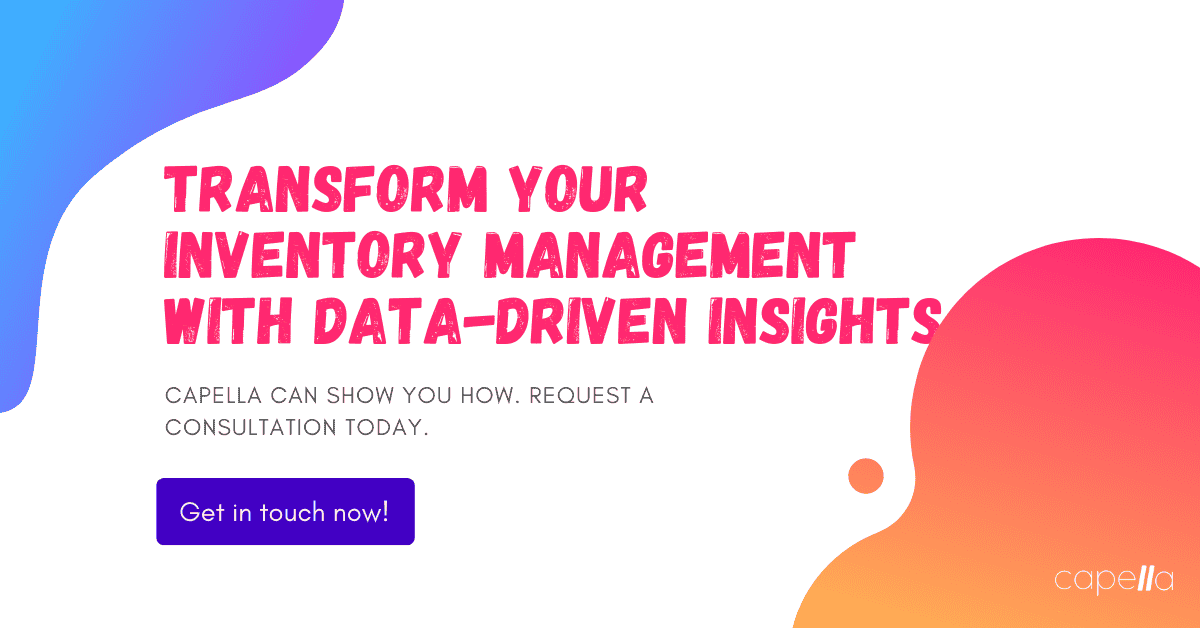
Closing Thoughts
Data analytics has emerged as a game-changer in inventory management, enabling companies to optimize their inventory levels, reduce costs, and improve customer satisfaction. Aliaxis Corporation's successful implementation of data analytics serves as a real-life example of the transformative impact this technology can have. By leveraging data analytics techniques, companies can gain actionable insights, enhance decision-making, and drive operational excellence in their inventory management practices. To stay ahead in today's competitive landscape, executives and decision-makers must embrace data analytics and leverage it to unlock the full potential of their inventory management processes.
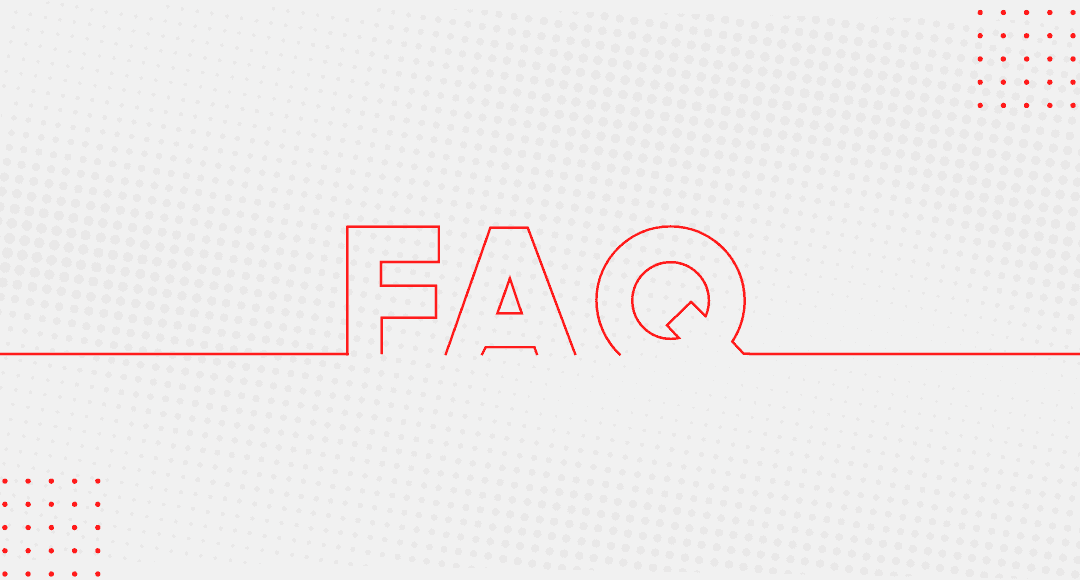
Q1: How can data analytics improve demand forecasting for inventory management?
A1: Data analytics improves demand forecasting by analyzing historical sales data, market trends, and external factors. Advanced techniques such as time series analysis and predictive modeling are applied to identify patterns, seasonality, and demand drivers. By leveraging these insights, companies can accurately forecast future demand, optimize inventory levels, and avoid both excess stock and stockouts.
Q2: What is the impact of data analytics on inventory carrying costs?
A2: Data analytics has a significant impact on inventory carrying costs. By optimizing reorder points and quantities, companies can reduce excess inventory, which directly lowers carrying costs. Accurate demand forecasting and real-time monitoring of inventory levels also help prevent stockouts, minimizing lost sales and associated costs. By identifying slow-moving and obsolete inventory, data analytics enables prompt action, freeing up valuable warehouse space and reducing carrying costs further.
Q3: How does data analytics help in supplier performance evaluation?
A3: Data analytics plays a vital role in evaluating supplier performance. By monitoring supplier metrics such as delivery times, quality, and pricing, companies can make informed decisions about supplier relationships. Advanced analytics techniques enable the analysis of supplier data, identifying areas of improvement and ensuring reliability and efficiency in the supply chain. By leveraging data-driven insights, companies can strengthen their supplier partnerships, negotiate better terms, and drive cost savings.
Q4: Can data analytics prevent stockouts and improve customer satisfaction?
A4: Yes, data analytics can prevent stockouts and improve customer satisfaction. Real-time monitoring of inventory levels combined with accurate demand forecasting helps companies proactively replenish stock, reducing the risk of stockouts. By ensuring products are available when customers need them, companies can meet customer expectations, increase fill rates, and enhance customer satisfaction. Timely delivery of orders and improved order accuracy, driven by data analytics insights, also contribute to customer satisfaction.
Q5: How can data analytics optimize inventory levels?
A5: Data analytics optimizes inventory levels by providing insights into demand patterns, seasonality, and customer behavior. By leveraging advanced analytics techniques, such as optimization algorithms, companies can determine optimal reorder points and order quantities for each product. This helps minimize lead times, eliminate overstocking, and achieve significant cost savings. By analyzing historical sales data and market trends, data analytics identifies opportunities to right-size inventory, improving operational efficiency and profitability.
Q6: What are the key challenges in implementing data analytics for inventory management?
A6: Implementing data analytics for inventory management can present challenges. Some key challenges include ensuring data quality and accuracy, integrating data from various sources, addressing skill set requirements, and managing change within the organization. Data cleansing and validation processes, data governance protocols, and investment in data integration tools can help overcome these challenges. Additionally, upskilling the workforce through training programs and fostering a data-driven culture are crucial to successful implementation.
Q7: How can companies overcome data quality challenges in data analytics implementation?
A7: Overcoming data quality challenges requires a multi-faceted approach. Companies can invest in data cleansing and validation processes to ensure data accuracy and completeness. Data governance protocols help maintain data integrity and consistency across systems. Regular data source audits and validation procedures can minimize errors. Collaborating with IT teams and implementing robust data quality management practices will ensure high-quality data for accurate analytics and reliable insights.
Q8: What are the best practices for effective data analytics in inventory management?
A8: Effective data analytics in inventory management requires following best practices. These include defining clear objectives for the data analytics initiative, leveraging advanced analytics techniques for accurate insights, investing in data quality through cleansing and validation processes, integrating data sources for comprehensive analysis, building a skilled team with data analytics expertise, fostering a data-driven culture, and continuously monitoring and improving based on data-driven insights. By adopting these best practices, companies can optimize inventory management and achieve better business outcomes.
Q9: How can companies foster a data-driven culture in inventory management?
A9: Fostering a data-driven culture in inventory management involves several key steps. It starts with executive support and commitment to data-driven decision-making. Companies should provide employees with access to data analytics tools and resources and offer training programs to enhance analytical skills. Creating cross-functional teams and encouraging collaboration between departments helps foster a culture of data-driven decision-making. Regular communication of successes achieved through data-driven approaches reinforces the value and benefits of using data analytics in inventory management.
Q10: How should companies monitor and evaluate the performance of their data analytics initiatives?
A10: Companies should establish a feedback loop to monitor and evaluate the performance of their data analytics initiatives. This involves defining key performance indicators (KPIs) aligned with the objectives of the initiative, such as inventory turnover, fill rates, and cost savings. Regularly analyze and measure the outcomes against these KPIs, leveraging data analytics tools and techniques. Conduct periodic reviews and refine strategies based on insights gained. Continuous monitoring and evaluation enable companies to identify areas for improvement, optimize processes, and drive ongoing enhancements in their inventory management practices.
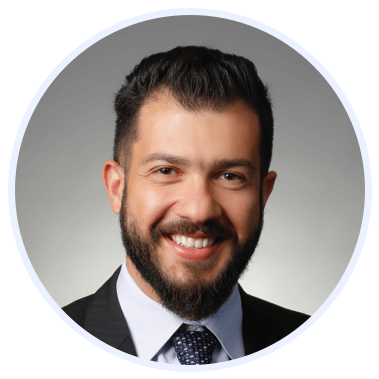
Rasheed Rabata
Is a solution and ROI-driven CTO, consultant, and system integrator with experience in deploying data integrations, Data Hubs, Master Data Management, Data Quality, and Data Warehousing solutions. He has a passion for solving complex data problems. His career experience showcases his drive to deliver software and timely solutions for business needs.